Identifying Top-k Players in Cooperative Games via Shapley Bandits.
LWDA(2021)
摘要
The usefulness of cooperative game theory and key concepts like the Shapley value, which measures the contribution of individual players to the overall performance of a coalition, has been demonstrated in various applications. Due to the computational effort growing exponentially with the number of participants in a game, several methods have been proposed to approximate Shapley values. Yet, in many applications, only the order of players according to their Shapley values is important, or maybe the set of the k best players, but not the values themselves. In this paper, we consider the problem of identifying the k players in a cooperative game with the highest Shapley values and denote it as the Top-k Shapley problem. By viewing the marginal contributions of a player as a random variable, we establish a connection between cooperative games and multi-armed bandits, which in turn allows us to reduce Top-k Shapley to the multiple arms identification problem. We call the resulting bandits problem Shapley bandits. Besides adopting existing algorithms for multiple arms identifications, we propose the Border Uncertainty Sampling algorithm (BUS) and provide empirical evidence for its superiority over state-of-the-art algorithms.
更多查看译文
关键词
shapley bandits,cooperative games,identifying
AI 理解论文
溯源树
样例
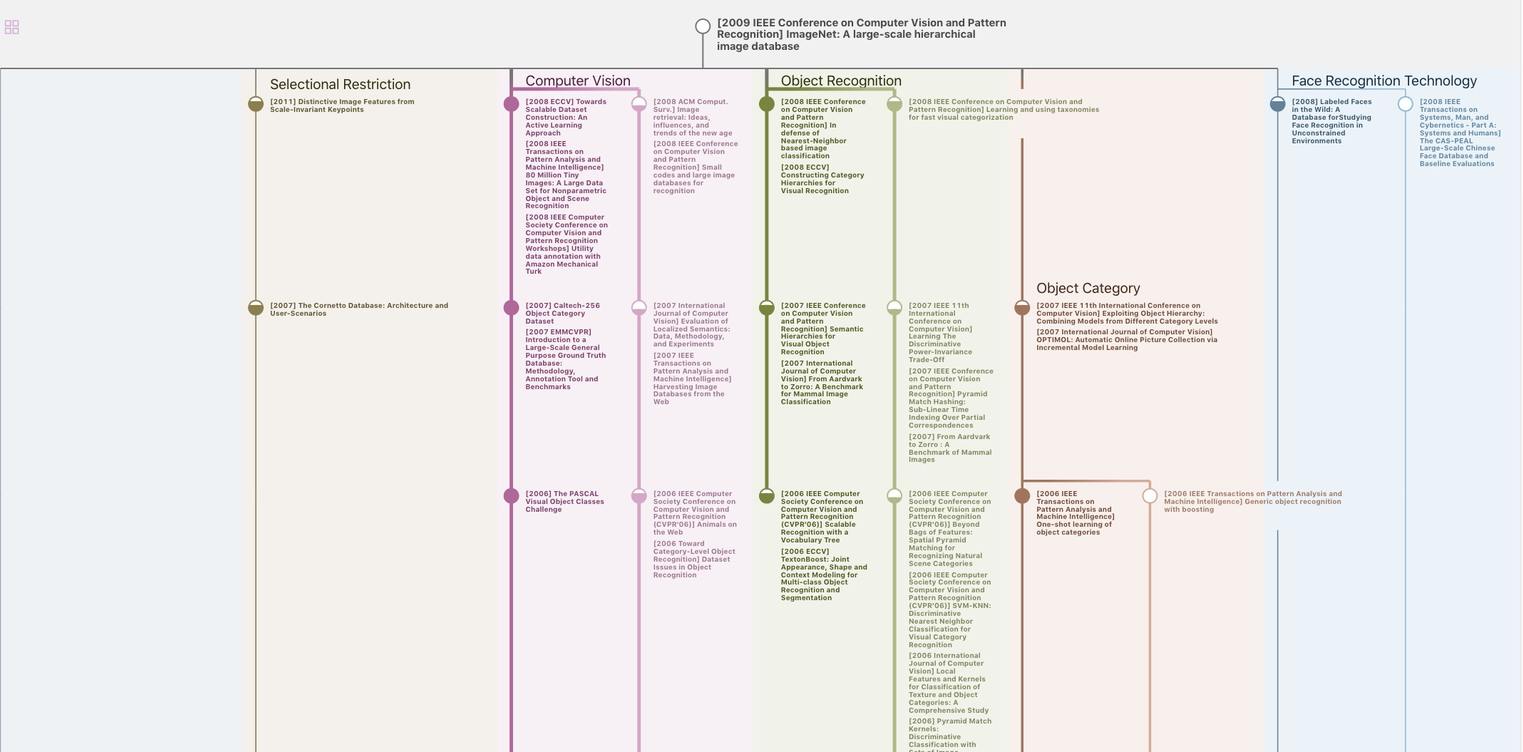
生成溯源树,研究论文发展脉络
Chat Paper
正在生成论文摘要