Learning Coordinated Terrain-Adaptive Locomotion by Imitating a Centroidal Dynamics Planner.
IEEE/RJS International Conference on Intelligent RObots and Systems (IROS)(2022)
摘要
Dynamic quadruped locomotion over challenging terrains with precise foot placements is a hard problem for both optimal control methods and Reinforcement Learning (RL). Non-linear solvers can produce coordinated constraint satisfying motions, but often take too long to converge for online application. RL methods can learn dynamic reactive controllers but require carefully tuned shaping rewards to produce good gaits and can have trouble discovering precise coordinated movements. Imitation learning circumvents this problem and has been used with motion capture data to extract quadruped gaits for flat terrains. However, it would be costly to acquire motion capture data for a very large variety of terrains with height differences. In this work, we combine the advantages of trajectory optimization and learning methods and show that terrain adaptive controllers can be obtained by training policies to imitate trajectories that have been planned over procedural terrains by a non-linear solver. We show that the learned policies transfer to unseen terrains and can be fine-tuned to dynamically traverse challenging terrains that require precise foot placements and are very hard to solve with standard RL.
更多查看译文
关键词
dynamics,learning,terrain-adaptive
AI 理解论文
溯源树
样例
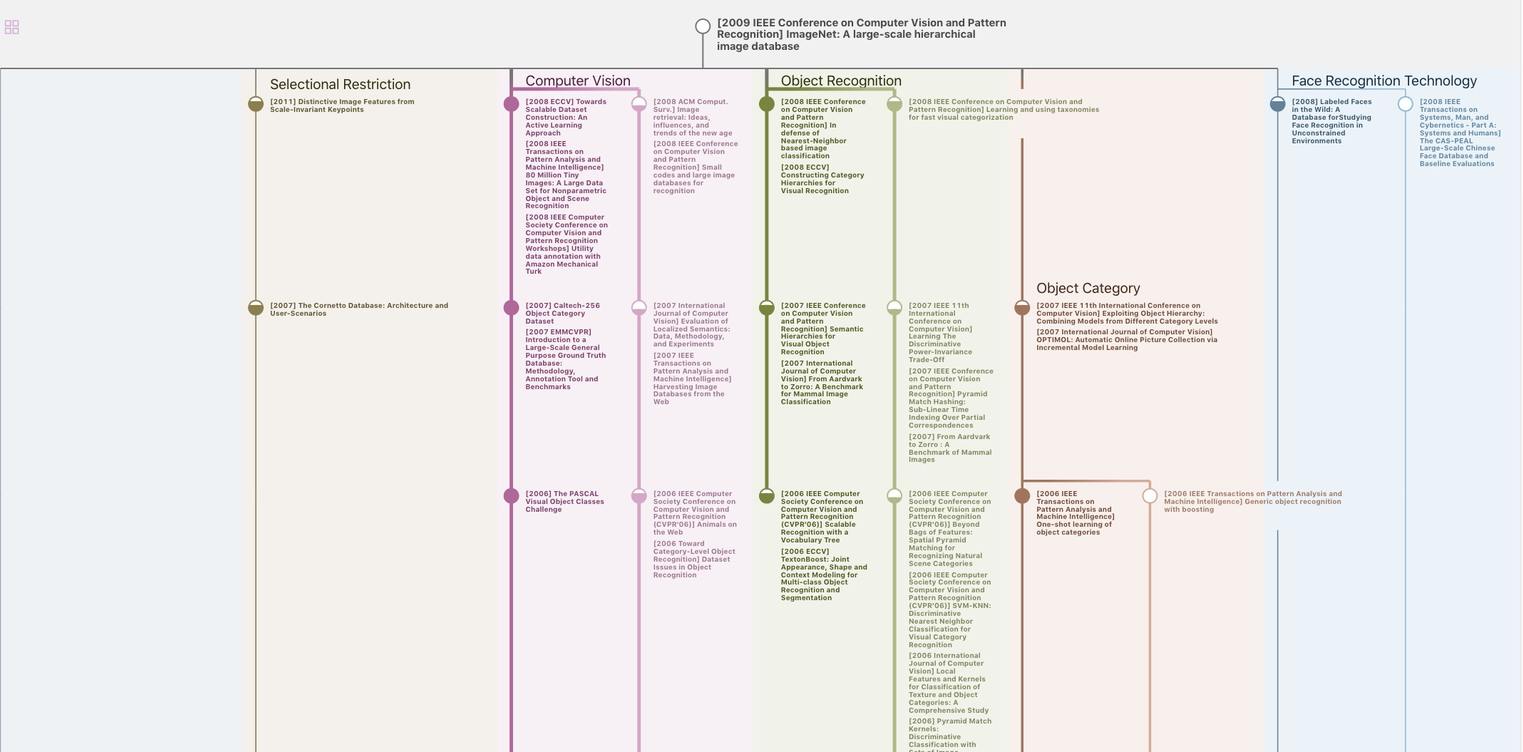
生成溯源树,研究论文发展脉络
Chat Paper
正在生成论文摘要