Context meta-reinforcement learning via neuromodulation
Neural Networks(2022)
摘要
Meta-reinforcement learning (meta-RL) algorithms enable agents to adapt quickly to tasks from few samples in dynamic environments. Such a feat is achieved through dynamic representations in an agent’s policy network (obtained via reasoning about task context, model parameter updates, or both). However, obtaining rich dynamic representations for fast adaptation beyond simple benchmark problems is challenging due to the burden placed on the policy network to accommodate different policies. This paper addresses the challenge by introducing neuromodulation as a modular component to augment a standard policy network that regulates neuronal activities in order to produce efficient dynamic representations for task adaptation. The proposed extension to the policy network is evaluated across multiple discrete and continuous control environments of increasing complexity. To prove the generality and benefits of the extension in meta-RL, the neuromodulated network was applied to two state-of-the-art meta-RL algorithms (CAVIA and PEARL). The result demonstrates that meta-RL augmented with neuromodulation produces significantly better result and richer dynamic representations in comparison to the baselines.
更多查看译文
关键词
Meta-learning,Lifelong-learning,Deep reinforcement learning,Neuromodulation
AI 理解论文
溯源树
样例
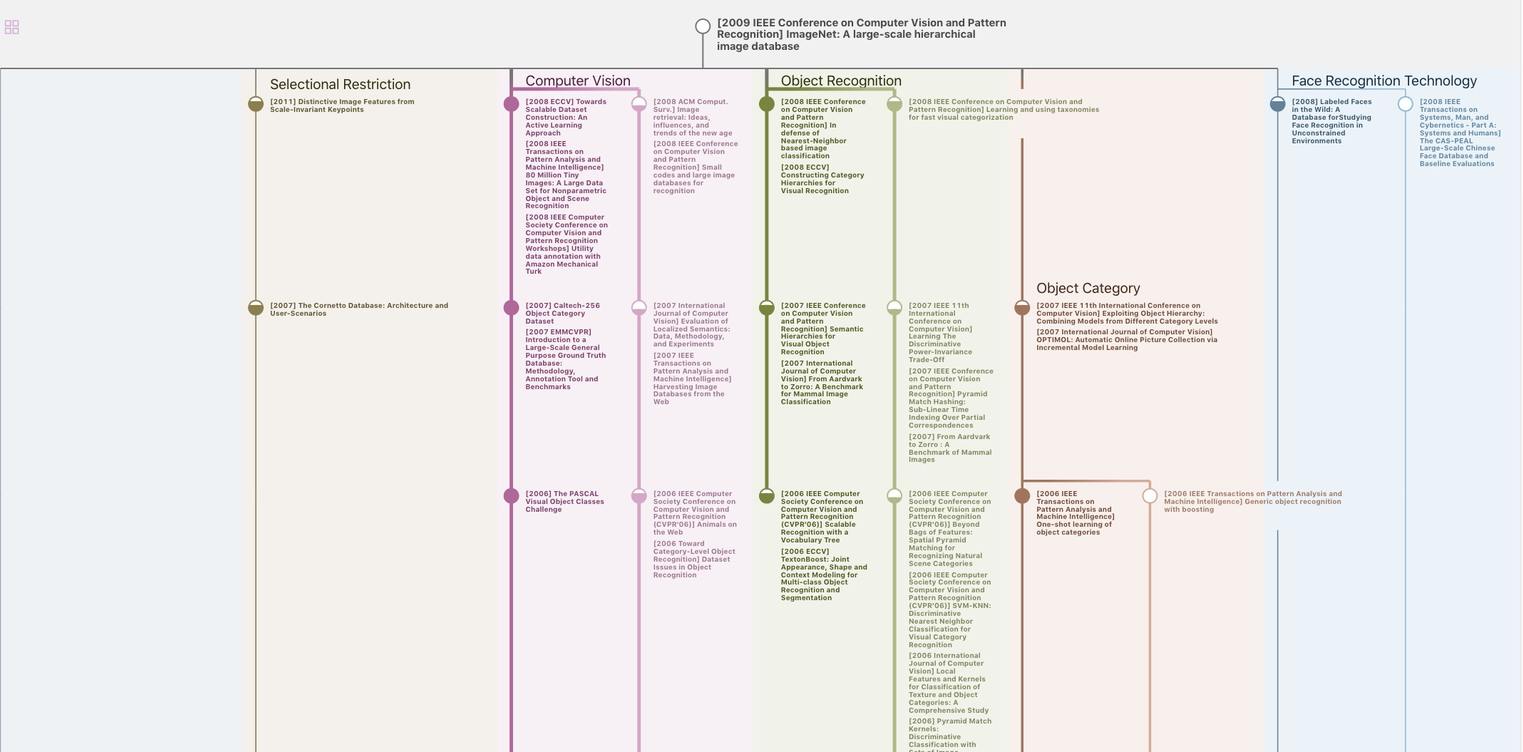
生成溯源树,研究论文发展脉络
Chat Paper
正在生成论文摘要