Imputation of Sensory Properties Using Deep Learning
Journal of computer-aided molecular design(2021)
摘要
Predicting the sensory properties of compounds is challenging due to the subjective nature of the experimental measurements. This testing relies on a panel of human participants and is therefore also expensive and time-consuming. We describe the application of a state-of-the-art deep learning method, Alchemite (TM), to the imputation of sparse physicochemical and sensory data and compare the results with conventional quantitative structure-activity relationship methods and a multi-target graph convolutional neural network. The imputation model achieved a substantially higher accuracy of prediction, with improvements in R-2 between 0.26 and 0.45 over the next best method for each sensory property. We also demonstrate that robust uncertainty estimates generated by the imputation model enable the most accurate predictions to be identified and that imputation also more accurately predicts activity cliffs, where small changes in compound structure result in large changes in sensory properties. In combination, these results demonstrate that the use of imputation, based on data from less expensive, early experiments, enables better selection of compounds for more costly studies, saving experimental time and resources.
更多查看译文
关键词
Sensory properties,In silico model,Deep learning,Imputation,Quantitative structure-activity relationship
AI 理解论文
溯源树
样例
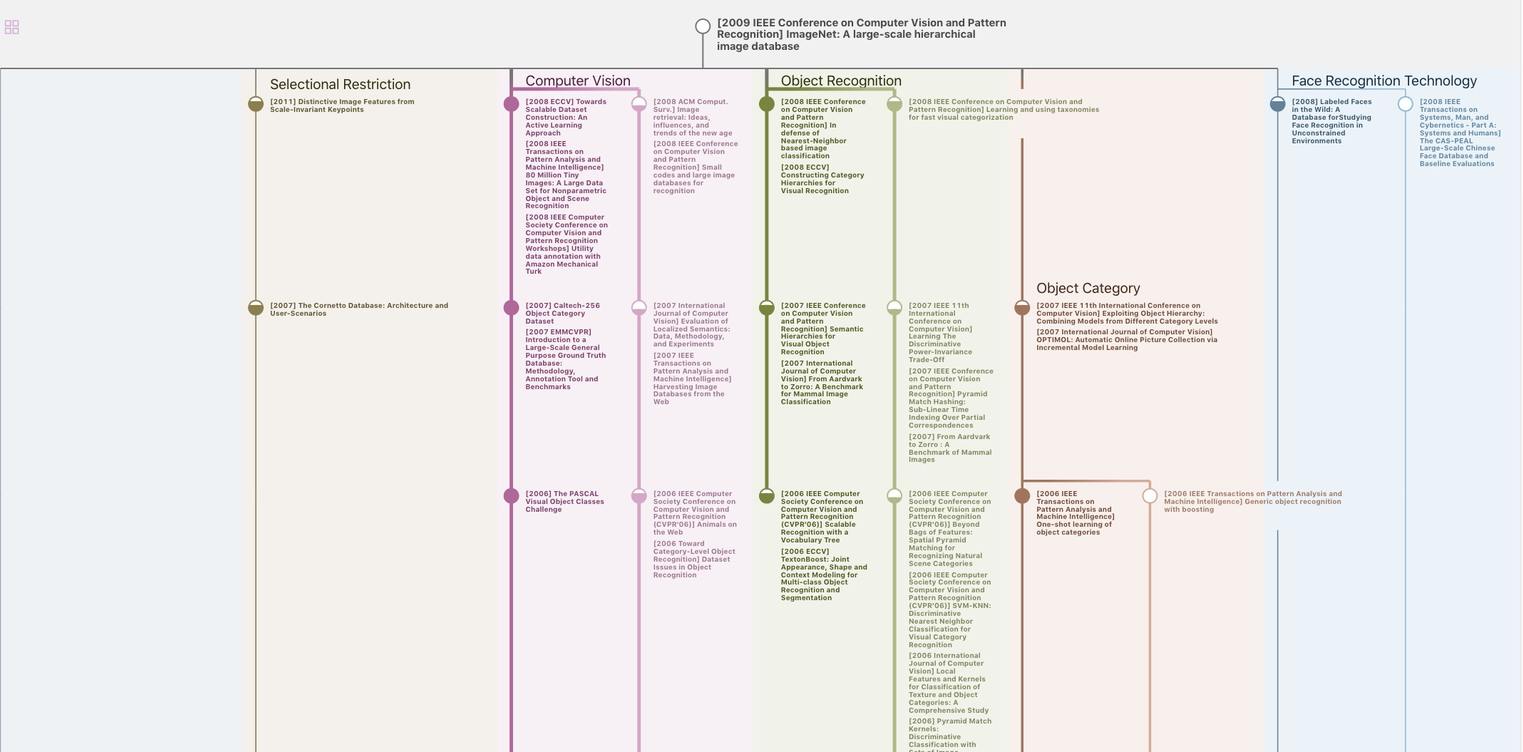
生成溯源树,研究论文发展脉络
Chat Paper
正在生成论文摘要