SARS-CoV-2 in Conjunctiva and Tears and Ocular Symptoms of Patients with COVID-19
Vision(2021)
University of Santiago de Compostela | Department of Ophthalmology | Department of Microbiology | Faculty of Medicine
Abstract
This study investigates the presence of SARS-CoV-2 in conjunctival secretions and tears and evaluates ocular symptoms in a group of patients with COVID-19. We included 56 hospitalized patients with COVID-19 in this cross-sectional cohort study. Conjunctival secretions and tears were collected using flocked swabs and Schirmer strips for SARS-CoV-2 reverse-transcriptase polymerase chain reaction (RT-PCR). Assessment of ocular surface manifestations included an OSDI (Ocular Surface Disease Index) questionnaire. Patients had been admitted to hospital for an average of 2.4 days (range 0–7) and had shown general symptoms for an average of 7.1 days (range 1–20) prior to ocular testing. Four (7.1%) of 56 conjunctival swabs and four (4%) of 112 Schirmer strips were positive for SARS-CoV-2. The mean E-gene cycle threshold values (Ct values) were 31.2 (SD 5.0) in conjunctival swabs and 32.9 (SD 2.7) in left eye Schirmer strips. Overall, 17 (30%) patients presented ocular symptoms. No association was found between positive ocular samples and ocular symptoms. This study shows that SARS-CoV-2 can be detected on the conjunctiva and tears of patients with COVID-19. Contact with the ocular surface may transmit the virus and preventive measures should be taken in this direction.
MoreTranslated text
Key words
COVID-19,coronavirus,SARS-CoV-2,tears,conjunctiva,ocular surface
PDF
View via Publisher
AI Read Science
AI Summary
AI Summary is the key point extracted automatically understanding the full text of the paper, including the background, methods, results, conclusions, icons and other key content, so that you can get the outline of the paper at a glance.
Example
Background
Key content
Introduction
Methods
Results
Related work
Fund
Key content
- Pretraining has recently greatly promoted the development of natural language processing (NLP)
- We show that M6 outperforms the baselines in multimodal downstream tasks, and the large M6 with 10 parameters can reach a better performance
- We propose a method called M6 that is able to process information of multiple modalities and perform both single-modal and cross-modal understanding and generation
- The model is scaled to large model with 10 billion parameters with sophisticated deployment, and the 10 -parameter M6-large is the largest pretrained model in Chinese
- Experimental results show that our proposed M6 outperforms the baseline in a number of downstream tasks concerning both single modality and multiple modalities We will continue the pretraining of extremely large models by increasing data to explore the limit of its performance
Try using models to generate summary,it takes about 60s
Must-Reading Tree
Example
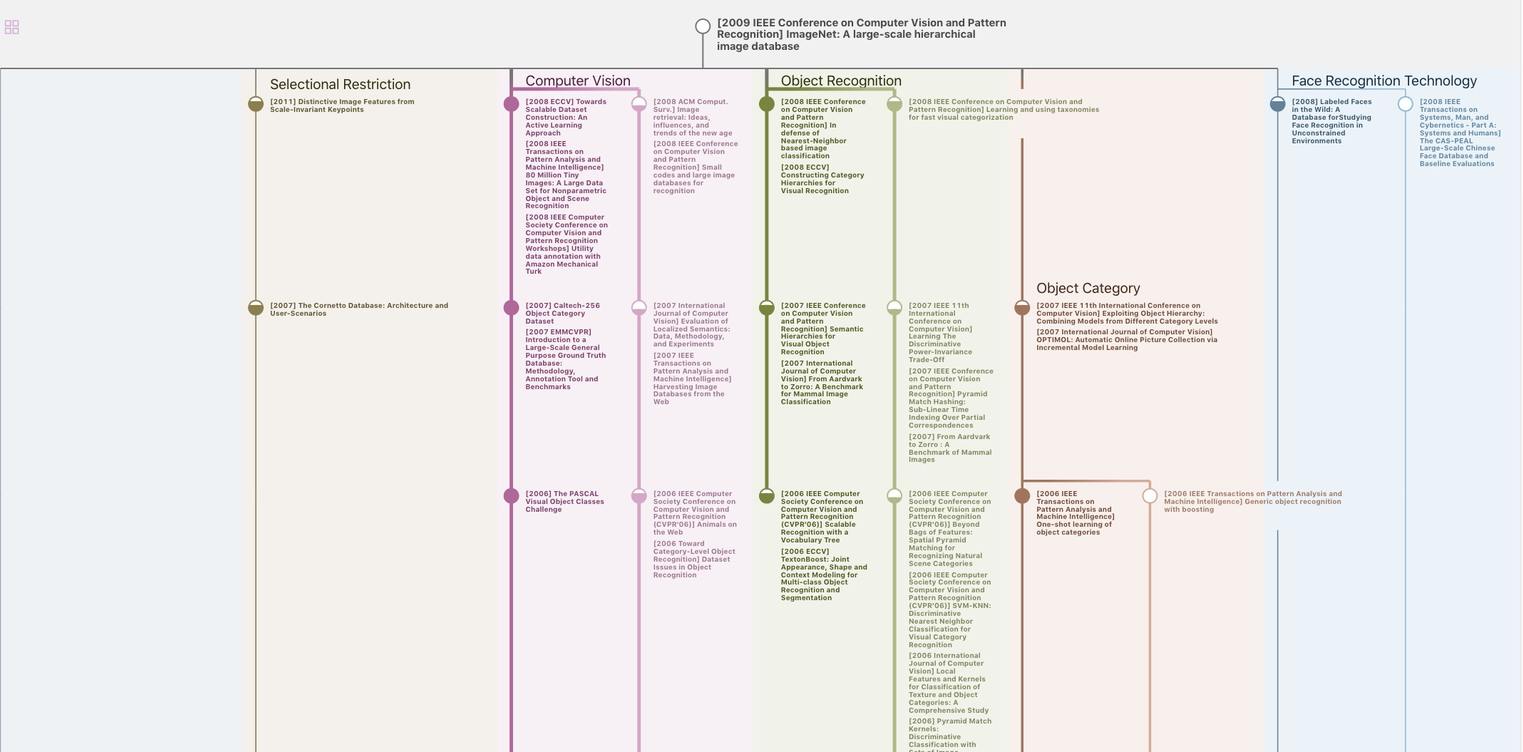
Generate MRT to find the research sequence of this paper
Related Papers
Ocular Symptoms in COVID-19 Infection: a Survey Study
Journal of Ophthalmic Inflammation and Infection 2022
被引用4
Evaluating Ocular Symptoms and Tear Film Cytokine Profiles in Symptomatic COVID-19 Patients
JOURNAL OF CLINICAL MEDICINE 2022
被引用6
Detection of Coronavirus in Tear Samples of Hospitalized Patients with COVID-19.
INTERNATIONAL OPHTHALMOLOGY 2023
被引用8
Journal of Clinical Medicine 2022
被引用3
Nanomaterials to Combat SARS-CoV-2: Strategies to Prevent, Diagnose and Treat COVID-19
Frontiers in bioengineering and biotechnology 2022
被引用2
Virological and Cytological Changes in Tears and Conjunctiva of Patients with COVID-19.
Indian Journal of Ophthalmology 2023
被引用0
Clinical Characteristics and Outcomes of Endophthalmitis Before and During the COVID-19 Pandemic.
JOURNAL OF OPHTHALMIC & VISION RESEARCH 2023
被引用0
Cureus 2023
被引用0
Data Disclaimer
The page data are from open Internet sources, cooperative publishers and automatic analysis results through AI technology. We do not make any commitments and guarantees for the validity, accuracy, correctness, reliability, completeness and timeliness of the page data. If you have any questions, please contact us by email: report@aminer.cn
Chat Paper
去 AI 文献库 对话