Deep Learning for Simultaneous Inference of Hydraulic and Transport Properties
Water resources research(2022)
摘要
Identifying the heterogeneous conductivity field and reconstructing the contaminant release history are key aspects of subsurface remediation. Achieving these two goals with limited and noisy hydraulic head and concentration measurements is challenging. The obstacles include solving an inverse problem for high-dimensional parameters, and the high-computational cost needed for the repeated forward modeling. We use a convolutional adversarial autoencoder (CAAE) for the parameterization of the heterogeneous non-Gaussian conductivity field with a low-dimensional latent representation. Additionally, we trained a three-dimensional dense convolutional encoder-decoder (DenseED) network to serve as the forward surrogate for the flow and transport processes. Combining the CAAE and DenseED forward surrogate models, the ensemble smoother with multiple data assimilation (ESMDA) algorithm is used to sample from the Bayesian posterior distribution of the unknown parameters, forming a CAAE-DenseED-ESMDA inversion framework. We applied this CAAE-DenseED-ESMDA inversion framework in a three-dimensional contaminant source and conductivity field identification problem. A comparison of the inversion results from CAAE-ESMDA with physical flow and transport simulator and CAAE-DenseED-ESMDA is provided, showing that accurate reconstruction results were achieved with a much higher computational efficiency.
更多查看译文
关键词
subsurface,inverse problem,source indentification,neural network,adversarial
AI 理解论文
溯源树
样例
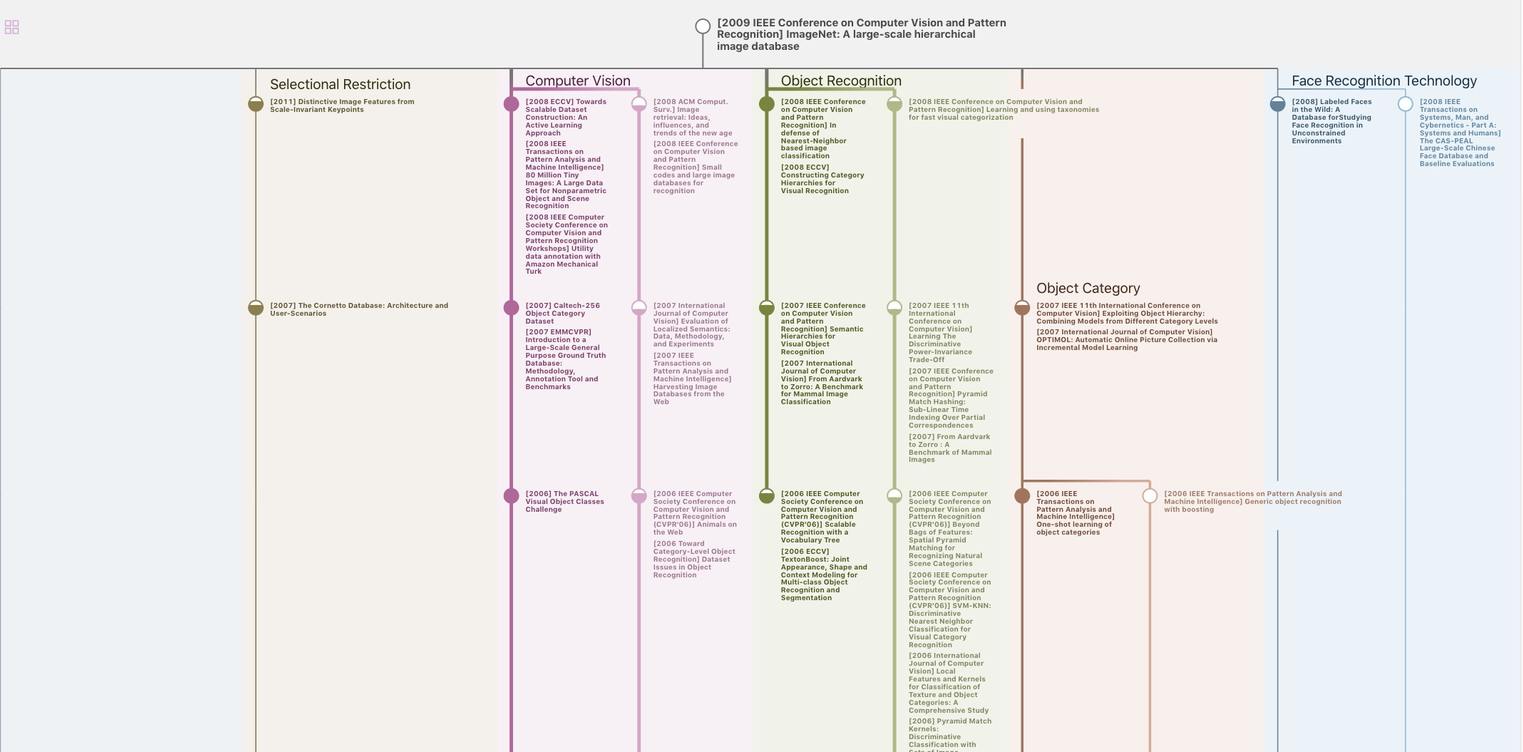
生成溯源树,研究论文发展脉络
Chat Paper
正在生成论文摘要