Uncertainty Quantification For Low-Rank Matrix Completion With Heterogeneous and Sub-Exponential Noise
INTERNATIONAL CONFERENCE ON ARTIFICIAL INTELLIGENCE AND STATISTICS, VOL 151(2022)
Abstract
The problem of low-rank matrix completion with heterogeneous and sub-exponential (as opposed to homogeneous and Gaussian) noise is particularly relevant to a number of applications in modern commerce. Examples include panel sales data and data collected from web-commerce systems such as recommendation engines. An important unresolved question for this problem is characterizing the distribution of estimated matrix entries under common low-rank estimators. Such a characterization is essential to any application that requires quantification of uncertainty in these estimates and has heretofore only been available under the assumption of homogenous Gaussian noise. Here we characterize the distribution of estimated matrix entries when the observation noise is heterogeneous sub-exponential and provide, as an application, explicit formulas for this distribution when observed entries are Poisson or Binary distributed.
MoreTranslated text
Key words
uncertainty quantification,matrix,low-rank,sub-exponential
AI Read Science
Must-Reading Tree
Example
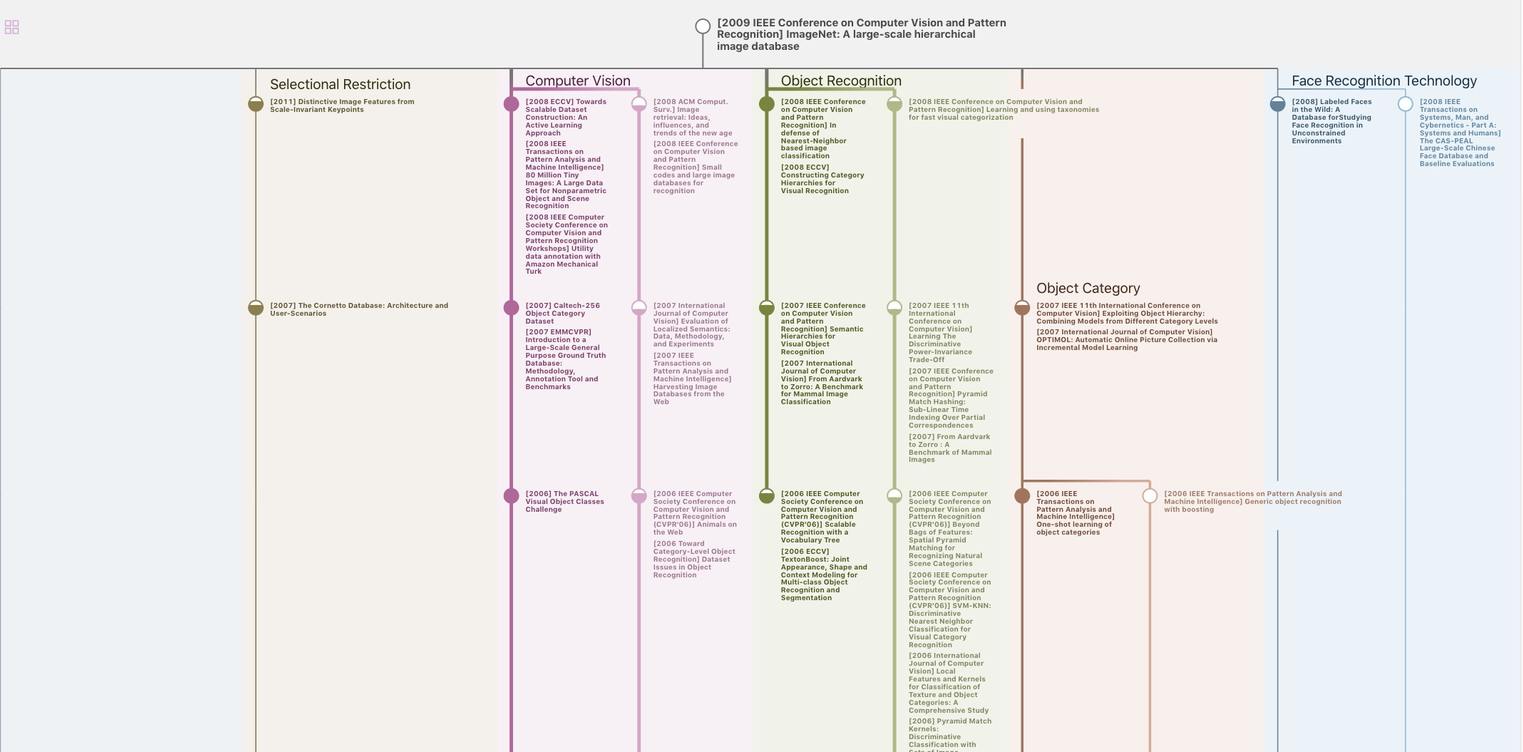
Generate MRT to find the research sequence of this paper
Chat Paper
Summary is being generated by the instructions you defined