Learning Disentangled Factors from Paired Data in Cross-Modal Retrieval: An Implicit Identifiable VAE Approach
International Multimedia Conference(2021)
摘要
ABSTRACTWe tackle the problem of learning the underlying disentangled latent factors that are shared between the paired bi-modal data in cross-modal retrieval. Typically the data in both modalities are complex, structured, and high dimensional (e.g., image and text), for which the conventional deep auto-encoding latent variable models such as the Variational Autoencoder (VAE) often suffer from difficulty of accurate decoder training or realistic synthesis. In this paper we propose a novel idea of the implicit decoder, which completely removes the ambient data decoding module from a latent variable model, via implicit encoder inversion that is achieved by Jacobian regularization of the low-dimensional embedding function. Motivated from the recent Identifiable-VAE (IVAE) model, we modify it to incorporate the query modality data as conditioning auxiliary input, which allows us to prove that the true parameters of the model can be identifiable under some regularity conditions. Tested on various datasets where the true factors are fully/partially available, our model is shown to identify the factors accurately, significantly outperforming conventional latent variable models.
更多查看译文
AI 理解论文
溯源树
样例
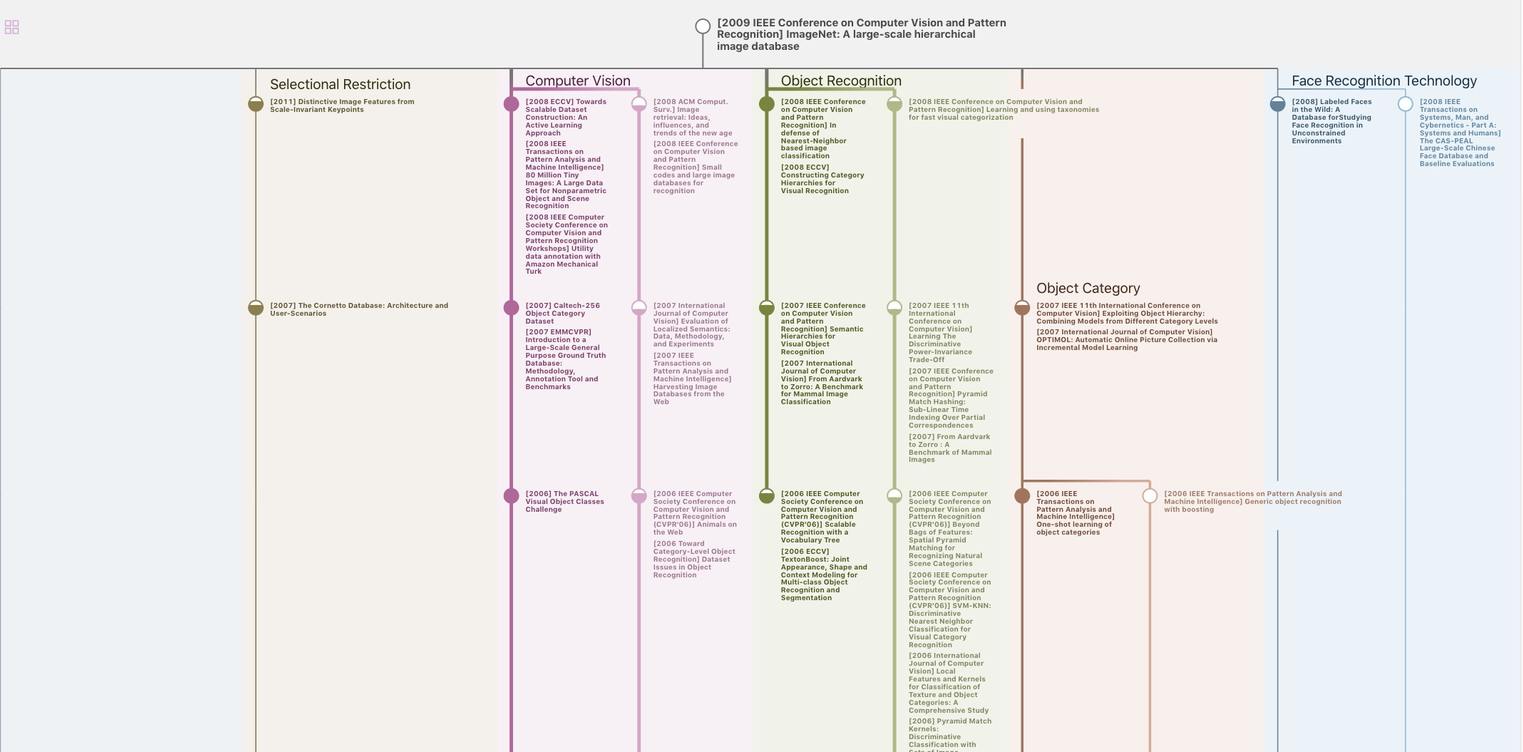
生成溯源树,研究论文发展脉络
Chat Paper
正在生成论文摘要