Stateful Offline Contextual Policy Evaluation and Learning
INTERNATIONAL CONFERENCE ON ARTIFICIAL INTELLIGENCE AND STATISTICS, VOL 151(2022)
摘要
We study off-policy evaluation and learning from sequential data in a structured class of Markov decision processes that arise from repeated interactions with an exogenous sequence of arrivals with contexts, which generate unknown individual-level responses to agent actions that induce known transitions. This is a relevant model, for example, for dynamic personalized pricing and other operations management problems in the presence of potentially high-dimensional user types. The individual-level response is not causally affected by the state variable. In this setting, we adapt doubly-robust estimation in the single-timestep setting to the sequential setting so that a state-dependent policy can be learned even from a single timestep's worth of data. We introduce a marginal MDP model and study an algorithm for off-policy learning, which can be viewed as fitted value iteration in the marginal MDP. We also provide structural results on when errors in the response model leads to the persistence, rather than attenuation, of error over time. In simulations, we show that the advantages of doubly-robust estimation in the single time-step setting, via unbiased and lower-variance estimation, can directly translate to improved out-of-sample policy performance. This structure-specific analysis sheds light on the underlying structure on a class of problems, operations research/management problems, often heralded as a real-world domain for offline RL, which are in fact qualitatively easier.
更多查看译文
关键词
policy evaluation,learning
AI 理解论文
溯源树
样例
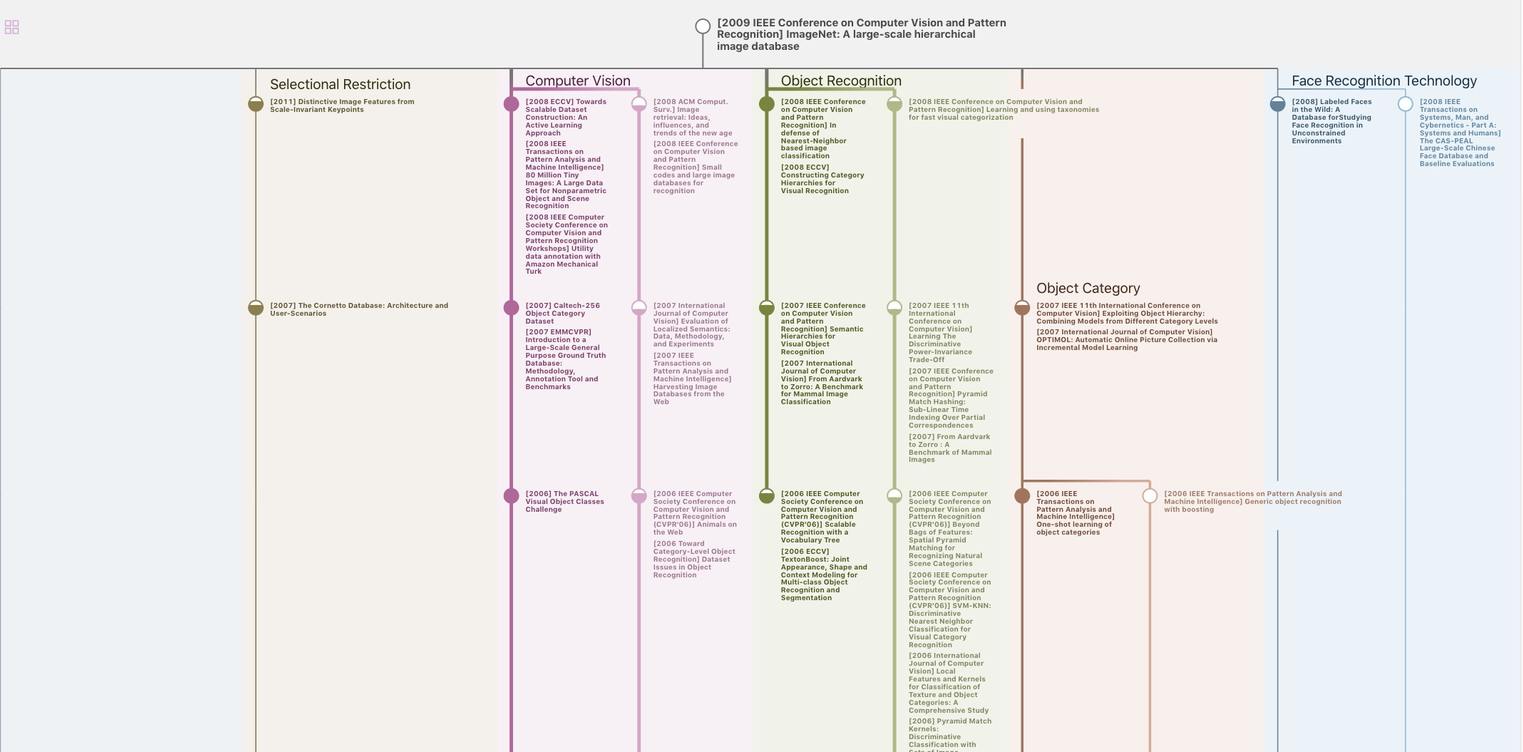
生成溯源树,研究论文发展脉络
Chat Paper
正在生成论文摘要