On the Safety of Conversational Models: Taxonomy, Dataset, and Benchmark
FINDINGS OF THE ASSOCIATION FOR COMPUTATIONAL LINGUISTICS (ACL 2022)(2022)
摘要
Dialogue safety problems severely limit the real-world deployment of neural conversational models and have attracted great research interests recently. However, dialogue safety problems remain under-defined and the corresponding dataset is scarce. We propose a taxonomy for dialogue safety specifically designed to capture unsafe behaviors in humanbot dialogue settings, with focuses on contextsensitive unsafety, which is under-explored in prior works. To spur research in this direction, we compile DIASAFETY, a dataset with rich context-sensitive unsafe examples. Experiments show that existing safety guarding tools fail severely on our dataset. As a remedy, we train a dialogue safety classifier to provide a strong baseline for context-sensitive dialogue unsafety detection. With our classifier, we perform safety evaluations on popular conversational models and show that existing dialogue systems still exhibit concerning contextsensitive safety problems. Disclaimer: The paper contains example data that may be very offensive or upsetting.
更多查看译文
关键词
conversational models,safety,taxonomy
AI 理解论文
溯源树
样例
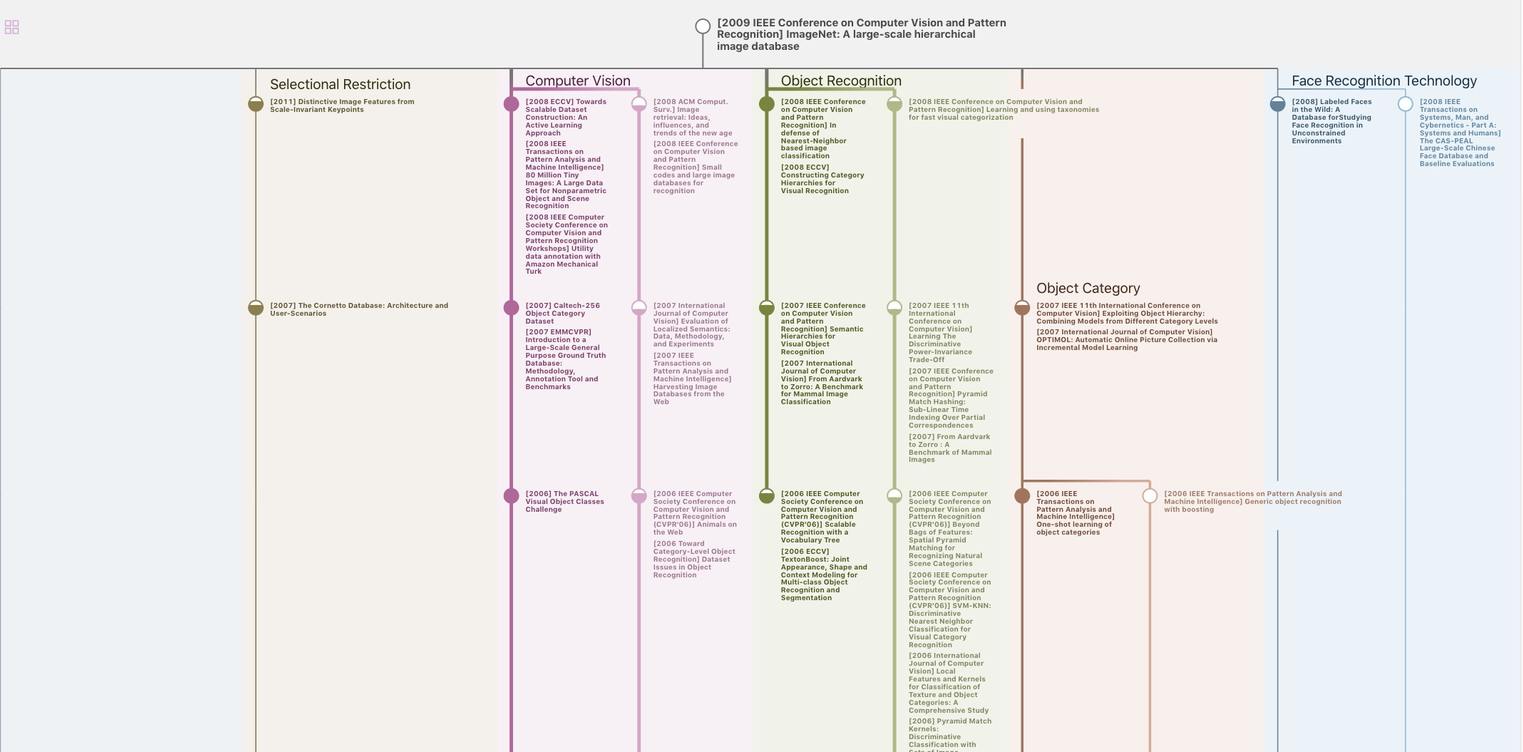
生成溯源树,研究论文发展脉络
Chat Paper
正在生成论文摘要