Multitask Prompted Training Enables Zero-Shot Task Generalization
International Conference on Learning Representations (ICLR)(2022)
摘要
Large language models have recently been shown to attain reasonable zero-shot generalization on a diverse set of tasks. It has been hypothesized that this is a consequence of implicit multitask learning in language model training. Can zero-shot generalization instead be directly induced by explicit multitask learning? To test this question at scale, we develop a system for easily mapping general natural language tasks into a human-readable prompted form. We convert a large set of supervised datasets, each with multiple prompts using varying natural language. These prompted datasets allow for benchmarking the ability of a model to perform completely unseen tasks specified in natural language. We fine-tune a pretrained encoder-decoder model on this multitask mixture covering a wide variety of tasks. The model attains strong zero-shot performance on several standard datasets, often outperforming models 16x its size. Further, our approach attains strong performance on a subset of tasks from the BIG-Bench benchmark, outperforming models 6x its size. All prompts and trained models are available at github.com/bigscience-workshop/promptsource/.
更多查看译文
关键词
multitask,generalization,training,zero-shot
AI 理解论文
溯源树
样例
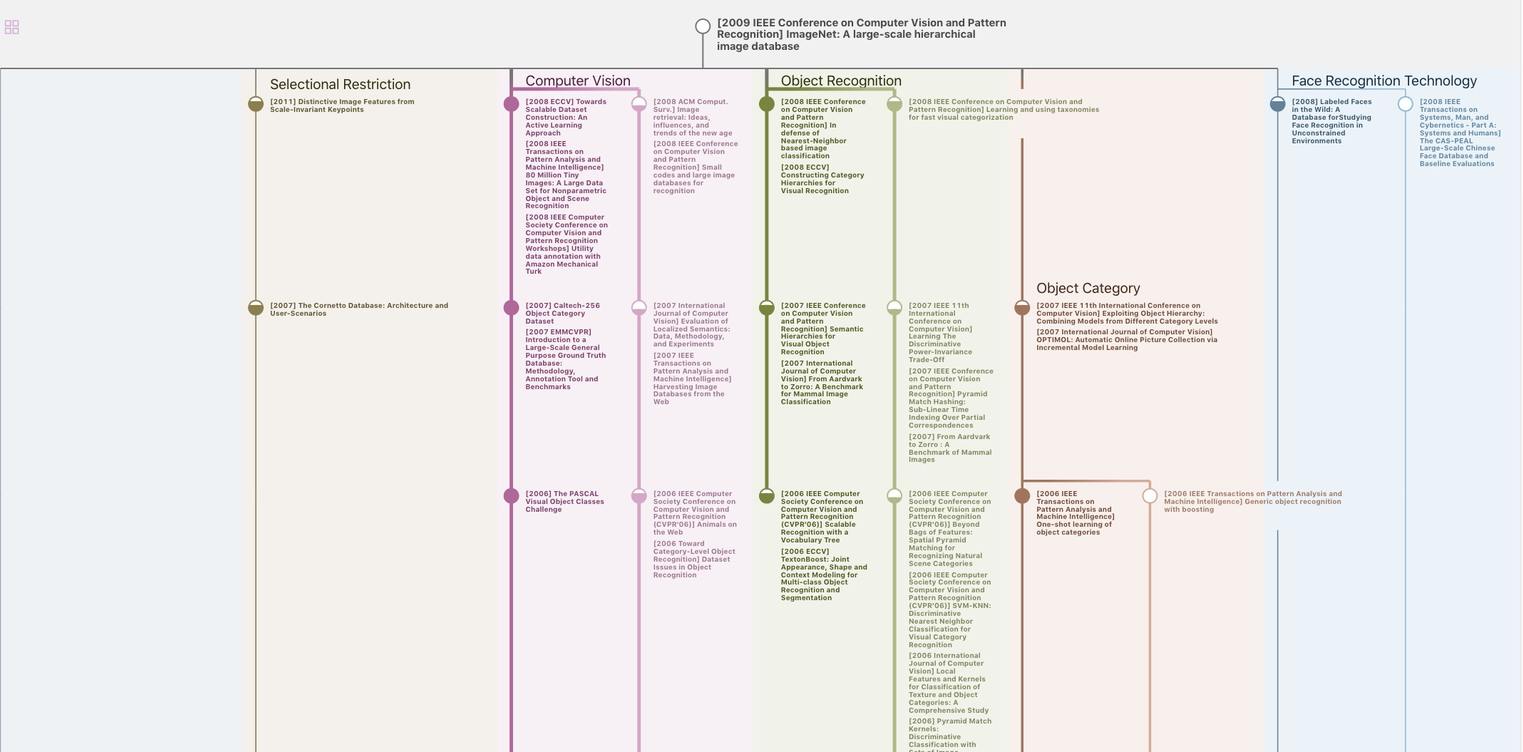
生成溯源树,研究论文发展脉络
Chat Paper
正在生成论文摘要