SGoLAM: Simultaneous Goal Localization and Mapping for Multi-Object Goal Navigation
CoRR(2021)
Abstract
We present SGoLAM, short for simultaneous goal localization and mapping, which is a simple and efficient algorithm for Multi-Object Goal navigation. Given an agent equipped with an RGB-D camera and a GPS/Compass sensor, our objective is to have the agent navigate to a sequence of target objects in realistic 3D environments. Our pipeline fully leverages the strength of classical approaches for visual navigation, by decomposing the problem into two key components: mapping and goal localization. The mapping module converts the depth observations into an occupancy map, and the goal localization module marks the locations of goal objects. The agent's policy is determined using the information provided by the two modules: if a current goal is found, plan towards the goal and otherwise, perform exploration. As our approach does not require any training of neural networks, it could be used in an off-the-shelf manner, and amenable for fast generalization in new, unseen environments. Nonetheless, our approach performs on par with the state-of-the-art learning-based approaches. SGoLAM is ranked 2nd in the CVPR 2021 MultiON (Multi-Object Goal Navigation) challenge. We have made our code publicly available at \emph{https://github.com/eunsunlee/SGoLAM}.
MoreTranslated text
Key words
simultaneous goal localization,mapping,multi-object
AI Read Science
Must-Reading Tree
Example
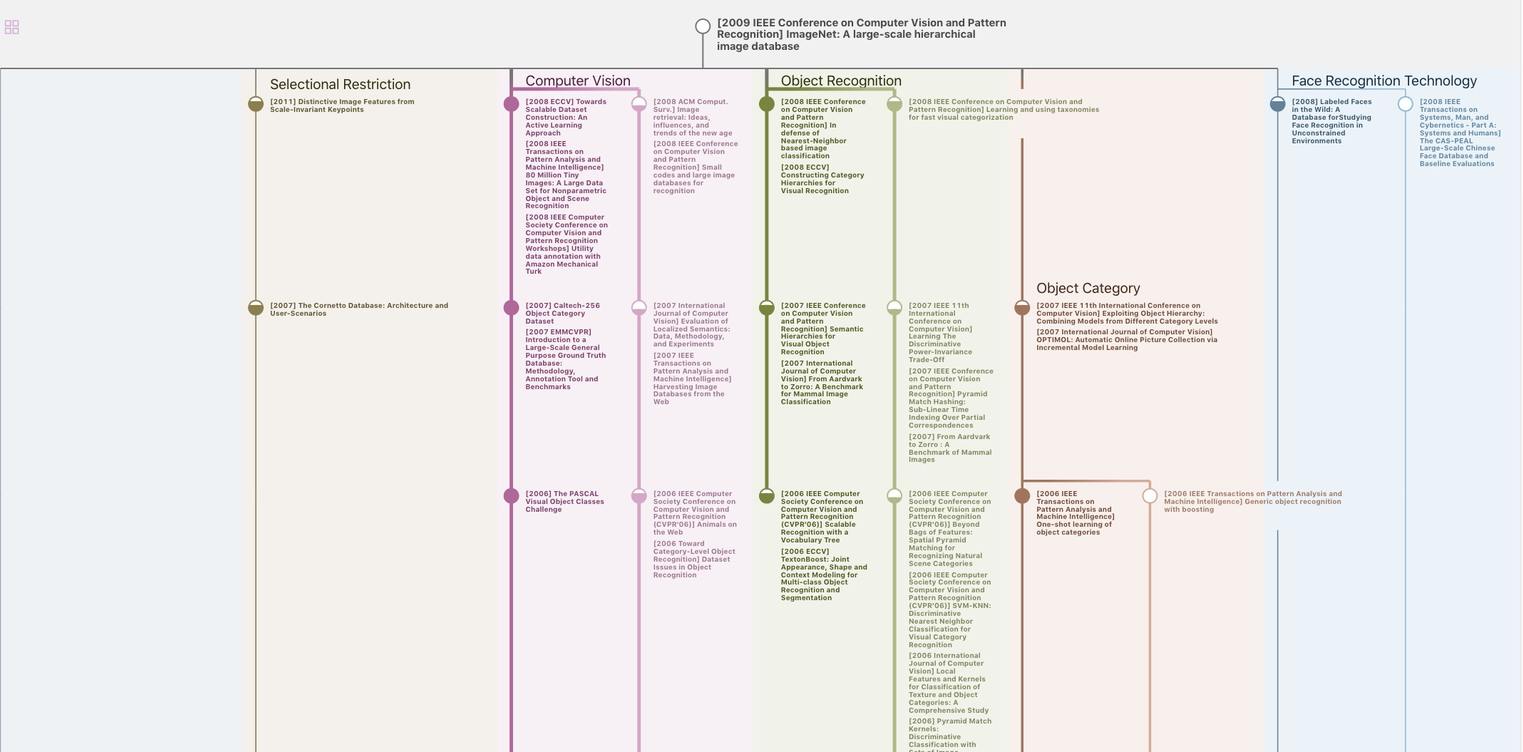
Generate MRT to find the research sequence of this paper
Chat Paper
Summary is being generated by the instructions you defined