Embed Everything: A Method for Efficiently Co-Embedding Multi-Modal Spaces
ArXiv(2021)
摘要
Any general artificial intelligence system must be able to interpret, operate on, and produce data in a multi-modal latent space that can represent audio, imagery, text, and more. In the last decade, deep neural networks have seen remarkable success in unimodal data distributions, while transfer learning techniques have seen a massive expansion of model reuse across related domains. However, training multi-modal networks from scratch remains expensive and illusive, while heterogeneous transfer learning (HTL) techniques remain relatively underdeveloped. In this paper, we propose a novel and cost-effective HTL strategy for co-embedding multi-modal spaces. Our method avoids cost inefficiencies by preprocessing embeddings using pretrained models for all components, without passing gradients through these models. We prove the use of this system in a joint image-audio embedding task. Our method has wide-reaching applications, as successfully bridging the gap between different latent spaces could provide a framework for the promised "universal" embedding.
更多查看译文
关键词
spaces,co-embedding,multi-modal
AI 理解论文
溯源树
样例
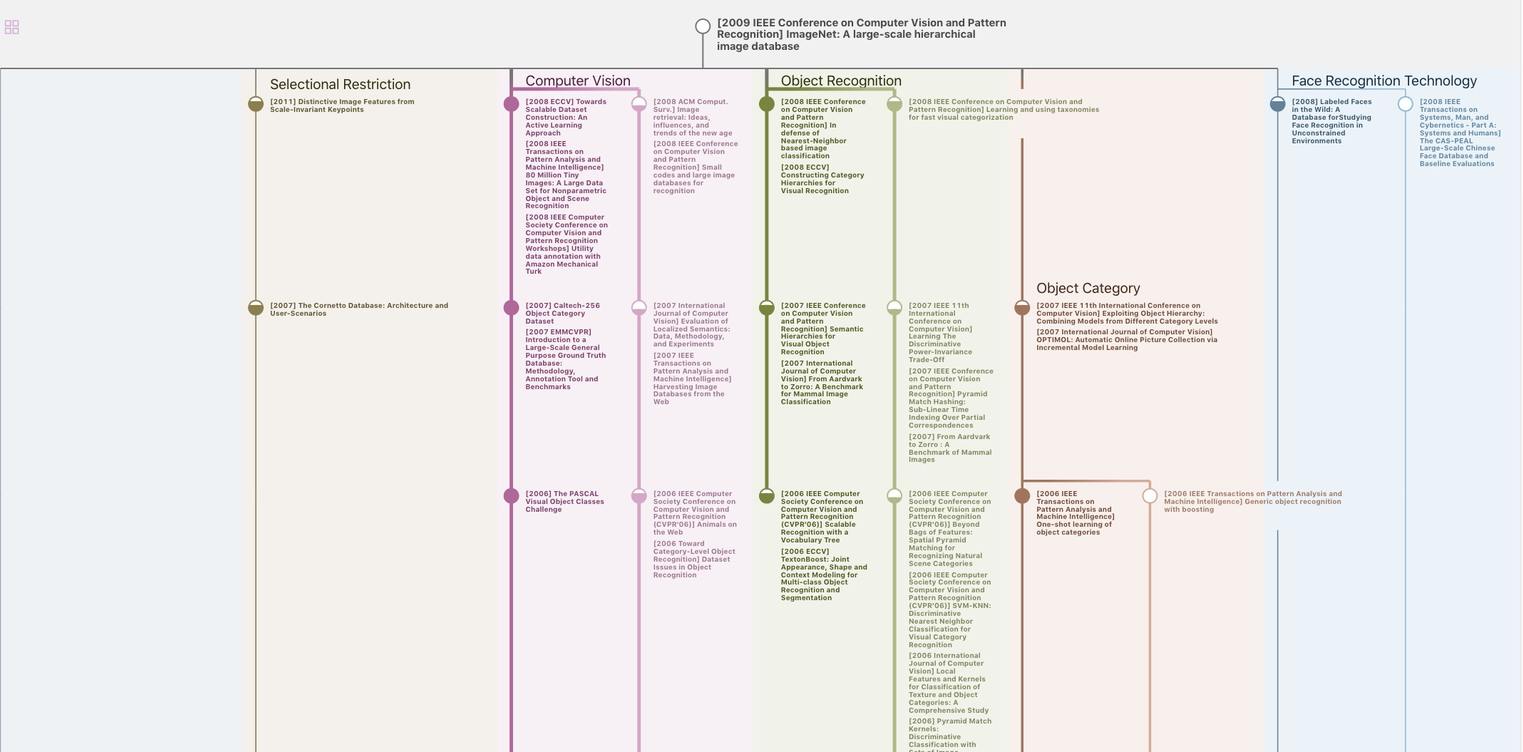
生成溯源树,研究论文发展脉络
Chat Paper
正在生成论文摘要