Cellular Junction and Mesenchymal Factors Delineate an Endometriosis-Specific Response of Endometrial Stromal Cells to the Mesothelium
Molecular and Cellular Endocrinology(2022)SCI 2区SCI 3区
Univ Texas Hlth San Antonio | Madigan Army Med Ctr | UT Hlth San Antonio | 8403 Floyd Curl Dr
Abstract
Endometriosis is a debilitating gynecologic disorder that affects ∼10% of women of reproductive age. Endometriosis is characterized by growth of endometriosis lesions within the abdominal cavity, generally thought to arise from retrograde menstruation of shed endometrial tissue. While the pathophysiology underlying peritoneal endometriosis lesion formation is still unclear, the interaction between invading endometrial tissue and the peritoneal mesothelial lining is an essential step in lesion formation. In this study, we assessed proteomic differences between eutopic endometrial stromal cells (ESCs) from women with and without endometriosis in response to peritoneal mesothelial cell (PMC) exposure, using single-cell cytometry by time-of-flight (CyTOF). Co-cultured primary eutopic ESCs from women with and without endometriosis with an established PMC line were subjected to immunostaining with a panel of Maxpar CyTOF metal-conjugated antibodies (n = 28) targeting cell junction and mesenchymal markers, which are involved in cell-cell adhesions and epithelial-mesenchymal transition. Exposure of the ESCs to PMCs resulted in a drastic shift in cellular expression profiles in ESCs derived from endometriosis, whereas little effect by PMCs was observed in ESCs from non-endometriosis subjects. The transcription factor SNAI1 was consistently repressed by PMC interactions. ESCs from endometriosis patients are unique in that they respond to PMCs by undergoing changes in adhesive properties and mesenchymal characteristics that would facilitate lesion formation.
MoreTranslated text
Key words
Endometriosis,Cellular adhesion,Mesenchymal factors,CyTOF,Mass cyrometry
求助PDF
上传PDF
View via Publisher
AI Read Science
AI Summary
AI Summary is the key point extracted automatically understanding the full text of the paper, including the background, methods, results, conclusions, icons and other key content, so that you can get the outline of the paper at a glance.
Example
Background
Key content
Introduction
Methods
Results
Related work
Fund
Key content
- Pretraining has recently greatly promoted the development of natural language processing (NLP)
- We show that M6 outperforms the baselines in multimodal downstream tasks, and the large M6 with 10 parameters can reach a better performance
- We propose a method called M6 that is able to process information of multiple modalities and perform both single-modal and cross-modal understanding and generation
- The model is scaled to large model with 10 billion parameters with sophisticated deployment, and the 10 -parameter M6-large is the largest pretrained model in Chinese
- Experimental results show that our proposed M6 outperforms the baseline in a number of downstream tasks concerning both single modality and multiple modalities We will continue the pretraining of extremely large models by increasing data to explore the limit of its performance
Upload PDF to Generate Summary
Must-Reading Tree
Example
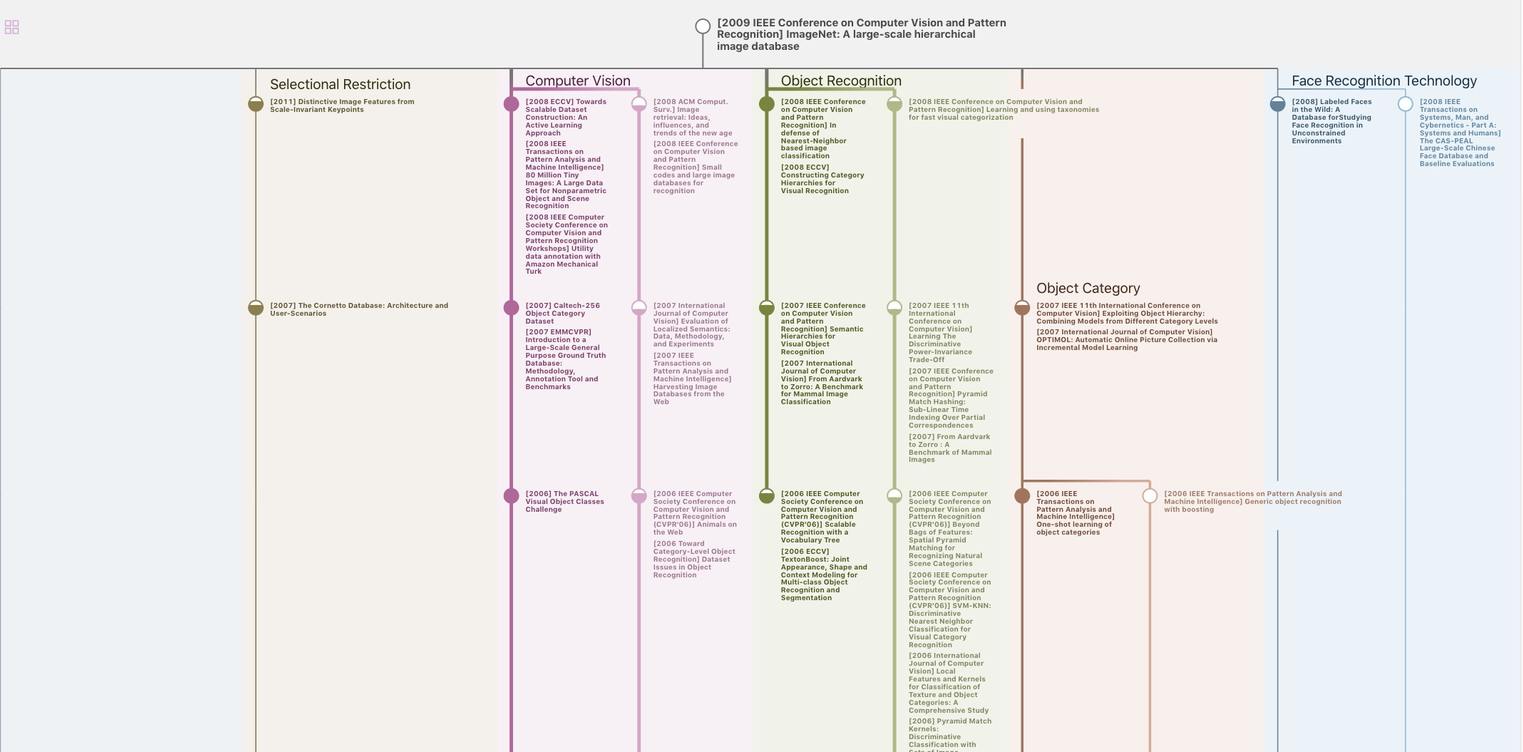
Generate MRT to find the research sequence of this paper
Related Papers
2000
被引用188 | 浏览
2006
被引用218 | 浏览
2003
被引用143 | 浏览
1993
被引用80 | 浏览
2009
被引用35 | 浏览
2014
被引用132 | 浏览
2011
被引用645 | 浏览
2002
被引用179 | 浏览
2012
被引用88 | 浏览
2003
被引用632 | 浏览
2016
被引用286 | 浏览
2016
被引用53 | 浏览
2017
被引用96 | 浏览
2020
被引用50 | 浏览
Data Disclaimer
The page data are from open Internet sources, cooperative publishers and automatic analysis results through AI technology. We do not make any commitments and guarantees for the validity, accuracy, correctness, reliability, completeness and timeliness of the page data. If you have any questions, please contact us by email: report@aminer.cn
Chat Paper