Data-Driven Synthesis Optimization of Cathode Materials
ECS Meeting Abstracts(2020)
摘要
Machine intelligence-based optimizing synthesis parameters offers exciting opportunities in various domain of materials chemistry where large and comprehensive dataset are available. Aiming to construct a “design-to-device” pipeline for battery application with small discrepancy, we adapt machine learning model based on actual experimental data from curated literatures to predict the discharge capacity of Nickel-Manganese-Cobalt (NCM) cathode material. However, missing data is an inherent problem from the results based on literature. Herein we introduce imputation techniques to fill the missing data based. To this end, we show that our model have remarkable predictive capability with the average coefficient of determinant, R2 = 0.84 for training sets. In addition, we also validate our model prediction by the experiments, which yields in less than 8% error. The present work highlights a promising data driven materials synthesis approach, which underpins nonlinear structure-properties relationship for broad range of materials development.
更多查看译文
关键词
Cathode,Engineering physics,Materials science,Data-driven
AI 理解论文
溯源树
样例
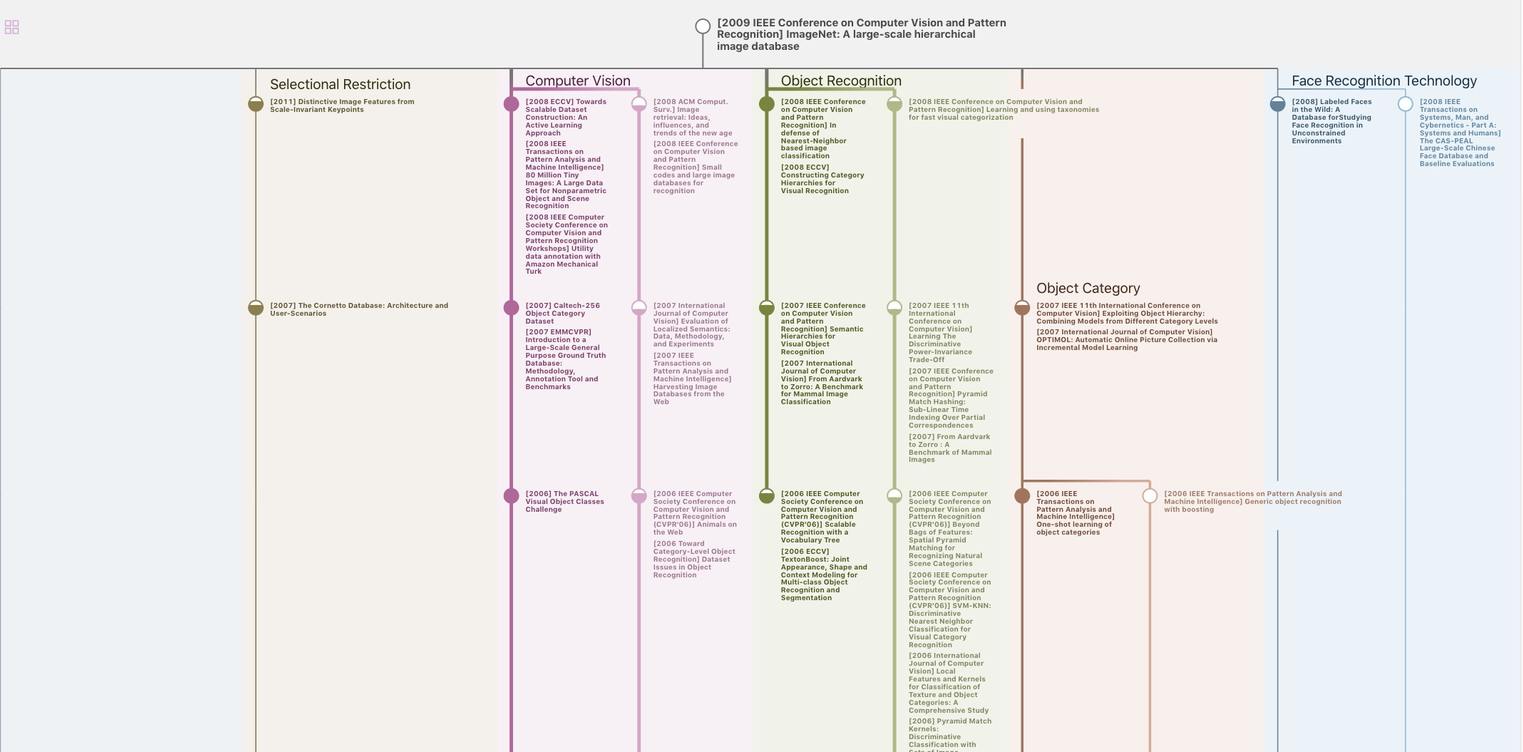
生成溯源树,研究论文发展脉络
Chat Paper
正在生成论文摘要