Prediction of operative mortality for patients undergoing cardiac surgical procedures without established risk scores
The Journal of thoracic and cardiovascular surgery(2023)
摘要
Objective: Current cardiac surgery risk models do not address a substantial frac-tion of procedures. We sought to create models to predict the risk of operative mortality for an expanded set of cases. Methods: Four supervised machine learning models were trained using preopera-tive variables present in the Society of Thoracic Surgeons (STS) data set of the Massachusetts General Hospital to predict and classify operative mortality in procedures without STS risk scores. A total of 424 (5.5%) mortality events occurred out of 7745 cases. Models included logistic regression with elastic net reg-ularization (LogReg), support vector machine, random forest (RF), and extreme gradient boosted trees (XGBoost). Model discrimination was assessed via area un-der the receiver operating characteristic curve (AUC), and calibration was assessed via calibration slope and expected-to-observed event ratio. External validation was performed using STS data sets from Brigham and Women's Hospital (BWH) and the Johns Hopkins Hospital (JHH).Results: Models performed comparably with the highest mean AUC of 0.83 (RF) and expected-to-observed event ratio of 1.00. On external validation, the AUC was 0.81 in BWH (RF) and 0.79 in JHH (LogReg/RF). Models trained and applied on the same institution's data achieved AUCs of 0.81 (BWH: LogReg/RF/XGBoost) and 0.82 (JHH: LogReg/RF/XGBoost). Conclusions: Machine learning models trained on preoperative patient data can predict operative mortality at a high level of accuracy for cardiac surgical proced-ures without established risk scores. Such procedures comprise 23% of all cardiac surgical procedures nationwide. This work also highlights the value of using local institutional data to train new prediction models that account for institution -specific practices. (J Thorac Cardiovasc Surg 2023;165:1449-59)
更多查看译文
AI 理解论文
溯源树
样例
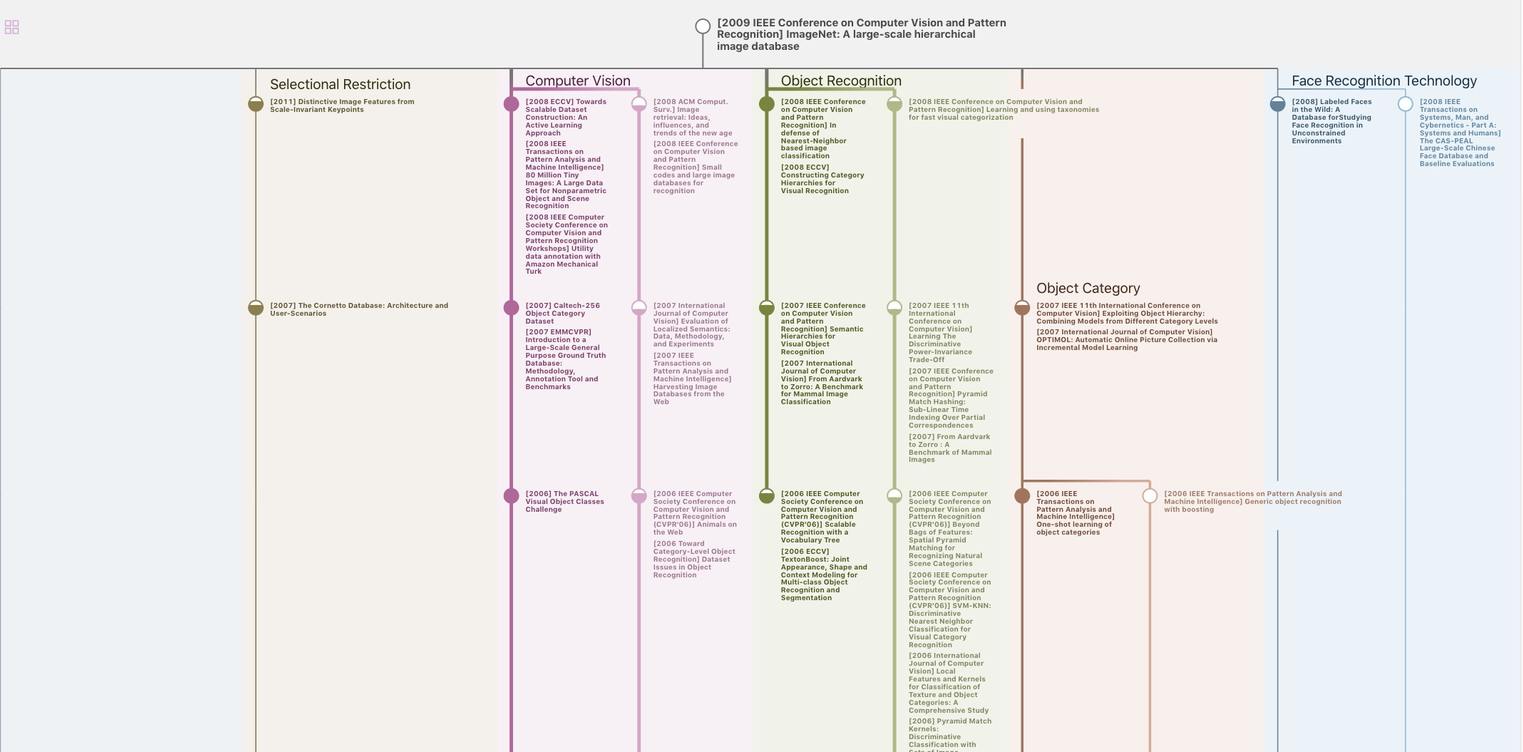
生成溯源树,研究论文发展脉络
Chat Paper
正在生成论文摘要