Incremental Layer-Wise Self-Supervised Learning for Efficient Unsupervised Speech Domain Adaptation On Device
arxiv(2022)
摘要
Streaming end-to-end speech recognition models have been widely applied to mobile devices and show significant improvement in efficiency. These models are typically trained on the server using transcribed speech data. However, the server data distribution can be very different from the data distribution on user devices, which could affect the model performance. There are two main challenges for on device training, limited reliable labels and limited training memory. While self-supervised learning algorithms can mitigate the mismatch between domains using unlabeled data, they are not applicable on mobile devices directly because of the memory constraint. In this paper, we propose an incremental layer-wise self-supervised learning algorithm for efficient unsupervised speech domain adaptation on mobile devices, in which only one layer is updated at a time. Extensive experimental results demonstrate that the proposed algorithm achieves a 24.2% relative Word Error Rate (WER) improvement on the target domain compared to a supervised baseline and costs 95.7% less training memory than the end-to-end self-supervised learning algorithm.
更多查看译文
关键词
adaptation,learning,layer-wise,self-supervised
AI 理解论文
溯源树
样例
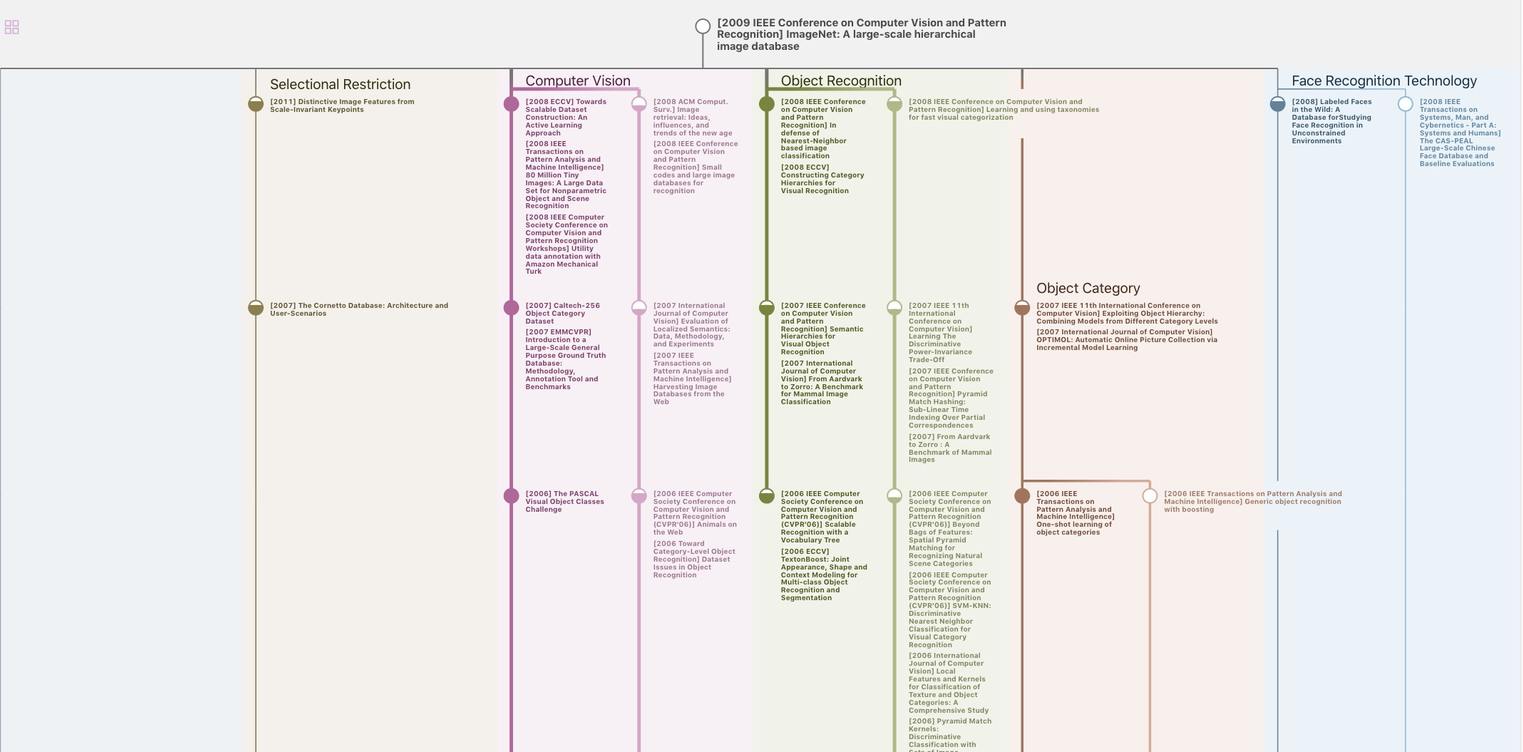
生成溯源树,研究论文发展脉络
Chat Paper
正在生成论文摘要