Multi-instance Conditional Autoencoder a Data Driven Compression Model for Strongly Correlated Datasets
2021 IEEE INTERNATIONAL SYMPOSIUM ON BROADBAND MULTIMEDIA SYSTEMS AND BROADCASTING (BMSB)(2021)
摘要
Multimedia compression is an essential data processing procedure to any Content Delivery Network (CDN). Autoencoder is a data driven model which compresses the high dimensional inputs into a low dimensional latent representation creating a bottleneck impelling the model to learn a compression which is able to reconstruct the input with minimum error. The model searches for patterns in the data to reduce the input dimensionality fitting the input through the bottleneck while minimizing the reconstruction loss. Conventional autoencoder's latent representation exploits intra-sample correlation without taking advantage of the inter-samples correlation. Our model, Multi-instance Conditional Autoencoder, compresses many files to comprise one latent representation, and then restore each file individually in the decoding phase. We achieved higher compression rate compared to traditional autoencoder in case of audio files, Javanese and VoxCeleb datasets, where data correlation is sufficiently high.
更多查看译文
关键词
Digital multimedia broadcasting,Correlation,Preforms,Content distribution networks,Fitting,Games,Data processing
AI 理解论文
溯源树
样例
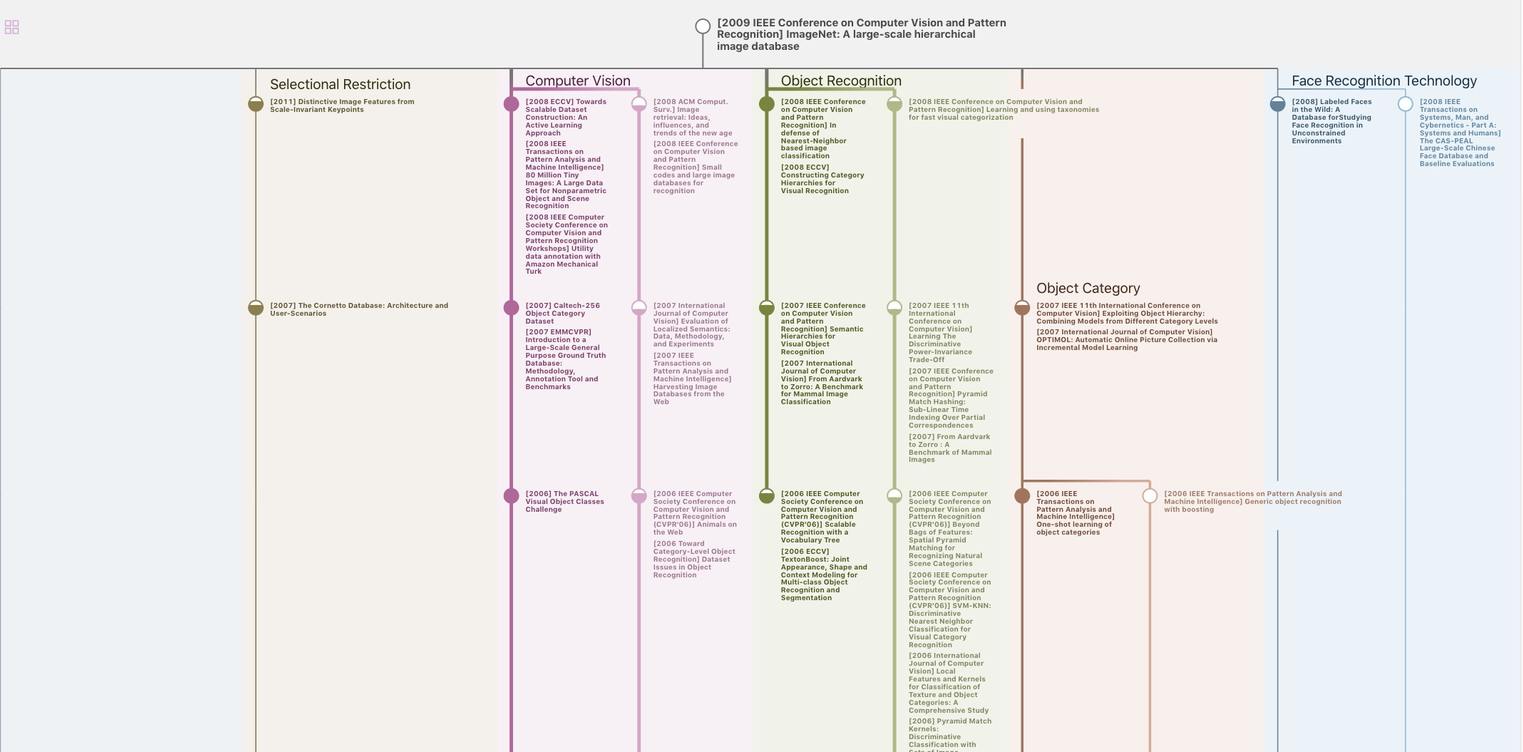
生成溯源树,研究论文发展脉络
Chat Paper
正在生成论文摘要