A Critical Empirical Study of Black-box Explanations in AI.
International Conference on Information Systems (ICIS)(2021)
摘要
This paper provides empirical concerns about post-hoc explanations of black-box ML models, one of the major trends in AI explainability (XAI), by showing its lack of interpretability and societal consequences. Using a representative consumer panel to test our assumptions, we report three main findings. First, we show that post-hoc explanations of black-box model tend to give partial and biased information on the underlying mechanism of the algorithm and can be subject to manipulation or information withholding by diverting users' attention. Secondly, we show the importance of tested behavioral indicators, in addition to self-reported perceived indicators, to provide a more comprehensive view of the dimensions of interpretability. This paper contributes to shedding new light on the actual theoretical debate between intrinsically transparent AI models and post-hoc explanations of black-box complex models-a debate which is likely to play a highly influential role in the future development and operationalization of AI systems.
更多查看译文
关键词
ai,explanations,critical empirical study,empirical study,black-box
AI 理解论文
溯源树
样例
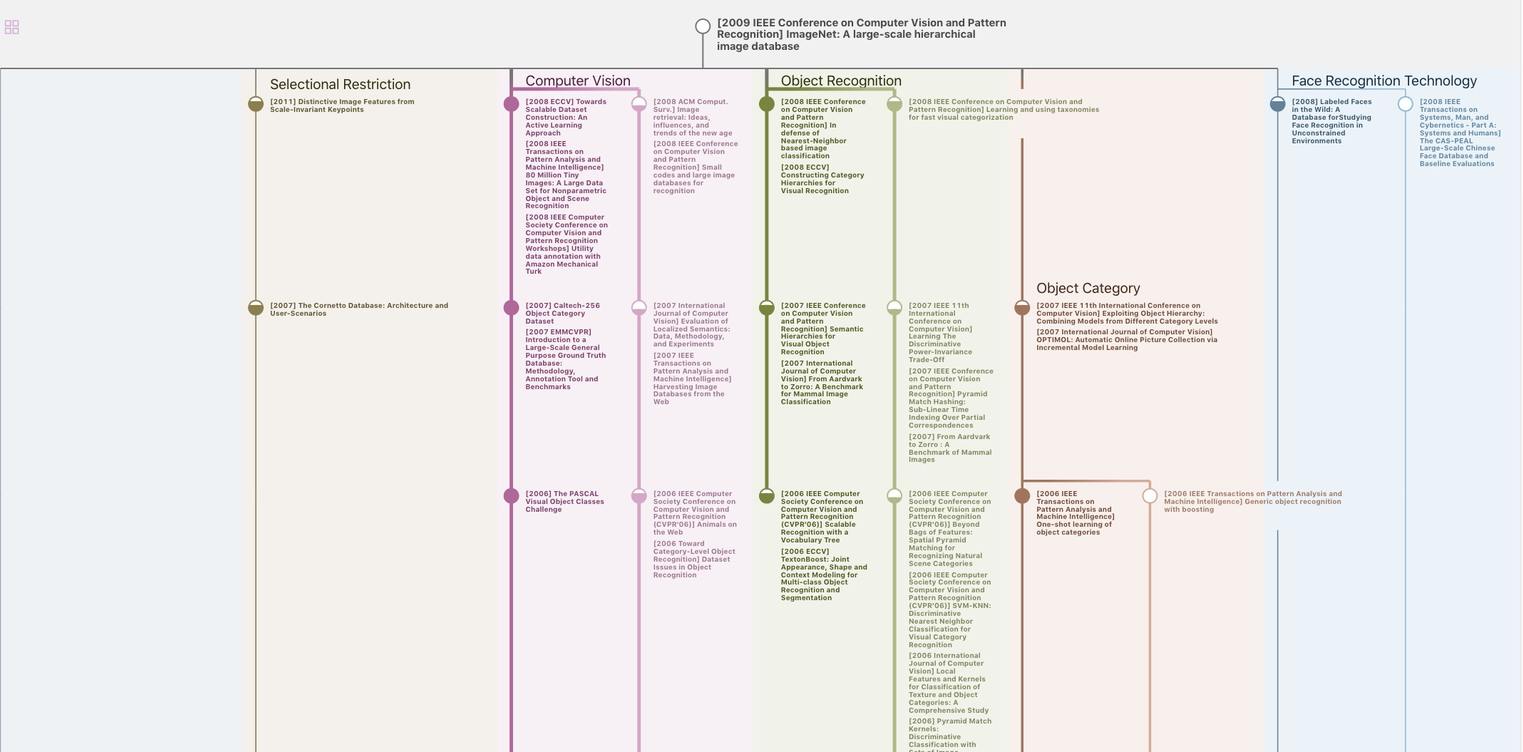
生成溯源树,研究论文发展脉络
Chat Paper
正在生成论文摘要