Joint Spinal Centerline Extraction and Curvature Estimation with Row-Wise Classification and Curve Graph Network
MEDICAL IMAGE COMPUTING AND COMPUTER ASSISTED INTERVENTION - MICCAI 2021, PT V(2021)
摘要
Spinal curvature estimation plays an important role in adolescent idiopathic scoliosis (AIS) evaluation and treatment. The Cobb angle is a well-established standard for spinal curvature estimation. Recent studies of Cobb angle estimation usually rely on detection of vertebra landmarks which requires complex post-processing of curvature calculation. Approaches directly regressing the Cobb angles apply entire image or centerline segmentation results as the network input, which limits exploring the specific curve structure of the spine. In this paper, we propose a deep learning-based approach to simultaneously estimate spine centerline and spinal curvature with shared convolutional backbone. The spine centerline extraction is formulated as a row-wise classification task. To directly regress Cobb angles, we adopt curve graph convolution to exploit curve structure of the spine centerline. In addition, given a spine centerline, a Curve Feature Pooling (CFP) module is designed to aggregate features used as the input of Curve Graph Network (CGN) to regress the Cobb angles. We evaluate our method on the accurate automated spinal curvature estimation (AASCE) challenge 2019, and the proposed approach achieves promising results on both spine centerline extraction and Cobb angles estimation tasks.
更多查看译文
关键词
Curve graph network, Curve feature pooling, Row-wise classification
AI 理解论文
溯源树
样例
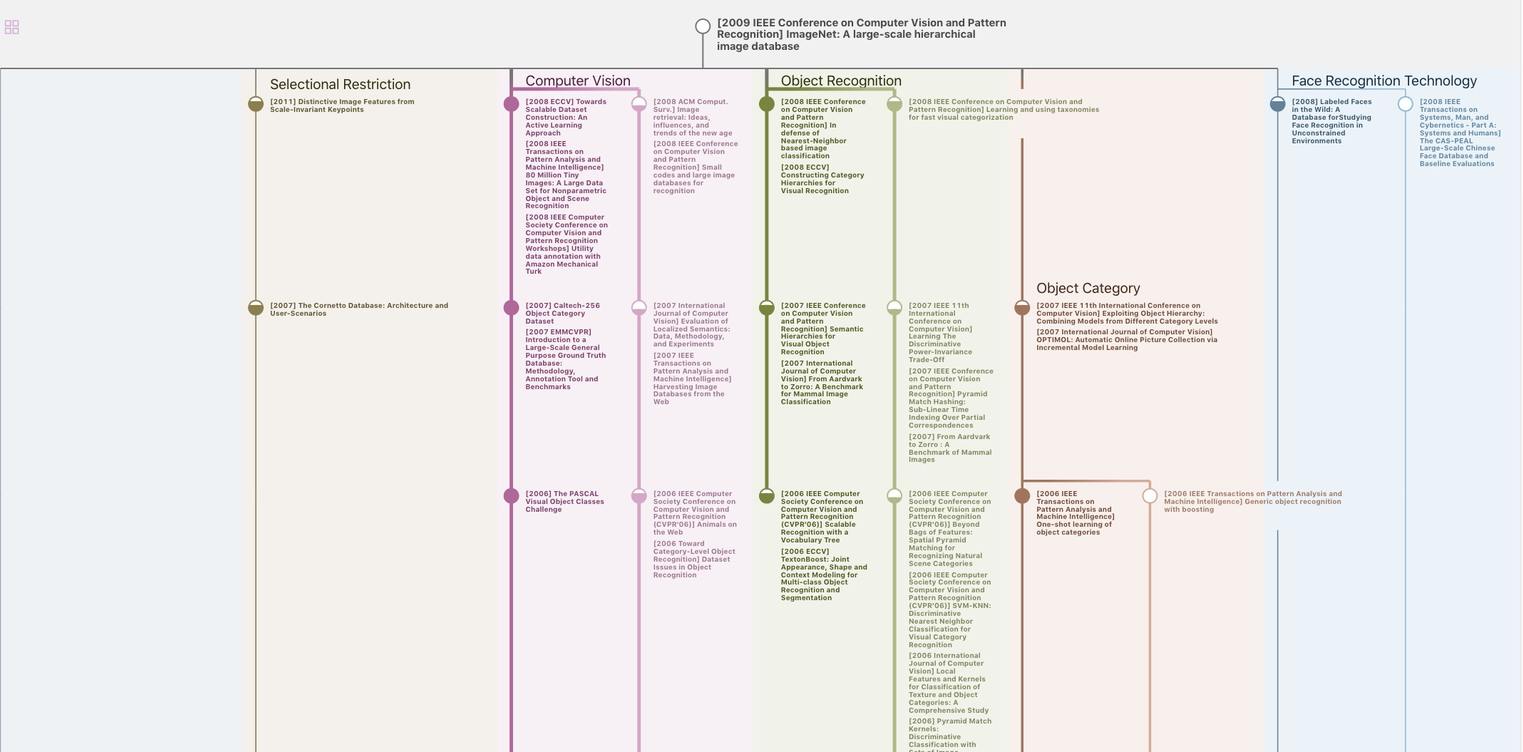
生成溯源树,研究论文发展脉络
Chat Paper
正在生成论文摘要