An Empirical Approach for Human Locomotion and Transportation Recognition from Radio Data
UBICOMP/ISWC '21 ADJUNCT PROCEEDINGS OF THE 2021 ACM INTERNATIONAL JOINT CONFERENCE ON PERVASIVE AND UBIQUITOUS COMPUTING AND PROCEEDINGS OF THE 2021 ACM INTERNATIONAL SYMPOSIUM ON WEARABLE COMPUTERS(2021)
摘要
Sussex-Huawei Locomotion-Transportation (SHL) recognition challenge, the fourth edition of the challenge provides an opportunity to recognize 8 modes of locomotion and transportation (activities) from radio data, including GPS reception, GPS location, WiFi reception, and GSM cell tower scans in a user-independent manner. Though the dataset has provided good location data, the main challenge has been how to use the variable number of data from satellite, WiFi, and cell tower information. We, team “Guerrilla”, have used the location data to extract different features like distance, velocity, acceleration, and hand-picked certain information from the satellite, WiFi, and cell tower data. Various features have been extracted from the frequency and time domain and diverse windowing techniques have been implemented. Using 1350 features, different models like Extra Trees Classifier, Random Forest Classifier, XGBoost Classifier, etc. have been trained among which the XGBoost Classifier model has performed the best. And finally, the top 250 features have been selected for the XGBoost Classifier to build an efficient model that has given accuracy and f1 score of 79.11% and 79.39% respectively on the validation dataset.
更多查看译文
关键词
Location Estimation,Location-Based Data,Indoor Localization,Activity Recognition,GPS Data
AI 理解论文
溯源树
样例
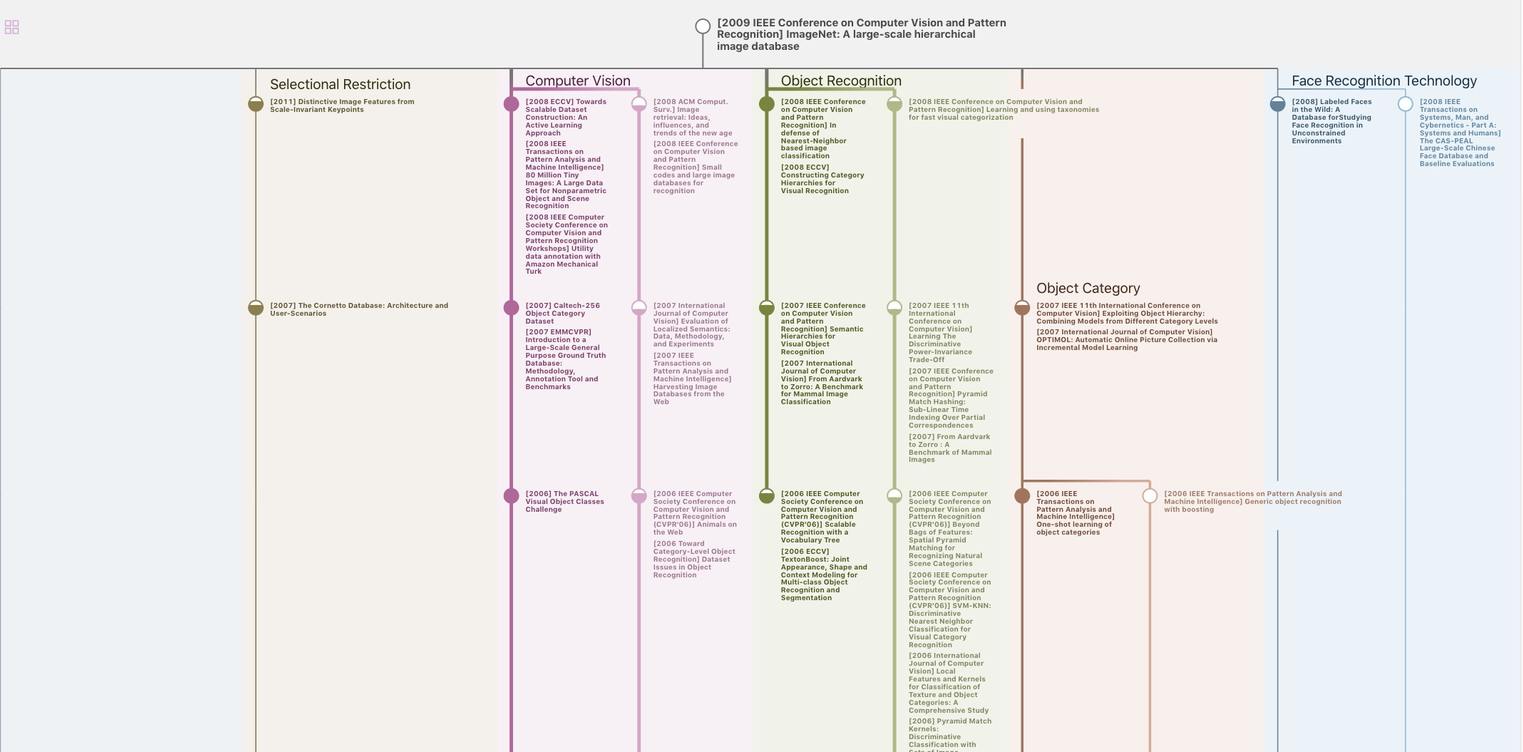
生成溯源树,研究论文发展脉络
Chat Paper
正在生成论文摘要