Multimodal Prediction of Alzheimer's Disease Severity Level Based on Resting-State EEG and Structural MRI
Frontiers in human neuroscience(2021)
摘要
While several biomarkers have been developed for the detection of Alzheimer's disease (AD), not many are available for the prediction of disease severity, particularly for patients in the mild stages of AD. In this paper, we explore the multimodal prediction of Mini-Mental State Examination (MMSE) scores using resting-state electroencephalography (EEG) and structural magnetic resonance imaging (MRI) scans. Analyses were carried out on a dataset comprised of EEG and MRI data collected from 89 patients diagnosed with minimal-mild AD. Three feature selection algorithms were assessed alongside four machine learning algorithms. Results showed that while MRI features alone outperformed EEG features, when both modalities were combined, improved results were achieved. The top-selected EEG features conveyed information about amplitude modulation rate-of-change, whereas top-MRI features comprised information about cortical area and white matter volume. Overall, a root mean square error between predicted MMSE values and true MMSE scores of 1.682 was achieved with a multimodal system and a random forest regression model.
更多查看译文
关键词
Alzheimer's disease,electroencephalography,magnetic resonance imaging,AD severity,multimodal prediction
AI 理解论文
溯源树
样例
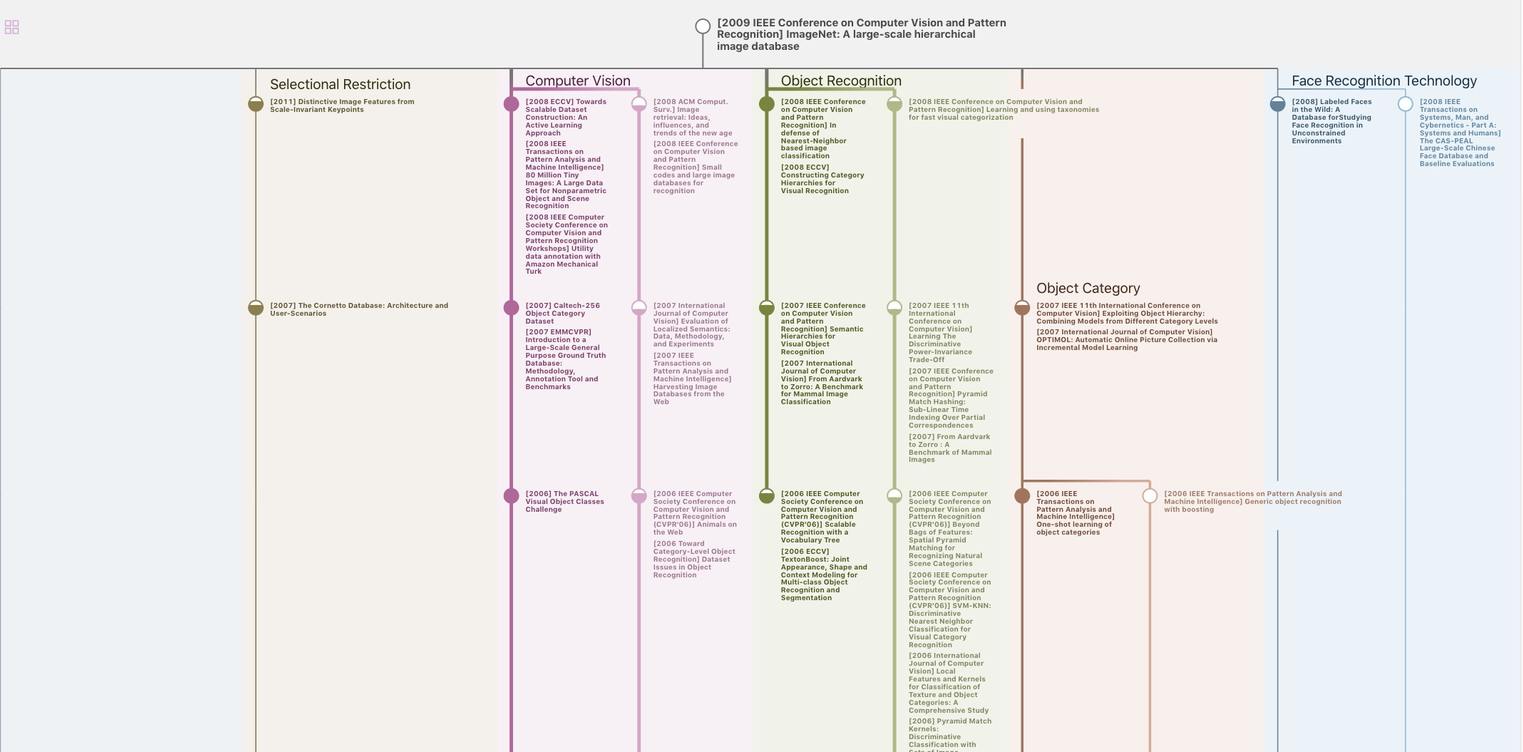
生成溯源树,研究论文发展脉络
Chat Paper
正在生成论文摘要