Comparing Text Representations - A Theory-Driven Approach.
EMNLP(2021)
摘要
Much of the progress in contemporary NLP has come from learning representations, such as masked language model (MLM) contextual embeddings, that turn challenging problems into simple classification tasks. But how do we quantify and explain this effect? We adapt general tools from computational learning theory to fit the specific characteristics of text datasets and present a method to evaluate the compatibility between representations and tasks. Even though many tasks can be easily solved with simple bag-of-words (BOW) representations, BOW does poorly on hard natural language inference tasks. For one such task we find that BOW cannot distinguish between real and randomized labelings, while pre-trained MLM representations show 72x greater distinction between real and random labelings than BOW. This method provides a calibrated, quantitative measure of the difficulty of a classification-based NLP task, enabling comparisons between representations without requiring empirical evaluations that may be sensitive to initializations and hyperparameters. The method provides a fresh perspective on the patterns in a dataset and the alignment of those patterns with specific labels.
更多查看译文
关键词
text representations,theory-driven
AI 理解论文
溯源树
样例
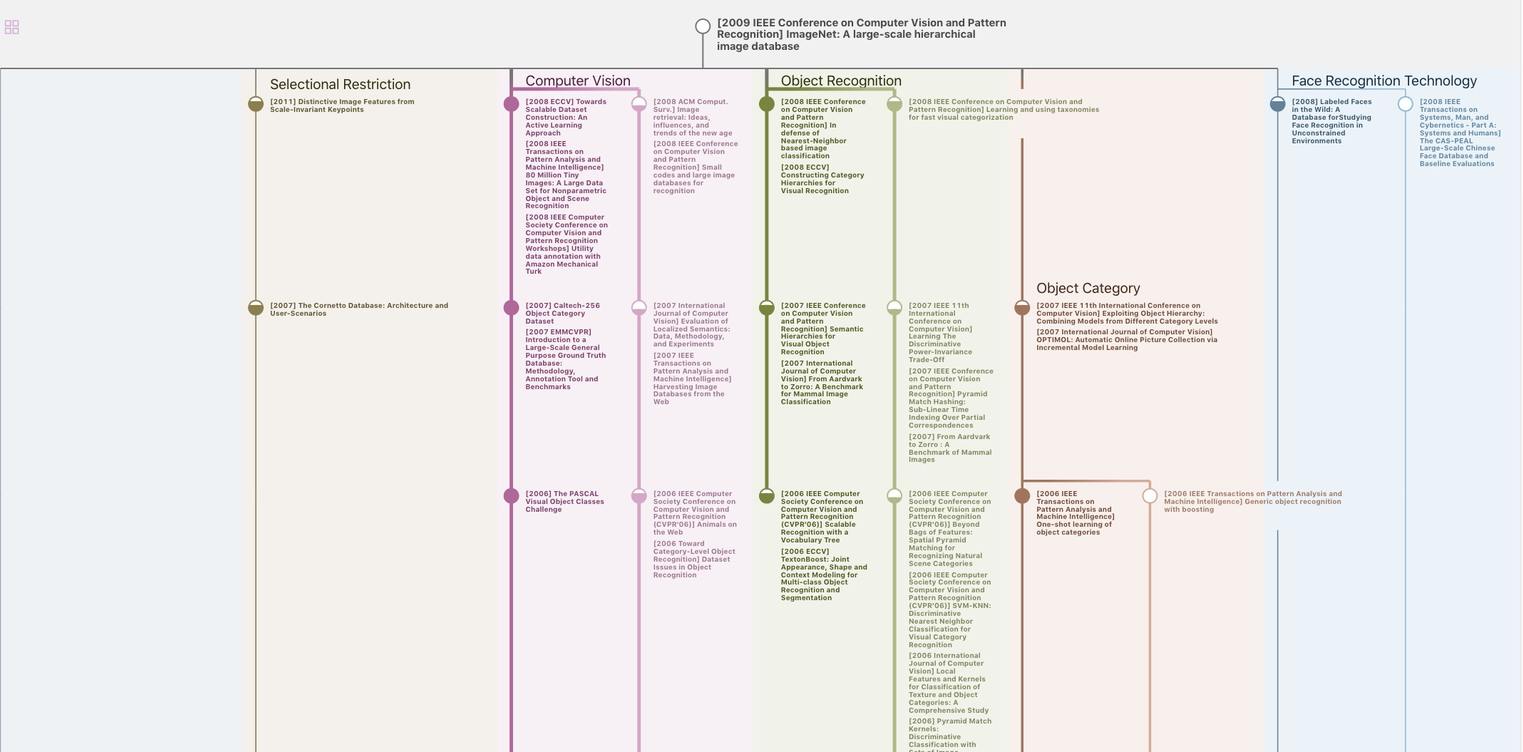
生成溯源树,研究论文发展脉络
Chat Paper
正在生成论文摘要