Utilizing Shannon's Entropy to Create Privacy Aware Architectures
arXiv (Cornell University)(2021)
摘要
Privacy is an individual choice to determine which personal details can be collected, used and shared. Individual consent and transparency are the core tenets for earning customers trust and this motivates the organizations to adopt privacy enhancing practices while creating the systems. The goal of a privacy-aware design is to protect information in a way that does not increase an adversary's existing knowledge about an individual beyond what is permissible. This becomes critical when these data elements can be linked with the wealth of auxiliary information available outside the system to identify an individual. Privacy regulations around the world provide directives to protect individual privacy but are generally complex and vague, making their translation into actionable and technical privacy-friendly architectures challenging. In this paper, we utilize Shannon's Entropy to create an objective metric that can help simplify the state-of-the-art Privacy Design Strategies proposed in the literature and aid our key technical design decisions to create privacy aware architectures.
更多查看译文
AI 理解论文
溯源树
样例
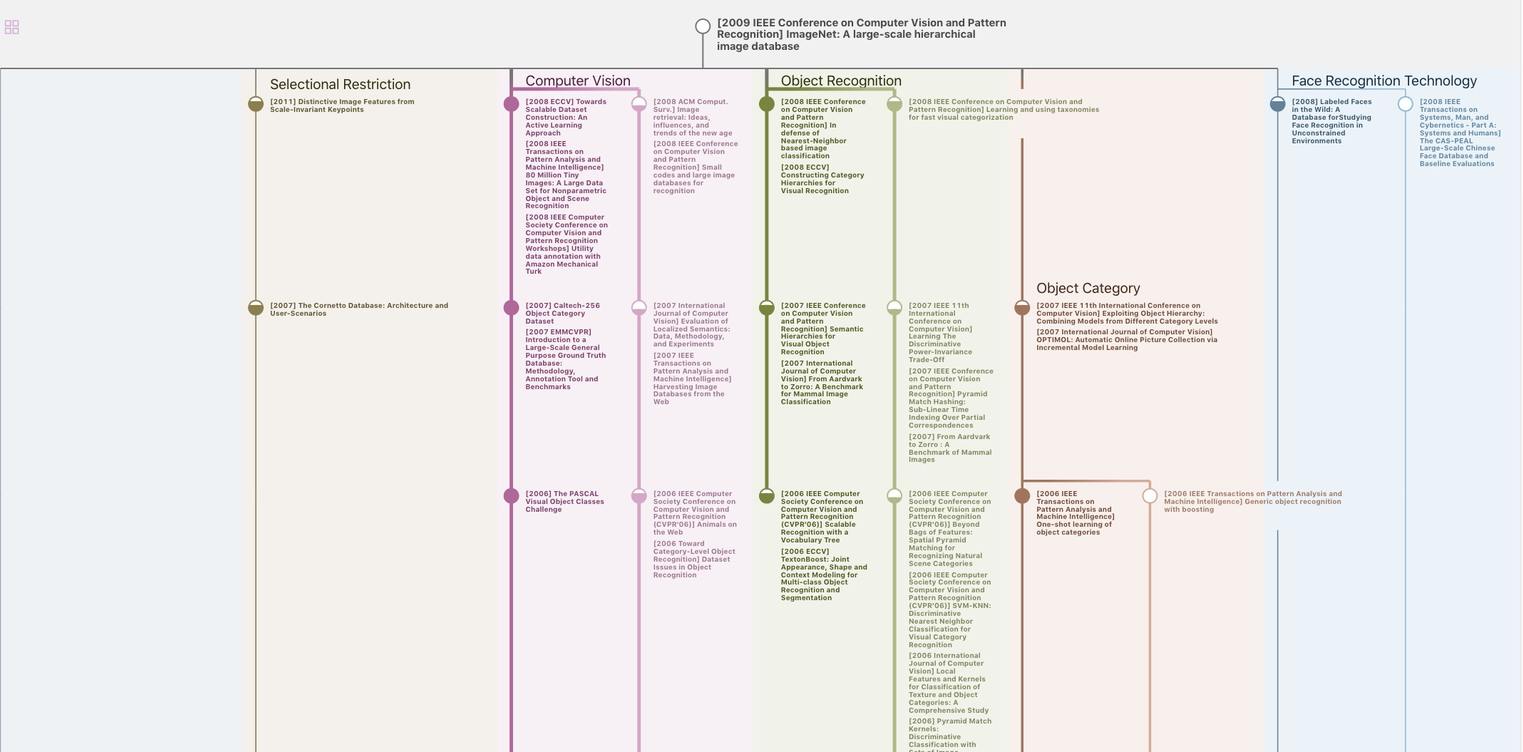
生成溯源树,研究论文发展脉络
Chat Paper
正在生成论文摘要