CoMSum and SIBERT : A Dataset and Neural Model for Query-Based Multi-document Summarization
DOCUMENT ANALYSIS AND RECOGNITION - ICDAR 2021, PT II(2021)
摘要
Document summarization compress source document(s) into succinct and information-preserving text. A variant of this is query-based multi-document summarization (q mds) that targets summaries to providing specific informational needs, contextualized to the query. However, the progress in this is hindered by limited availability to large-scale datasets. In this work, we make two contributions. First, we propose an approach for automatically generated dataset for both extractive and abstractive summaries and release a version publicly. Second, we design a neural model SIBERT for extractive summarization that exploits the hierarchical nature of the input. It also infuses queries to extract query-specific summaries. We evaluate this model on CoMSum dataset showing significant improvement in performance. This should provide a baseline and enable using CoMSum for future research on q mds.
更多查看译文
关键词
Extractive summarization,Abstractive summarization,Neural models,Transformers,Summarization dataset
AI 理解论文
溯源树
样例
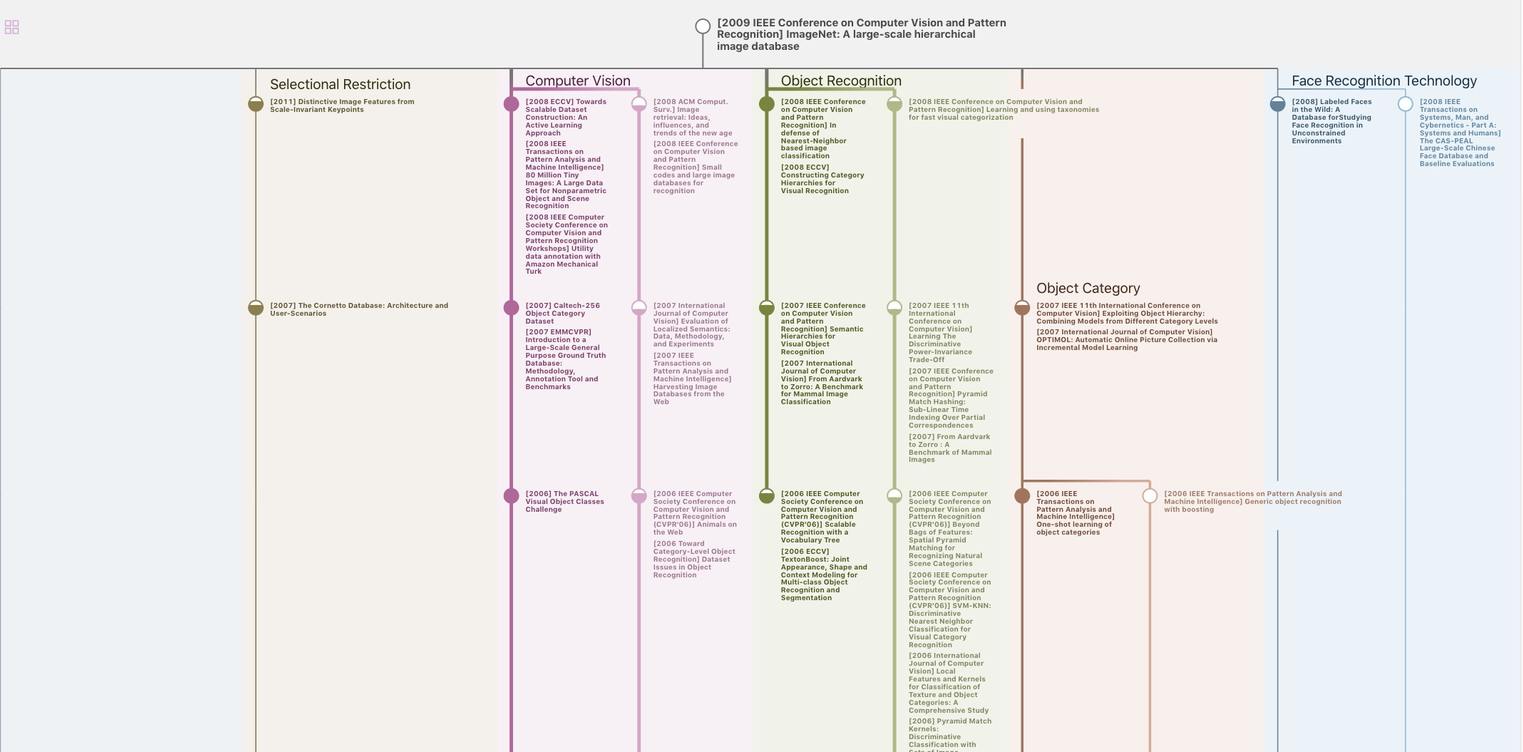
生成溯源树,研究论文发展脉络
Chat Paper
正在生成论文摘要