Handwritten Chess Scoresheet Recognition Using a Convolutional BiLSTM Network.
dblp
摘要
Chess players while playing in Over-the-Board (OTB) events use scoresheets to record their moves by hand, and later the event organizers digitize these sheets for an official record. This paper presents a framework for decoding these handwritten scoresheets automatically using a convolutional BiLSTM neural network designed and trained specifically to handle chess moves. Our proposed network is pretrained with the IAM handwriting dataset [1] and later fine-tuned with our own Handwritten Chess Scoresheet (HCS) dataset [2,3]. We also developed two basic post-processing schemes to improve accuracy by cross-checking moves between the two scoresheets collected from the players with white and black pieces. The autonomous post-processing involves no human input and achieves a Move Recognition Accuracy (MRA) of 90.1% on our test set. A second semi-autonomous algorithm involves requesting user input on certain unsettling cases. On our testing, this approach requests user input on 7% of the cases and increases MRA to 97.2%. Along with this recognition framework, we are also releasing the HCS dataset which contains scoresheets collected from actual chess events, digitized using standard cellphone cameras and tagged with associated ground truths. This is the first reported work for handwritten chess move recognition and we believe this has the potential to revolutionize the scoresheet digitization process for the thousands of chess events that happen each day.
更多查看译文
关键词
Chess scoresheet recognition,Offline handwriting recognition,Convolutional BiLSTM network,Latin handwriting recognition,Handwritten chess dataset
AI 理解论文
溯源树
样例
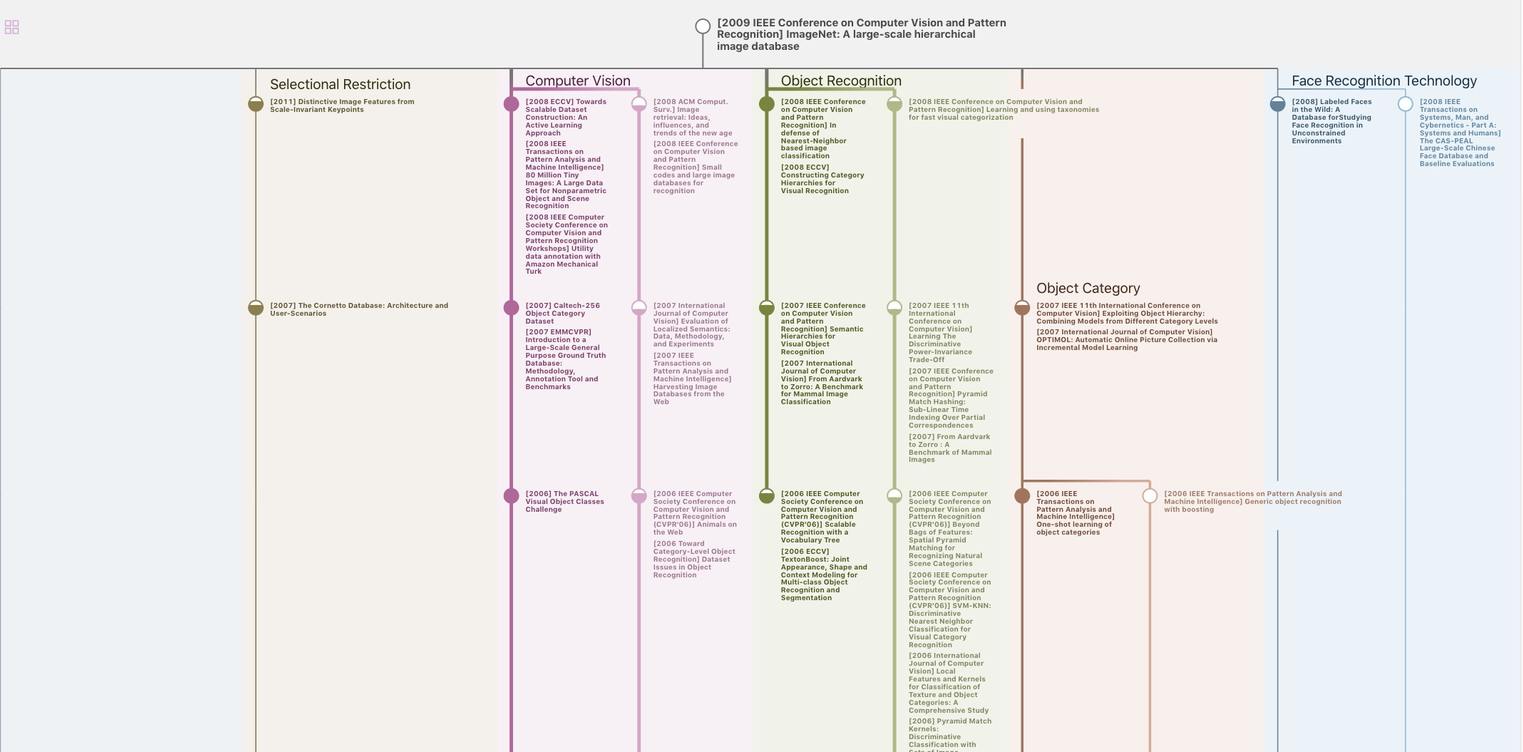
生成溯源树,研究论文发展脉络
Chat Paper
正在生成论文摘要