Subsystem Density Functional Theory Augmented by a Delta Learning Approach to Achieve Kohn-Sham Accuracy
JOURNAL OF CHEMICAL THEORY AND COMPUTATION(2021)
摘要
Simulations based on electronic structure theory naturally include polarization and have no transferability problems. In particular, Kohn-Sham density functional theory (KS-DFT) has become the method of reference for ab initio molecular dynamics simulations of condensed matter systems. However, the high computational cost often poses strict limits on the affordable system size as well as on the extension of sampling (number of configurations). In this work, we propose an improvement to the subsystem density functional theory approach, known as the Kim-Gordon (KG) scheme, thus enabling the sampling of configurations for condensed molecular systems keeping the KS-DFT level accuracy at a fraction of computer time. Our scheme compensates the known KG shortcomings of the electronic kinetic energy term by adding a simple correction and can match KS-DFT accuracy in energies and forces. The computationally cheap correction is determined by means of a machine learning procedure. The proposed KG scheme is applied within a linear scaling self-consistent field formalism and is assessed by a series of molecular dynamics simulations of liquid water under different conditions. Although system-dependent, the correction is transferable between system sizes and temperatures.
更多查看译文
关键词
Density-Functional Theory,Molecular Simulations,Hybrid Density Functionals
AI 理解论文
溯源树
样例
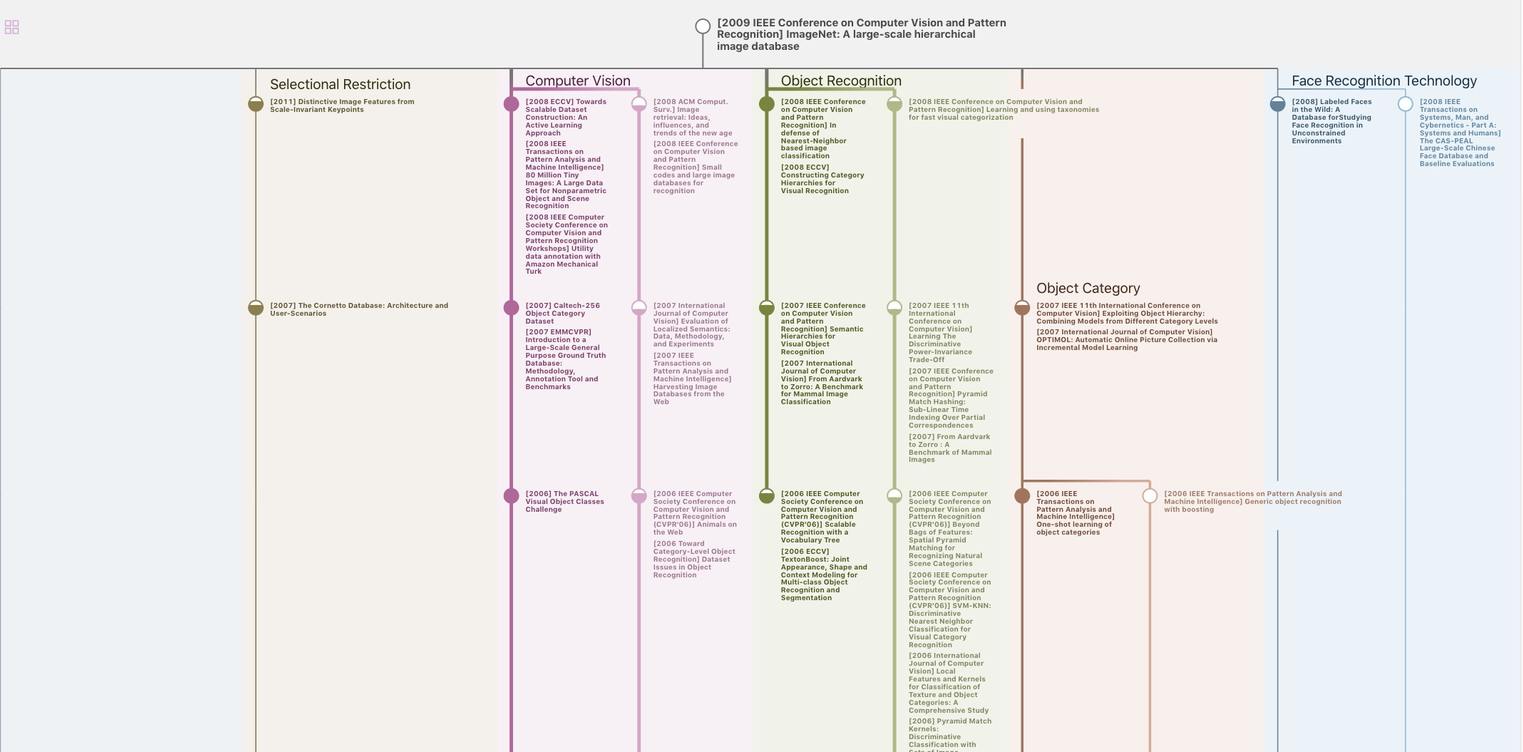
生成溯源树,研究论文发展脉络
Chat Paper
正在生成论文摘要