Vu1SPG: Vulnerability Detection Based on Slice Property Graph Representation Learning
2021 IEEE 32ND INTERNATIONAL SYMPOSIUM ON SOFTWARE RELIABILITY ENGINEERING (ISSRE 2021)(2021)
关键词
Security,Vulnerability detection,Program representation learning,Deep graph neural network,Program slicing
AI 理解论文
溯源树
样例
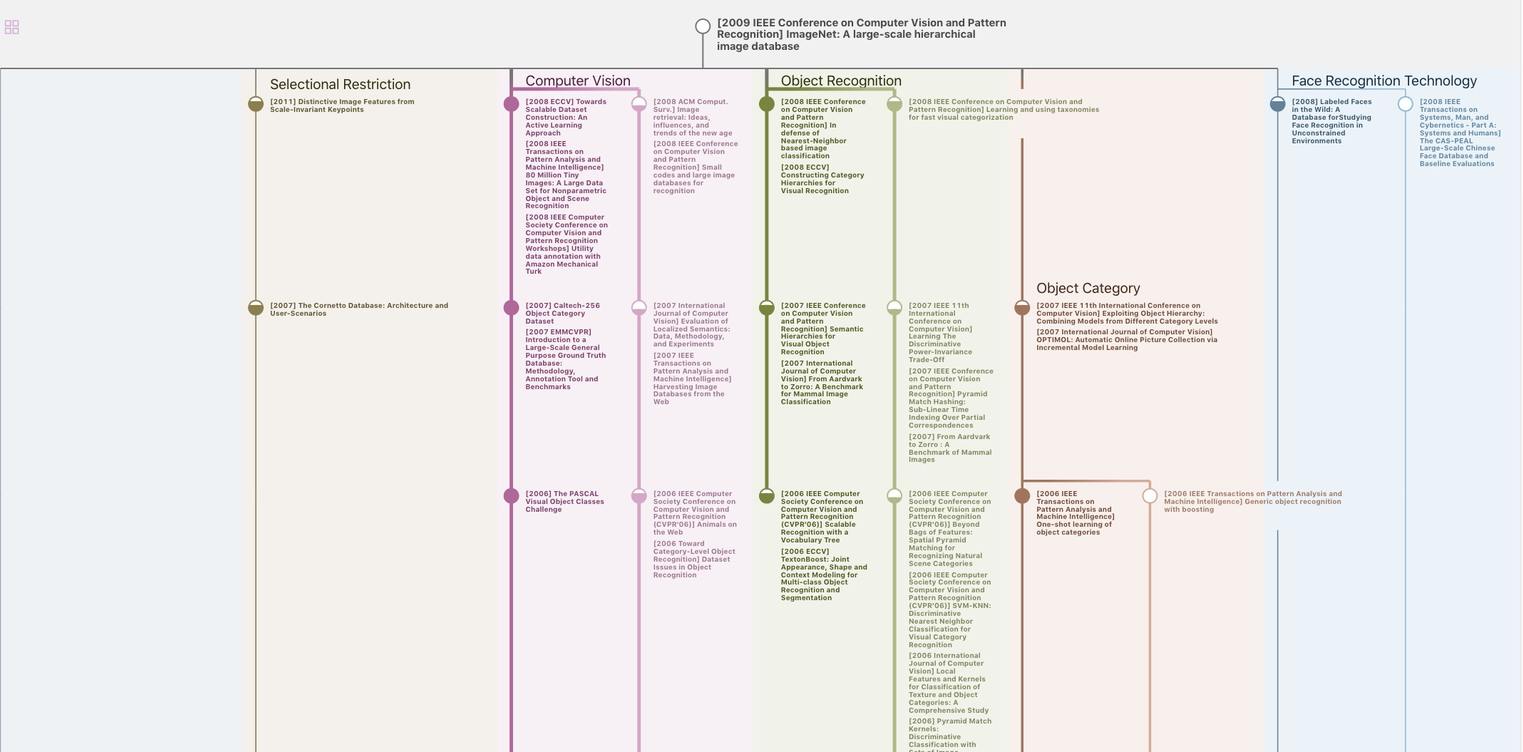
生成溯源树,研究论文发展脉络
Chat Paper
正在生成论文摘要