Learning to predict crop type from heterogeneous sparse labels using meta-learning
2021 IEEE/CVF CONFERENCE ON COMPUTER VISION AND PATTERN RECOGITION WORKSHOPS (CVPRW 2021)(2021)
摘要
There are many labelled datasets relating to land cover and crop type mapping that cover diverse geographies, agroecologies and land uses. However, these labels are often extremely sparse, particularly in low- and middle-income regions, with as few as tens of examples for certain crop types. This makes it challenging to train supervised machine learning models to detect specific crops in satellite observations of these regions. We investigate the utility of model-agnostic meta-learning (MAML) to learn from diverse global datasets and improve performance in data-sparse regions. We find that in a variety of countries (Togo, Kenya and Brazil) and across a variety of tasks (crop type mapping, crop vs. non-crop mapping), MAML improves performance compared to pretrained and random initial weights. We also investigate the utility of MAML for different target data-size regimes. We find MAML outperforms other methods for a wide range of training set sizes and positive to negative label ratios, indicating its general suitability for land use and crop type mapping.
更多查看译文
关键词
heterogeneous sparse labels,labelled datasets,low-and-middle-income regions,supervised machine learning models,model-agnostic meta-learning,MAML,diverse global datasets,data-sparse regions,diverse geographies,agroecologies,crop type mapping prediction
AI 理解论文
溯源树
样例
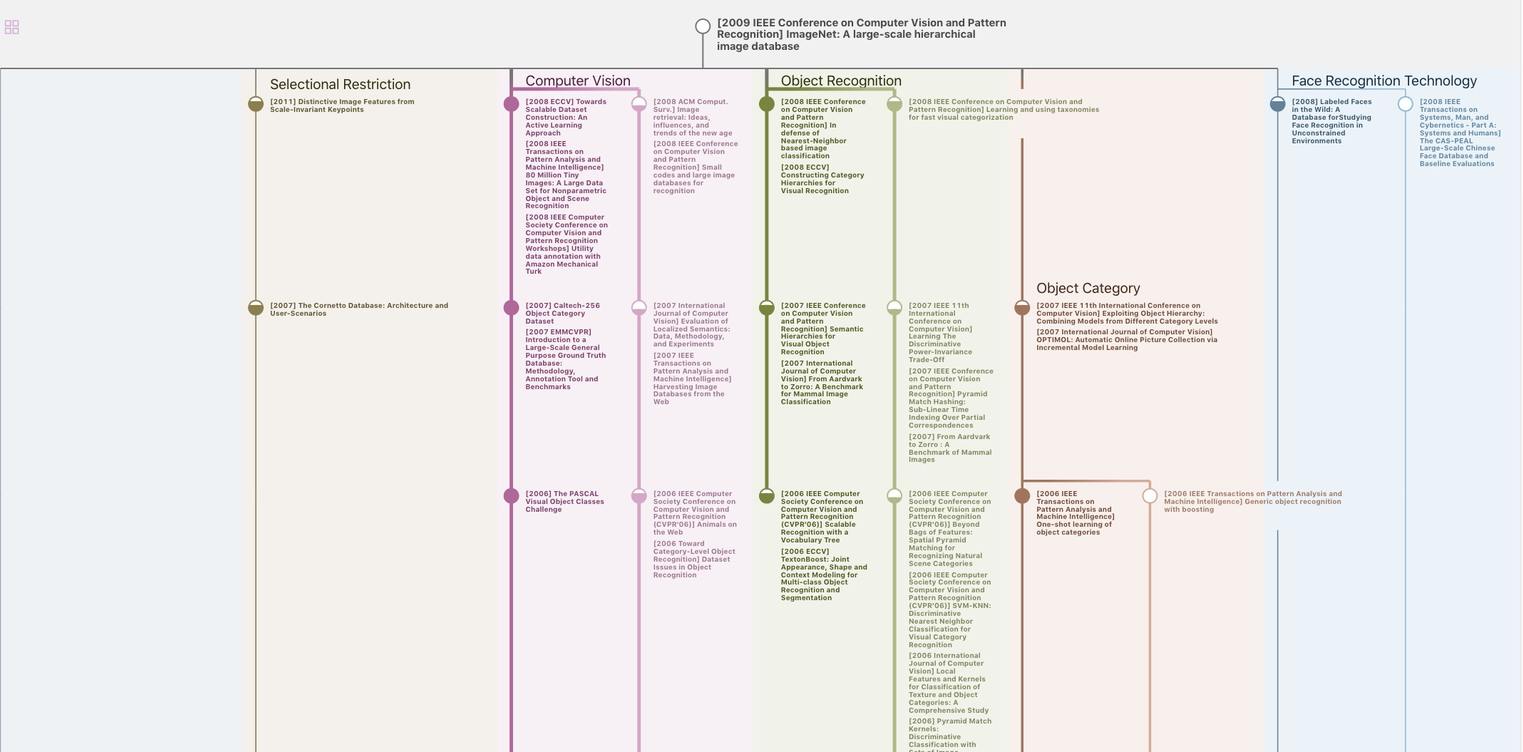
生成溯源树,研究论文发展脉络
Chat Paper
正在生成论文摘要