QUEACO: Borrowing Treasures from Weakly-labeled Behavior Data for Query Attribute Value Extraction
International Conference on Information and Knowledge Management(2021)
摘要
ABSTRACTWe study the problem of query attribute value extraction, which aims to identify named entities from user queries as diverse surface form attribute values and afterward transform them into formally canonical forms. Such a problem consists of two phases: named entity recognition (NER) and attribute value normalization (AVN). However, existing works only focus on the NER phase but neglect equally important AVN. To bridge this gap, this paper proposes a unified query attribute value extraction system in e-commerce search named QUEACO, which involves both two phases. Moreover, by leveraging large-scale weakly-labeled behavior data, we further improve the extraction performance with less supervision cost. Specifically, for the NER phase, QUEACO adopts a novel teacher-student network, where a teacher network that is trained on the strongly-labeled data generates pseudo-labels to refine the weakly-labeled data for training a student network. Meanwhile, the teacher network can be dynamically adapted by the feedback of the student's performance on strongly-labeled data to maximally denoise the noisy supervisions from the weak labels. For the AVN phase, we also leverage the weakly-labeled query-to-attribute behavior data to normalize surface form attribute values from queries into canonical forms from products. Extensive experiments on a real-world large-scale E-commerce dataset demonstrate the effectiveness of QUEACO.
更多查看译文
AI 理解论文
溯源树
样例
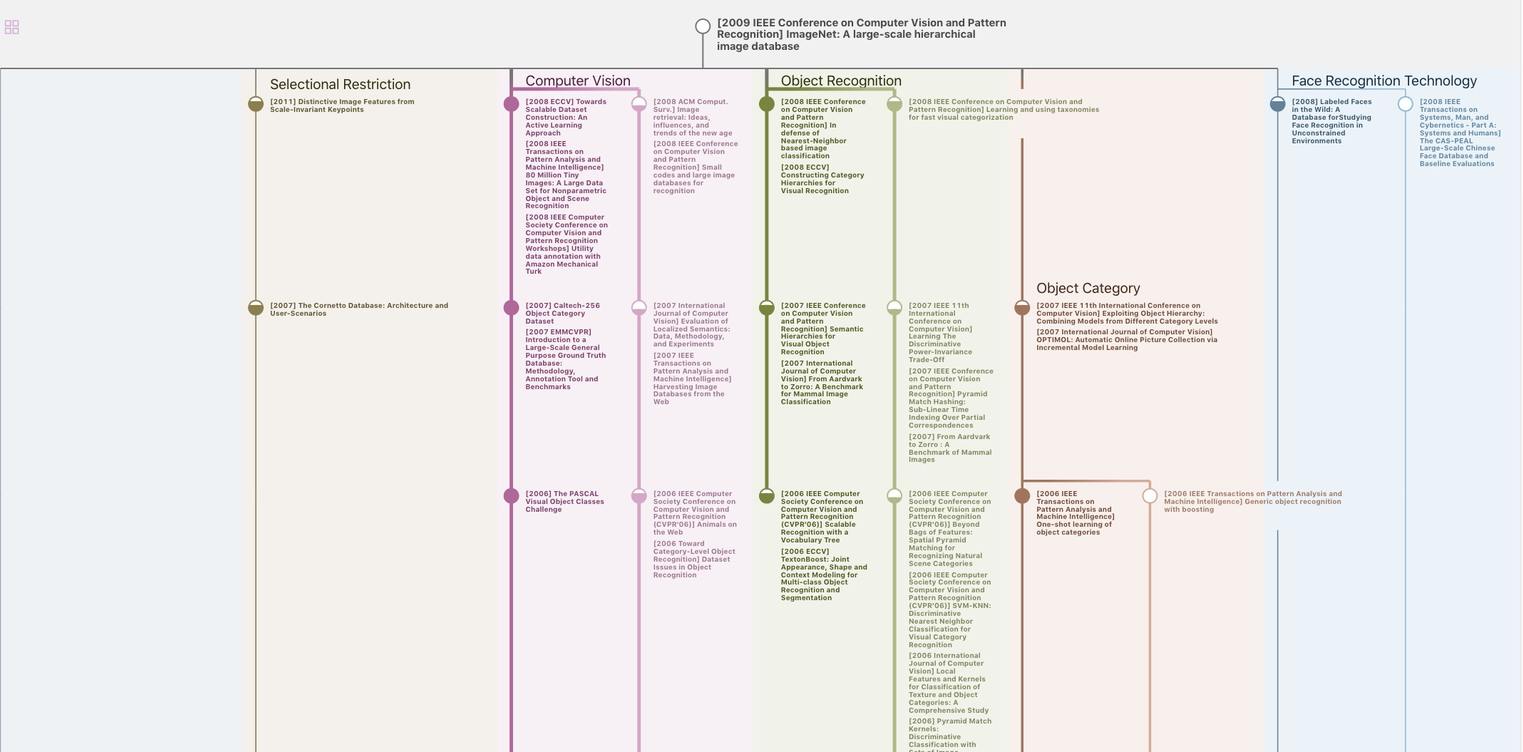
生成溯源树,研究论文发展脉络
Chat Paper
正在生成论文摘要