Quantifying wintertime O 3 and NO x formation with relevance vector machines.
Atmospheric environment (Oxford, England : 1994)(2021)
摘要
This paper uses a machine learning model called a relevance vector machine (RVM) to quantify ozone (O) and nitrogen oxides (NO) formation under wintertime conditions. Field study measurements were based on previous work described by Olson et al. (2019), where continuous measurements were reported from a wintertime field study in Utah. RVMs were formulated using either O or nitrogen dioxide (NO) as the output variable. Values of the correlation coefficient (r) between predicted and measured concentrations were 0.944 for O and 0.931 for NO. RVMs are constructed from the observed measurements and result in sparse model formulations, meaning that only a subset of the data is used to approximate the entire dataset. For this study, the RVM with O as the output variable used only 20% of the measurement data while the RVM with NO used 16%. RVMs were then used as a predictive model to assess the importance of individual precursors. Using O as the output variable, increases in three species resulted in increased O concentrations: hydrogen peroxide (HO), dinitrogen pentoxide (NO), and molecular chlorine (Cl). For the two termination products measured during the study, nitric acid (HNO) and formic acid (CHO), no change in O concentration was observed. Using NO as the output variable, only increases in NO resulted in increased NO concentrations.
更多查看译文
关键词
dinitrogen pentoxide,hydrogen peroxide,machine learning,nitrous acid,nitryl chloride,support vector machine
AI 理解论文
溯源树
样例
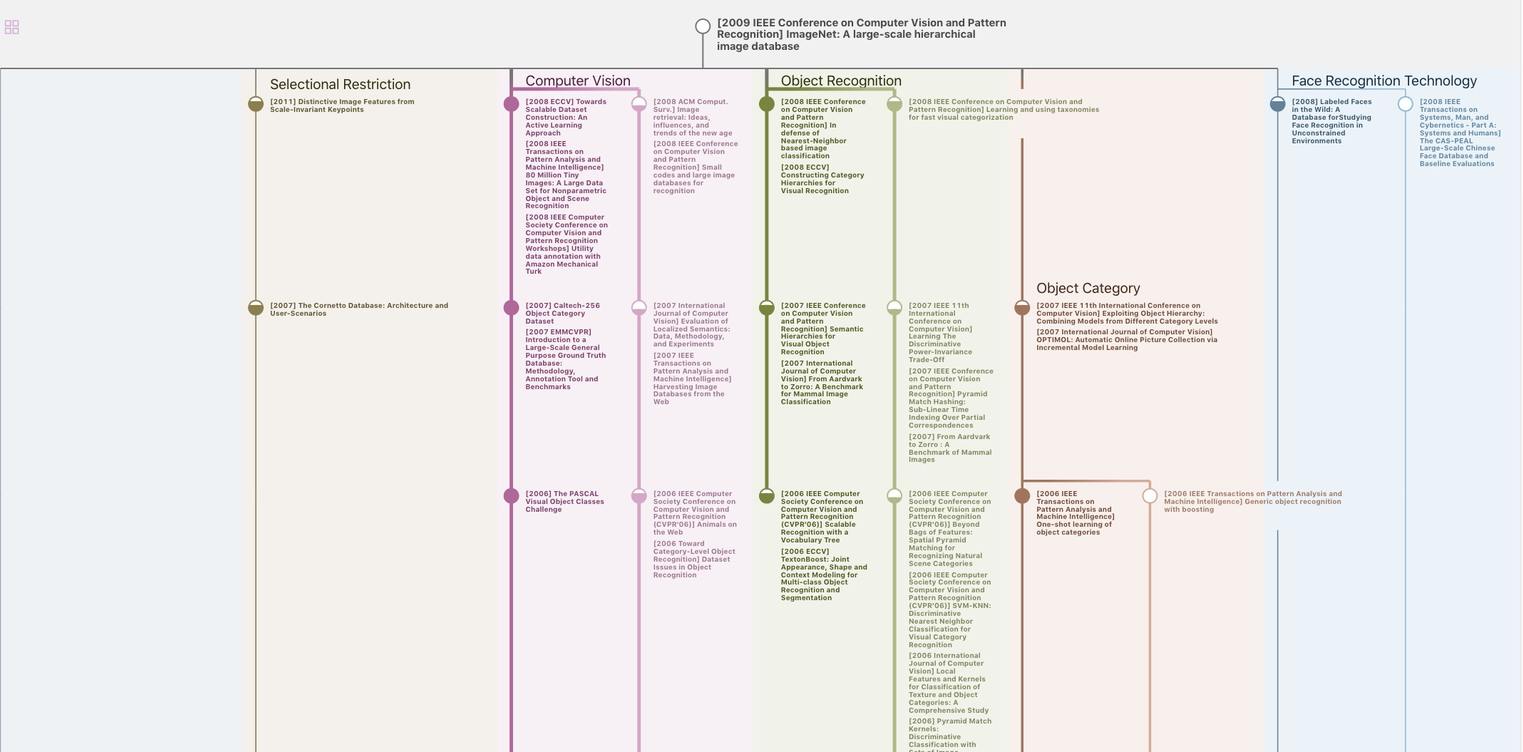
生成溯源树,研究论文发展脉络
Chat Paper
正在生成论文摘要