Managing ML pipelines: feature stores and the coming wave of embedding ecosystems
Hosted Content(2021)
摘要
AbstractThe industrial machine learning pipeline requires iterating on model features, training and deploying models, and monitoring deployed models at scale. Feature stores were developed to manage and standardize the engineer's workflow in this end-to-end pipeline, focusing on traditional tabular feature data. In recent years, however, model development has shifted towards using self-supervised pretrained embeddings as model features. Managing these embeddings and the downstream systems that use them introduces new challenges with respect to managing embedding training data, measuring embedding quality, and monitoring downstream models that use embeddings. These challenges are largely unaddressed in standard feature stores. Our goal in this tutorial is to introduce the feature store system and discuss the challenges and current solutions to managing these new embedding-centric pipelines.
更多查看译文
关键词
ml pipelines,feature stores,ecosystems
AI 理解论文
溯源树
样例
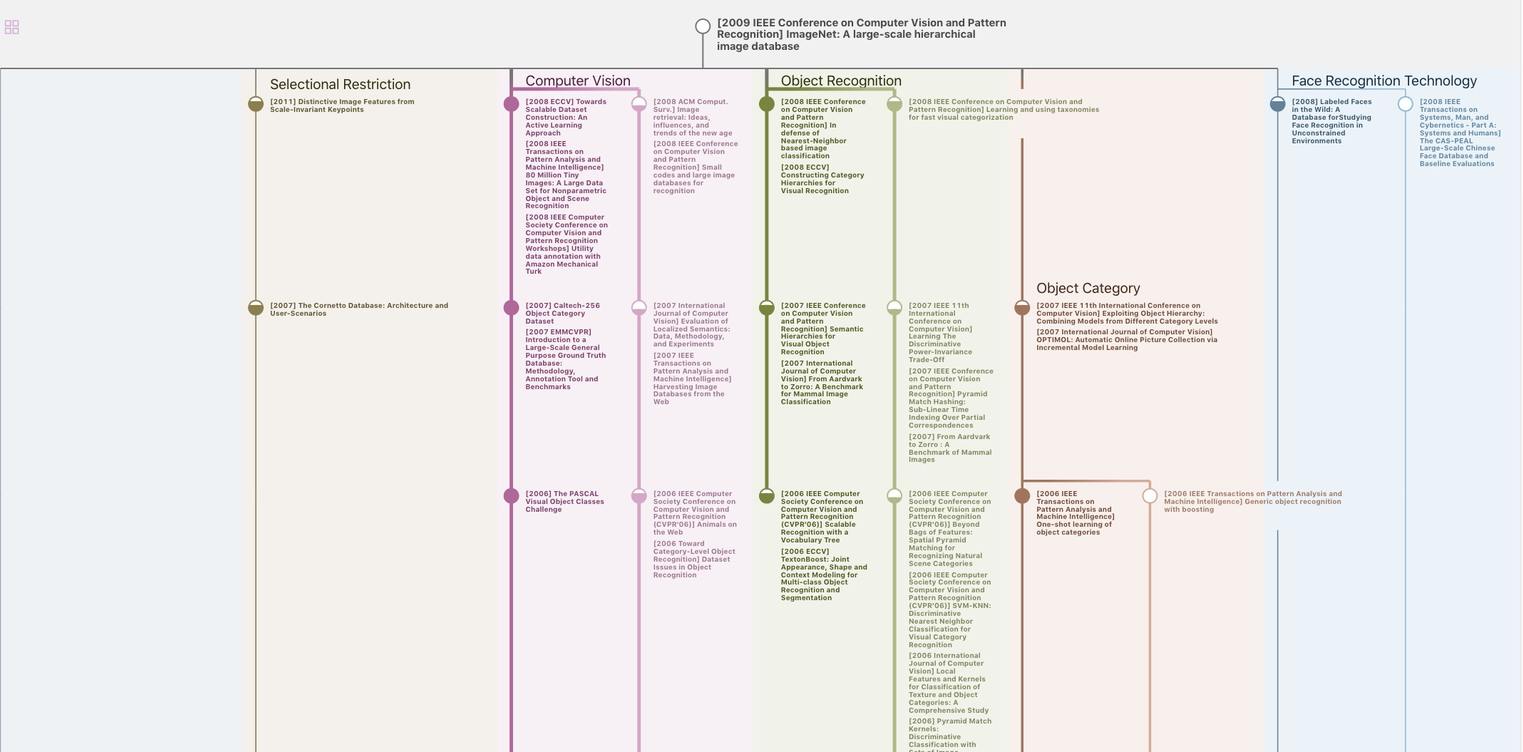
生成溯源树,研究论文发展脉络
Chat Paper
正在生成论文摘要