Improved-Mask R-CNN: Towards an Accurate Generic MSK MRI Instance Segmentation Platform (data from the Osteoarthritis Initiative).
Computerized medical imaging and graphics(2022)
摘要
INTRODUCTION:Objective assessment of osteoarthritis (OA) Magnetic Resonance Imaging (MRI) scans can address the limitations of the current OA assessment approaches. Detecting and extracting bone, cartilage, and joint fluid is a necessary component for the objective assessment of OA, which helps to quantify tissue characteristics such as volume and thickness. Many algorithms, based on Artificial Intelligence (AI), have been proposed over recent years for segmenting bone and soft tissues. Most of these segmentation methods suffer from the class imbalance problem, can't differentiate between the same anatomic structure, or do not support segmenting different rang of tissue sizes. Mask R-CNN is an instance segmentation framework, meaning it segments and distinct each object of interest like different anatomical structures (e.g. bone and cartilage) using a single model. In this study, the Mask R-CNN architecture was deployed to address the need for a segmentation method that is applicable to use for different tissue scales, pathologies, and MRI sequences associated with OA, without having a problem with imbalanced classes. In addition, we modified the Mask R-CNN to improve segmentation accuracy around instance edges.METHODS:A total of 500 adult knee MRI scans from the publicly available Osteoarthritis Initiative (OAI), and 97 hip MRI scans from adults with symptomatic hip OA, evaluated by two readers, were used for training and validating the network. Three specific modifications to Mask R-CNN yielded the improved-Mask R-CNN (iMaskRCNN): an additional ROIAligned block, an extra decoder block in the segmentation header, and connecting them using a skip connection. The results were evaluated using Hausdorff distance, dice score for bone and cartilage segmentation, and differences in detected volume, dice score, and coefficients of variation (CoV) for effusion segmentation.RESULTS:The iMaskRCNN led to improved bone and cartilage segmentation compared to Mask RCNN as indicated with the increase in dice score from 95% to 98% for the femur, 95-97% for the tibia, 71-80% for the femoral cartilage, and 81-82% for the tibial cartilage. For the effusion detection, the dice score improved with iMaskRCNN 72% versus Mask R-CNN 71%. The CoV values for effusion detection between Reader1 and Mask R-CNN (0.33), Reader1 and iMaskRCNN (0.34), Reader2 and Mask R-CNN (0.22), Reader2 and iMaskRCNN (0.29) are close to CoV between two readers (0.21), indicating a high agreement between the human readers and both Mask R-CNN and iMaskRCNN.CONCLUSION:Mask R-CNN and iMaskRCNN can reliably and simultaneously extract different scale articular tissues involved in OA, forming the foundation for automated assessment of OA. The iMaskRCNN results show that the modification improved the network performance around the edges.
更多查看译文
关键词
Deep learning,Tissue segmentation,Osteoarthritis,MRI,Bone and cartilage,Effusion
AI 理解论文
溯源树
样例
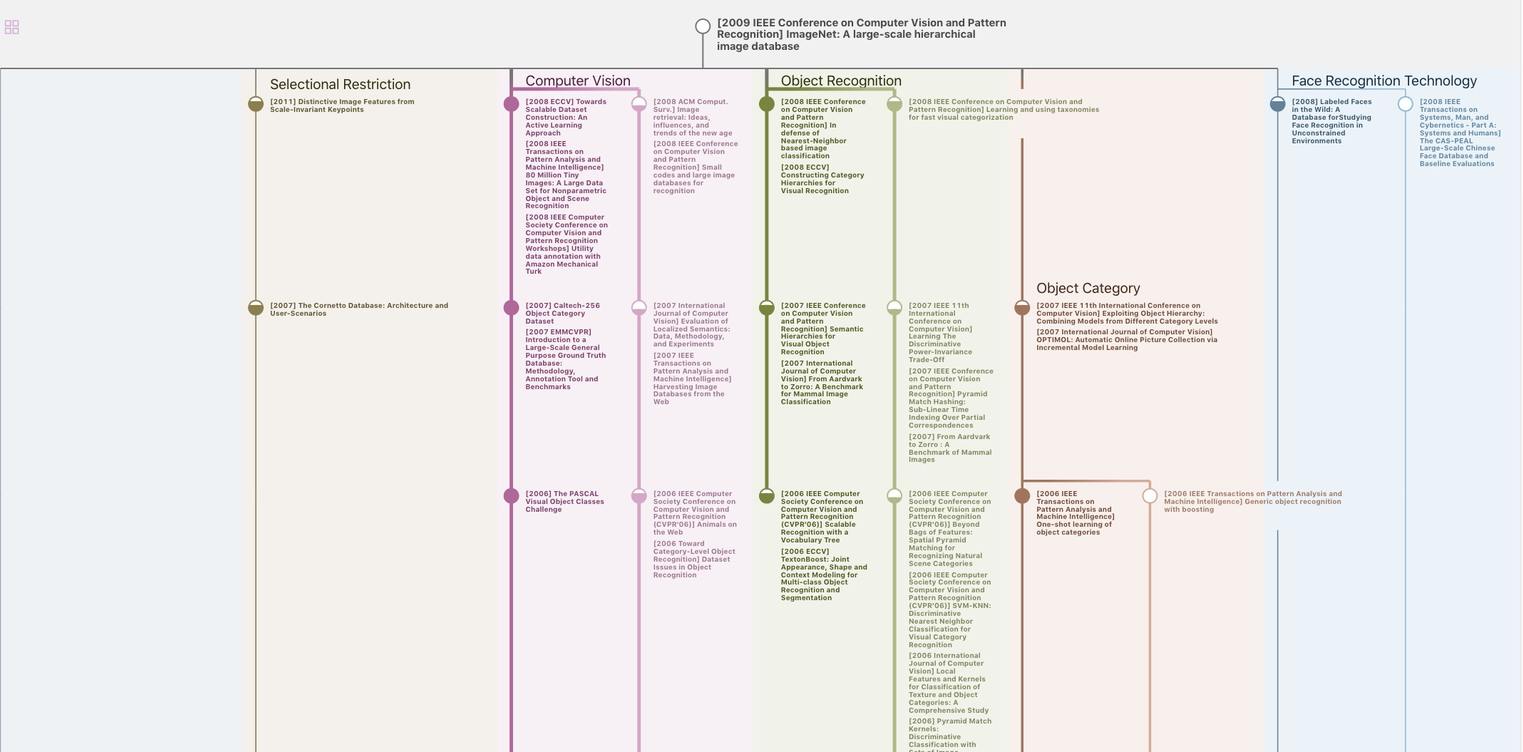
生成溯源树,研究论文发展脉络
Chat Paper
正在生成论文摘要