Optimizing DNNs with Partially Equivalent Transformations and Automated Corrections
Operating Systems Design and Implementation(2021)
Abstract
Deep neural network (DNN) applications are typically represented by tensor programs. To boost the performance of DNN computations, existing works adopt fully equivalent transformations for tensor program optimization by guaranteeing the equivalence on each element of tensors. However, as there are thousands of elements in a tensor, such optimization misses the opportunities that allow the in-equivalence of minority elements. In this work, we propose Pet , the first work that introduces partially equivalent transformations to optimize tensor programs. To maintain the functional equivalence of tensor programs, Pet automatically finds and corrects the in-equivalent positions by leveraging the multi-linearity of DNN computations. Pet further uses a mutation manager to improve search efficiency. Evaluation results show that Pet can achieve up to 1.98 $\times$ and 2.20 $\times$ speedups on NVIDIA Tesla A100 and V100 respectively compared with existing DNN frameworks by introducing new optimization opportunities of partially equivalent transformations.
MoreTranslated text
Key words
Tensors,Optimization,Shape,Kernel,Artificial neural networks,Generators,Task analysis,AI compiler,DNN optimization,tensor programs,partially equivalent transformation
AI Read Science
Must-Reading Tree
Example
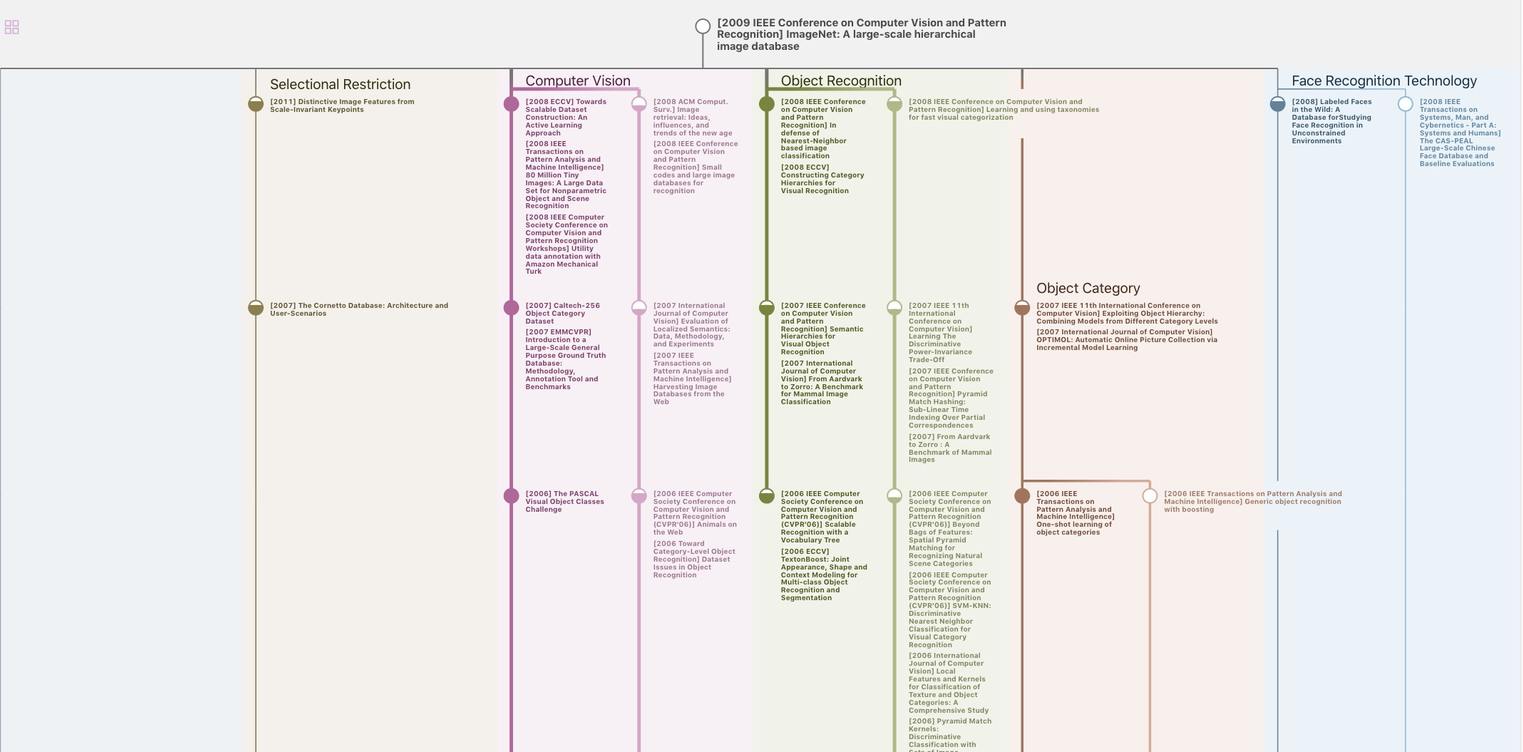
Generate MRT to find the research sequence of this paper
Chat Paper
Summary is being generated by the instructions you defined