Investigation of the impact of thermodynamic profiles of ground based lidar systems on short-range forecast skill by means of ETKF-hybrid 3DVAR data assimilation
user-5dd52aee530c701191bf1b99(2021)
摘要
<p>Ground based active remote-sensing instruments have proved its potential through its high quality observations of thermodynamic profiles. In this study, thermodynamic profiles obtained from the temperature Raman lidar (TRL) and the water-vapour differential absorption lidar (DIAL) of the University of Hohenheim (UHOH) are assimilated into the Weather Research and Forecasting model data assimilation (WRFDA) system through a new forward operator for absolute humidity and mixing ratio developed in-house.<br>Thermodynamic DA was performed either with the deterministic 3-dimensional variational (3DVAR) DA system or with the hybrid 3DVAR-Ensemble Transform Kalman Filter (ETKF) approach. We used data of the High Definition of Clouds and Precipitation for advancing Climate Prediction (HD(CP)2 project Observation Prototype Experiment (HOPE). The WRF model was configured for a central European domain at a convection permitting resolution of 2.5 km spatial grid increment and 100 levels in the vertical with fine resolution in the planetary boundary layer (PBL). The assimilation experiments were conducted in a rapid update cycle (RUC) mode with an hourly update frequency. The hybrid 3DVAR-ETKF DA system was incorporated with an adaptive inflation scheme using a set of 10 ensemble members each with the same configuration as the previous experiments for the 3DVAR.  We will present the results of three DA experiments. In the first experiment (CONV_DA), or the control run, only assimilation of the conventional observations was carried out with 3DVAR DA. The second experiment (QT_DA) was a 3DVAR DA assimilating WVMR and temperature together in addition to the conventional dataset. The third experiment (QT_HYB_DA) assimilated WVMR and temperature together in addition to the conventional dataset with Hybrid DA.<br>The WVMR RMSE with respect to the WVDIAL reduced by 41 % in 3DVAR and still reduced to 51 % in QT_HYB_DA compared to CONV_DA. Although temperature RMSE with respect to TRL increased by 5 % in QT_DA, RMSE significantly reduced to 47 % in QT_HYB_DA compared to CONV_DA. The correlation between the temperature and WVMR variables in the background error covariance matrix of the 3DVAR, which is static and not flow-dependent, limited the improvement in temperature. Flow-dependency in Hybrid DA improved the error correlations.<br>We also present results of a collaborative effort with the Raman lidar for meteorological observation (RALMO) from the MeteoSwiss and the Atmospheric Raman Temperature and Humidity Sounder (ARTHUS) using even finer model resolution. The initial results of the new study will also be presented here.</p>
更多查看译文
关键词
Forecast skill,Lidar,Data assimilation,Range (statistics),Meteorology,Environmental science
AI 理解论文
溯源树
样例
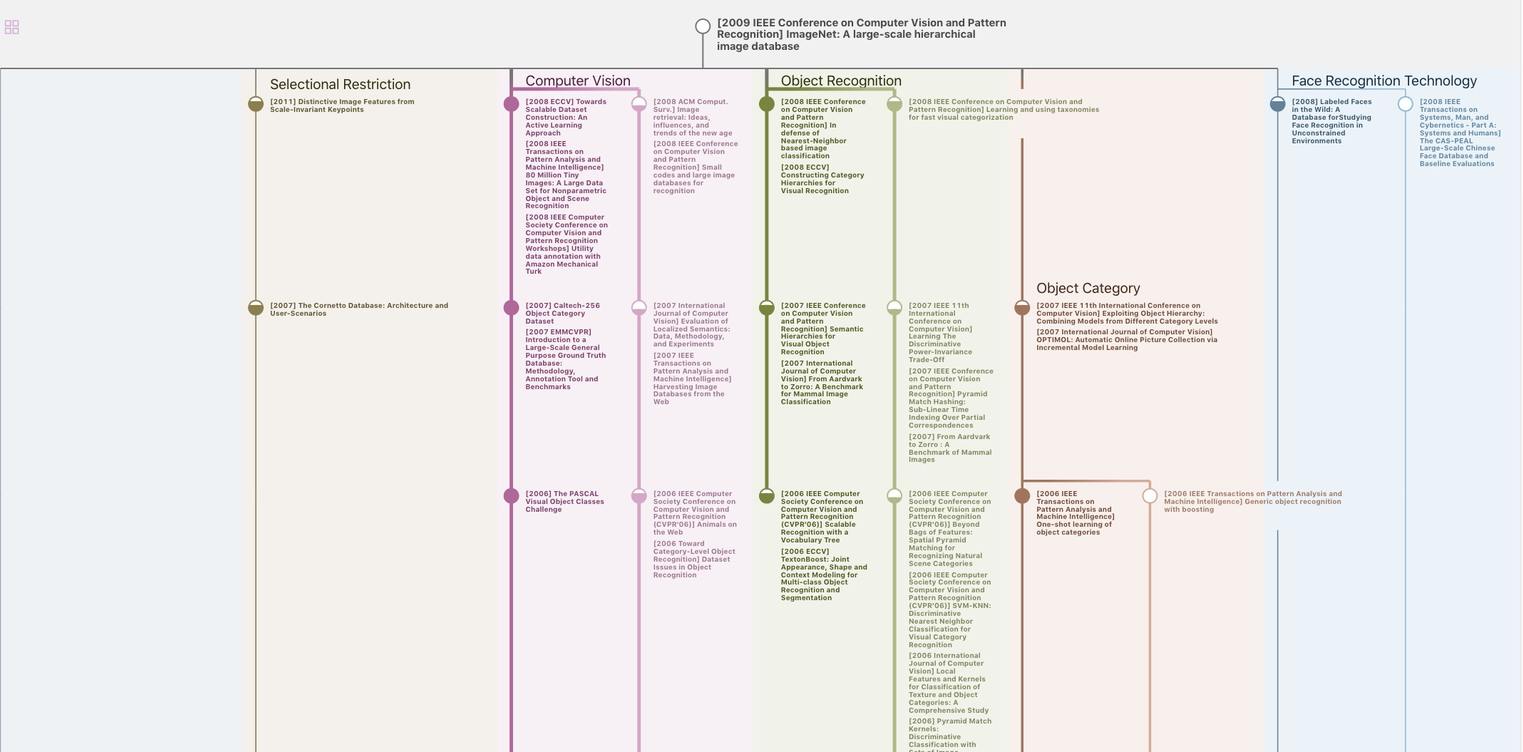
生成溯源树,研究论文发展脉络
Chat Paper
正在生成论文摘要