Improved Prediction of Protein-Protein Interaction Using a Hybrid of Functional-Link Siamese Neural Network and Gradient Boosting Machines.
Briefings in bioinformatics(2021)
摘要
In this paper, for accurate prediction of protein-protein interaction (PPI), a novel hybrid classifier is developed by combining the functional-link Siamese neural network (FSNN) with the light gradient boosting machine (LGBM) classifier. The hybrid classifier (FSNN-LGBM) uses the fusion of features derived using pseudo amino acid composition and conjoint triad descriptors. The FSNN extracts the high-level abstraction features from the raw features and LGBM performs the PPI prediction task using these abstraction features. On performing 5-fold cross-validation experiments, the proposed hybrid classifier provides average accuracies of 98.70 and 98.38%, respectively, on the intraspecies PPI data sets of Saccharomyces cerevisiae and Helicobacter pylori. Similarly, the average accuracies for the interspecies PPI data sets of the Human-Bacillus and Human-Yersinia data sets are 98.52 and 97.40%, respectively. Compared with the existing methods, the hybrid classifier achieves higher prediction accuracy on the independent test sets and network data sets. The improved prediction performance obtained by the FSNN-LGBM makes it a flexible and effective PPI prediction model.
更多查看译文
关键词
Protein-protein interaction,Siamese architecture,functional-link Siamese neural network,light gradient boosting machine
AI 理解论文
溯源树
样例
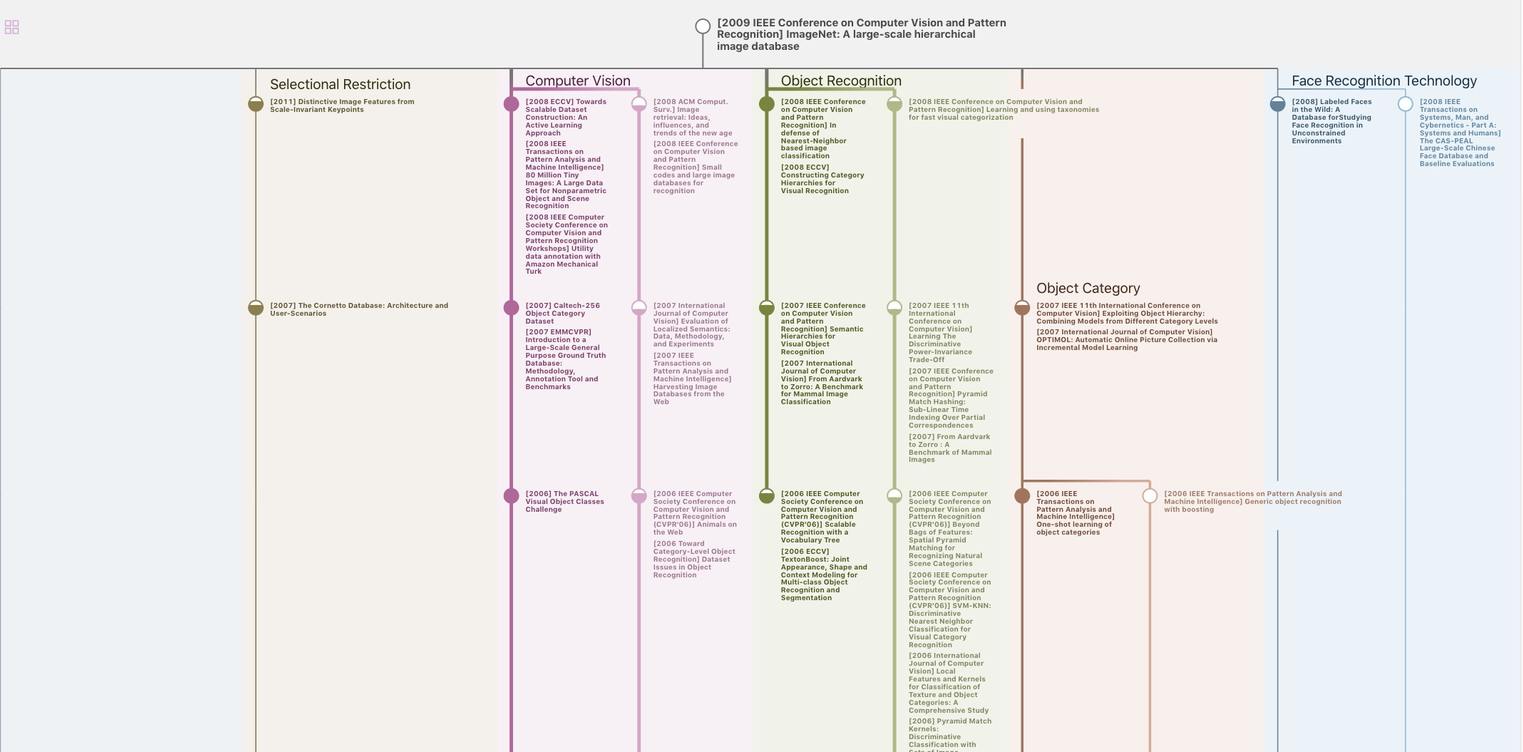
生成溯源树,研究论文发展脉络
Chat Paper
正在生成论文摘要