Mortality, Appropriate and Inappropriate Shocks - Observations from an Institutional Implantable Cardioverter Defibrillator Registry
EP Europace(2021)
AI Read Science
Must-Reading Tree
Example
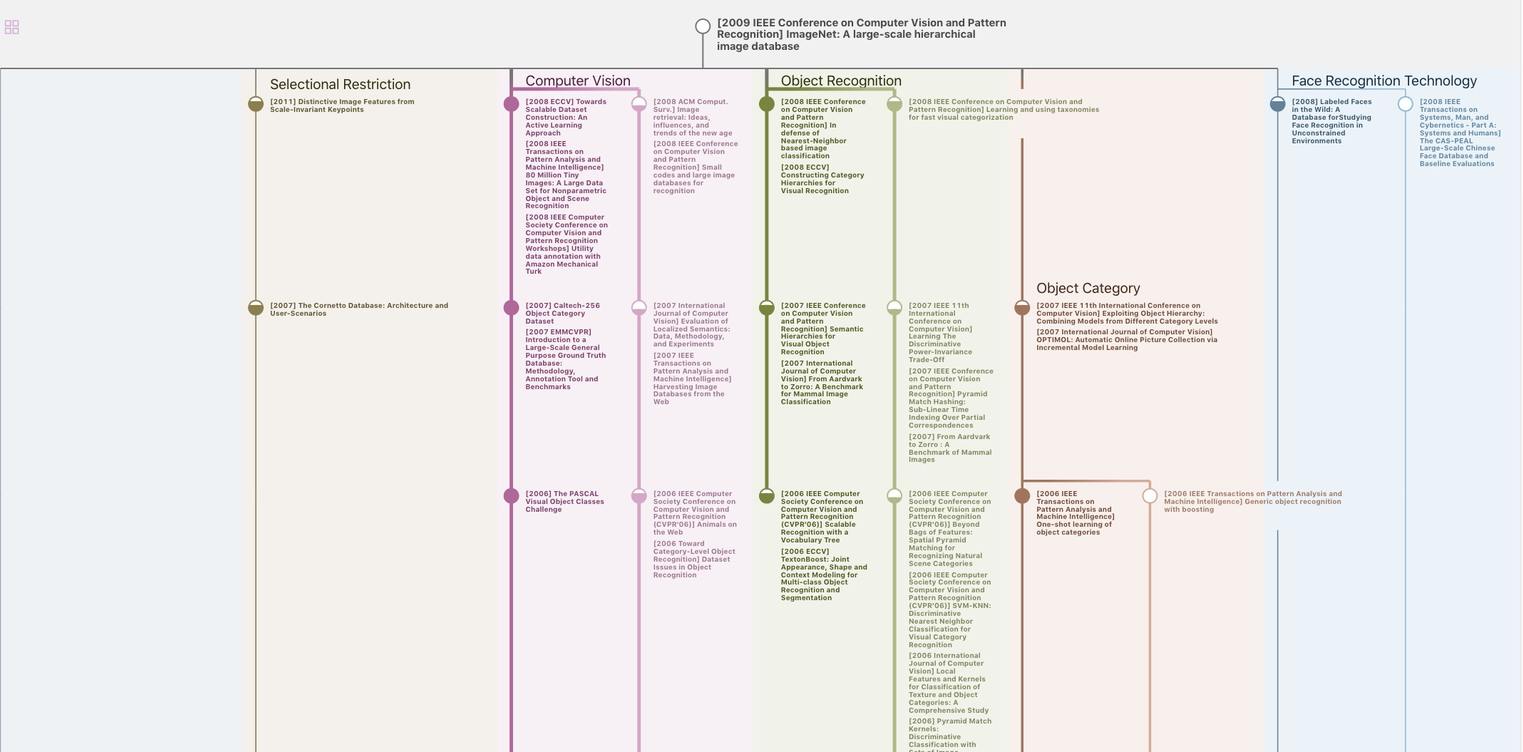
Generate MRT to find the research sequence of this paper
Chat Paper
Summary is being generated by the instructions you defined