OP0018 PREDICTING RESPONSES TO ANTI-TNF TREATMENTS IN RHEUMATOID ARTHRITIS PATIENTS FROM GENETIC AND CLINICAL DATA USING A MACHINE LEARNING APPROACH
Annals of the Rheumatic Diseases(2021)
摘要
Background: Tumor necrosis factor (TNF) inhibitors are key therapies in rheumatoid arthritis (RA). However, a third of patients fail to respond to these agents, and there are no reliable predictors for response. Predictive models, potentially based on clinical and genomic data, are vital to personalizing therapy. The Dialogue for Reverse Engineering Assessments and Methods (DREAM) RA Responder Challenge invited research teams to create models for patient response to anti-TNF therapy. The winning model relied heavily on limited genetic input and was unable to correctly predict responses in a large number of individuals. Objectives: We compared non-linear and linear analytic methods to predict response and non-response to anti-TNF treatment for RA patients in the DREAM database, using moth clinical variables and a large number of potential genome-wide predictors. Methods: DREAM data on anti-TNF treated RA patients were accessed through Synapse (synapse.sagebase.org). Analogously to the DREAM challenge, we were provided with the clinical and genomic data of 2706 patients with at least moderate disease activity according to their composite disease activity scores for 28 joints (DAS28). In contrast to the previous analysis that focused on single nucleotide polymorphisms (SNPs) based on existing knowledge of RA, we used the full genome-wide dataset of 2.5 million SNPs. We first reduced this to 284 SNPs by considering the marginal p-value of 0.001 for each SNP based on whether or not it predicted response. Then, we removed SNPs with borderline significant p-values if they were in linkage disequilibrium with the most significant SNPs. Instead of predicting a binary outcome of responder or non-responder, we trained both linear (e.g. least absolute shrinkage and selection operator, or LASSO) and non-linear models (e.g. Random Forest) to predict a continuous outcome, the change in DAS28 from baseline to 3-12 months after initiation of anti-TNF therapy. We split the patients into training (N=2031) and testing (N=675) subsets and used the predicted response scores to evaluate the true binary response labels for the test patients. Results: The best performing method was Random Forest (RF), a non-linear model that uses decision trees to progressively separate subjects into groups based on the most predictive features. Support Vector Regression (SVR) also out-performed linear methods. Compared to only clinical covariates such as age and sex, adding SNPs improved the prediction from an area under the receiver operating curve (AUROC) of 0.63 to 0.67, i.e., 0.04 improvement. This AUROC of 0.67 was 0.046 greater than the DREAM challenge winner. Conclusion: Non-linear methods such as RF and SVR gave larger predictive improvements compared to linear methods. This may imply some interaction between SNPs and clinical covariatesas potential predictors of response to anti-TNF therapy in RA. We are further investigating these 284 SNPs and their interactions in this regard. References: [1]Guan, Y., Zhang, H., Quang, D., Wang, Z., Parker, S., Pappas, D. A., Kremer, J. M., & Zhu, F. (2019). Machine Learning to Predict Anti-Tumor Necrosis Factor Drug Responses of Rheumatoid Arthritis Patients by Integrating Clinical and Genetic Markers. Arthritis & rheumatology (Hoboken, N.J.), 71(12), 1987–1996. https://doi.org/10.1002/art.41056 [2]Sieberts, S. K., Zhu, F., Garcia-Garcia, J., Stahl, E., Pratap, A., Pandey, G., Pappas, D., Aguilar, D., Anton, B., Bonet, J., Eksi, R., Fornes, O., Guney, E., Li, H., Marin, M. A., Panwar, B., Planas-Iglesias, J., Poglayen, D., Cui, J., Falcao, A. O., … Mangravite, L. M. (2016). Crowdsourced assessment of common genetic contribution to predicting anti-TNF treatment response in rheumatoid arthritis. Nature communications, 7, 12460. https://doi.org/10.1038/ncomms12460 Disclosure of Interests: None declared
更多查看译文
关键词
rheumatoid arthritis patients,rheumatoid arthritis,clinical data,anti-tnf
AI 理解论文
溯源树
样例
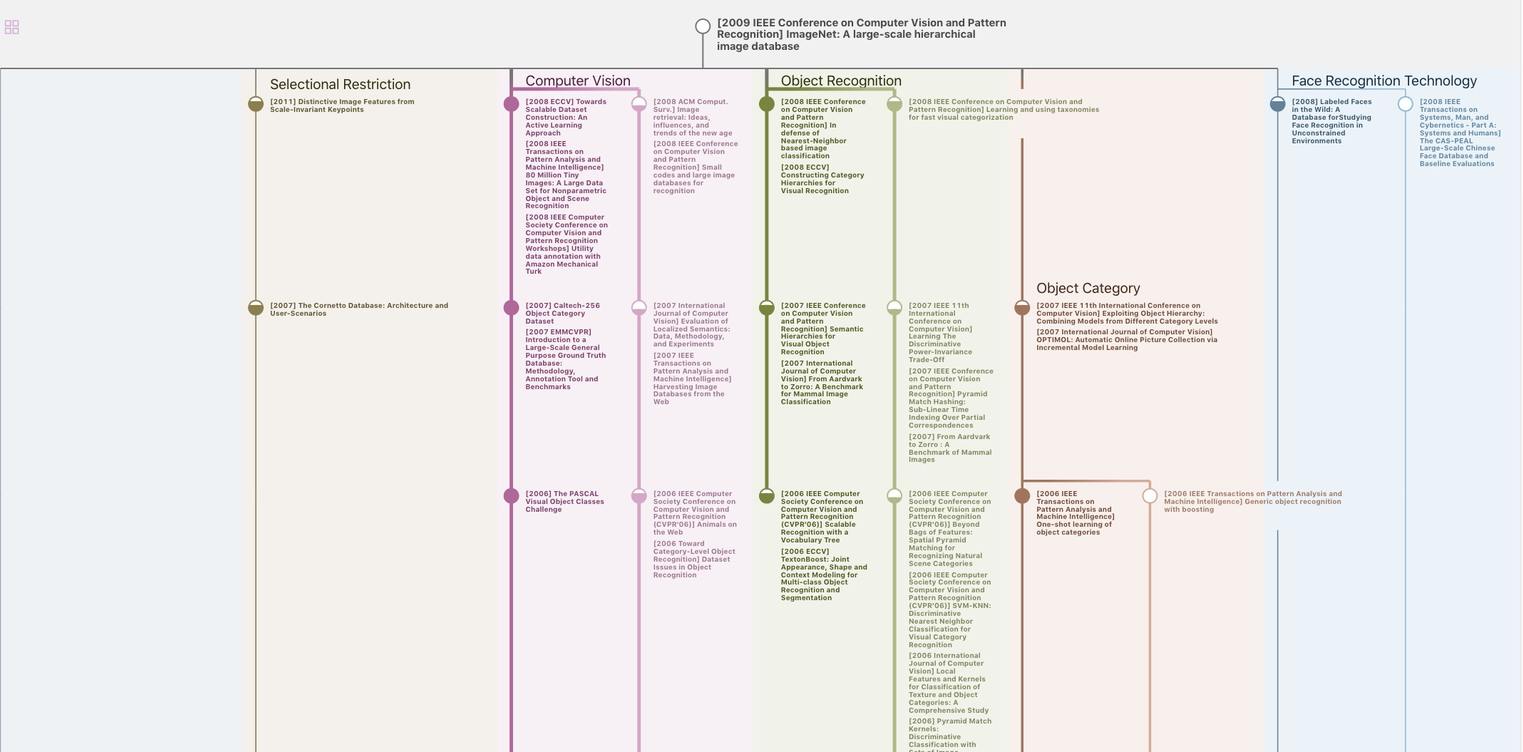
生成溯源树,研究论文发展脉络
Chat Paper
正在生成论文摘要