Improved nutrient management in cereals using Nutrient Expert and machine learning tools: Productivity, profitability and nutrient use efficiency
Agricultural Systems(2021)
摘要
CONTEXT: Smallholder farmers of the Eastern Indo-Gangetic Plains (EIGP) of South Asia rely mainly on cereal- based cropping systems to meet the food and nutritional demand and support their livelihood. Yet the productivity of the major cereals - rice, wheat, and maize - in the region are far lower than their potential. Nutrient management plays a crucial role in improving cereal yields and economic return, and continued improvement in nutrient management practices and their on-farm implementation is required to develop locally relevant solutions that are site-specific, easy-to-develop and geared towards system resilience. OBJECTIVES: The objective of the study was to conduct the comparative assessment of three nutrient management strategies for the three major cereals considering productivity, profitability and nutrient use efficiencies (NUE); estimate their potential yields and yield gaps; and explain the causes of yield variability across farmer-participatory on-farm trials in the EIGP of Nepal. METHODS: We compared three nutrient management strategies (farmer's fertilizer practice- FP, government recommendation -GR, and Nutrient Expert (R)- NE-based recommendation), in 600 on-farm trials. We used the NE DSS tool, APSIM - a cropping system simulation model, and machine learning (ML) approaches (Linear Mixed Effect model-LME; and Random Forest model - RF) for the three cereals using data from those trials. The NE and APSIM were chosen due to simplicity in use and their wider evaluation and application in fertilizer recommendation yield prediction; RF was chosen due to its robustness in predictive ability and identifying and ranking factors determining yield or other variables of interest. RESULTS: The NE-based fertilizer recommendations for maize, wheat and rice increased yield by about 3.5, 1.4, and 1.3 t ha(-1) respectively, increased profits, and improved NUE over FP or GR. The risk analysis showed that at a given probability level, NE always resulted in higher yields of all cereals than GR or FP. APSIM identified 25th June as optimum transplanting date for rice and 10th December as optimum sowing date for maize and wheat and simulated long-term average potential yield of 7-7.5, 5-5.5 and 13-13.3 t ha(-1) respectively for rice, wheat and maize. There were larger yield gaps between PY and FP (2.6-8.5 t ha(-1)) than PY and NE (2.0-3.7 t ha(-1)) across crops and villages. The LME model showed highly significant treatment and location effects for grain yield of all cereals. The point estimate of the difference for grain yield as estimated by Tukey's HSD test was highest for NE-FP and lowest for GR-FP for all crops. The RF model identified grain N uptake for rice and grain P and K uptakes for wheat and maize as most influential factors contributing to their grain yield under each nutrient management strategy. CONCLUSIONS: The NE-based nutrient management had significant effects over FP and GR leading to positive changes on yield and economic performance under varied growing environments. SIGNIFICANCE: These findings based on novel tools and approaches have important policy implications for increasing food security and profits from the major cereals by refining or improving the GR or FP and increasing their NUE in Nepal. Studies with larger sample size across varied agro-climatic zones in the EIGP and much of South Asia would help policy makers consider DSS tools and ML approaches suitable for upscaling and largescale adoption by smallholder farmers.
更多查看译文
关键词
APSIM,Partial factor productivity,Partial nutrient balance,Return on investment,Random Forest,Yield gap
AI 理解论文
溯源树
样例
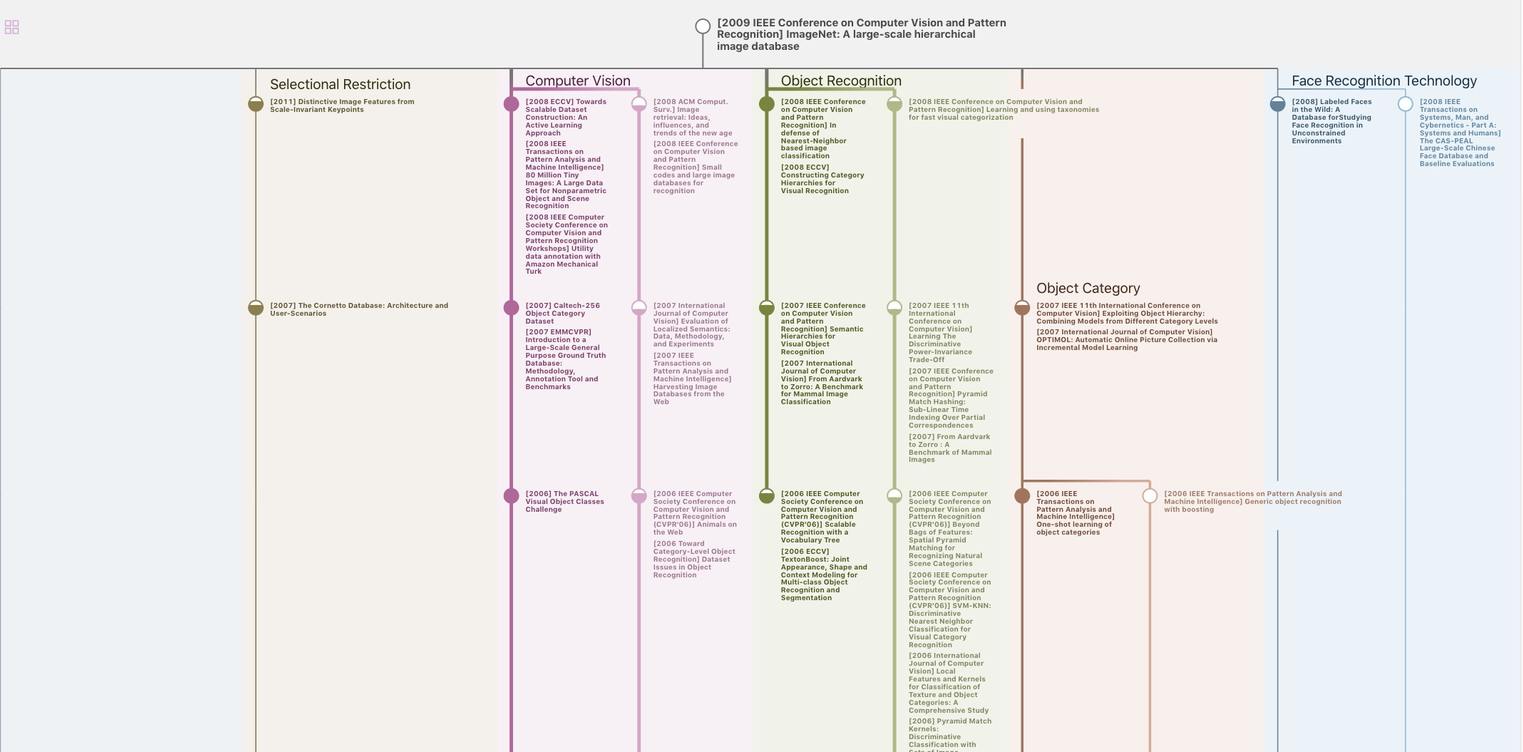
生成溯源树,研究论文发展脉络
Chat Paper
正在生成论文摘要