Learning Video Moment Retrieval Without a Single Annotated Video
IEEE Transactions on Circuits and Systems for Video Technology(2022)
Abstract
Video moment retrieval has progressed significantly over the past few years, aiming to search the moment that is most relevant to a given natural language query. Most existing methods are trained in a fully-supervised or a weakly-supervised manner, which requires a time-consuming and expensive manually labeling process. In this work, we propose an alternative approach to achieving video moment retrieval that requires no textual annotations of videos and instead leverages the existing visual concept detectors and a pre-trained image-sentence embedding space. Specifically, we design a video-conditioned sentence generator to produce a suitable sentence representation by utilizing the mined visual concepts in videos. We then design a GNN-based relation-aware moment localizer to reasonably select a portion of video clips under the guidance of the generated sentence. Finally, the pre-trained image-sentence embedding space is adopted to evaluate the matching scores between the generated sentence and moment representations with the knowledge transferred from the image domain. By maximizing these scores, the sentence generator and moment localizer can enhance and complement each other to achieve the moment retrieval task. Experimental results on the Charades-STA and ActivityNet Captions datasets demonstrate the effectiveness of our proposed method.
MoreTranslated text
Key words
Visualization,Task analysis,Generators,Training,Graph neural networks,Semantics,Detectors,Video moment retrieval,graph neural network,unpaired learning
求助PDF
上传PDF
View via Publisher
AI Read Science
Must-Reading Tree
Example
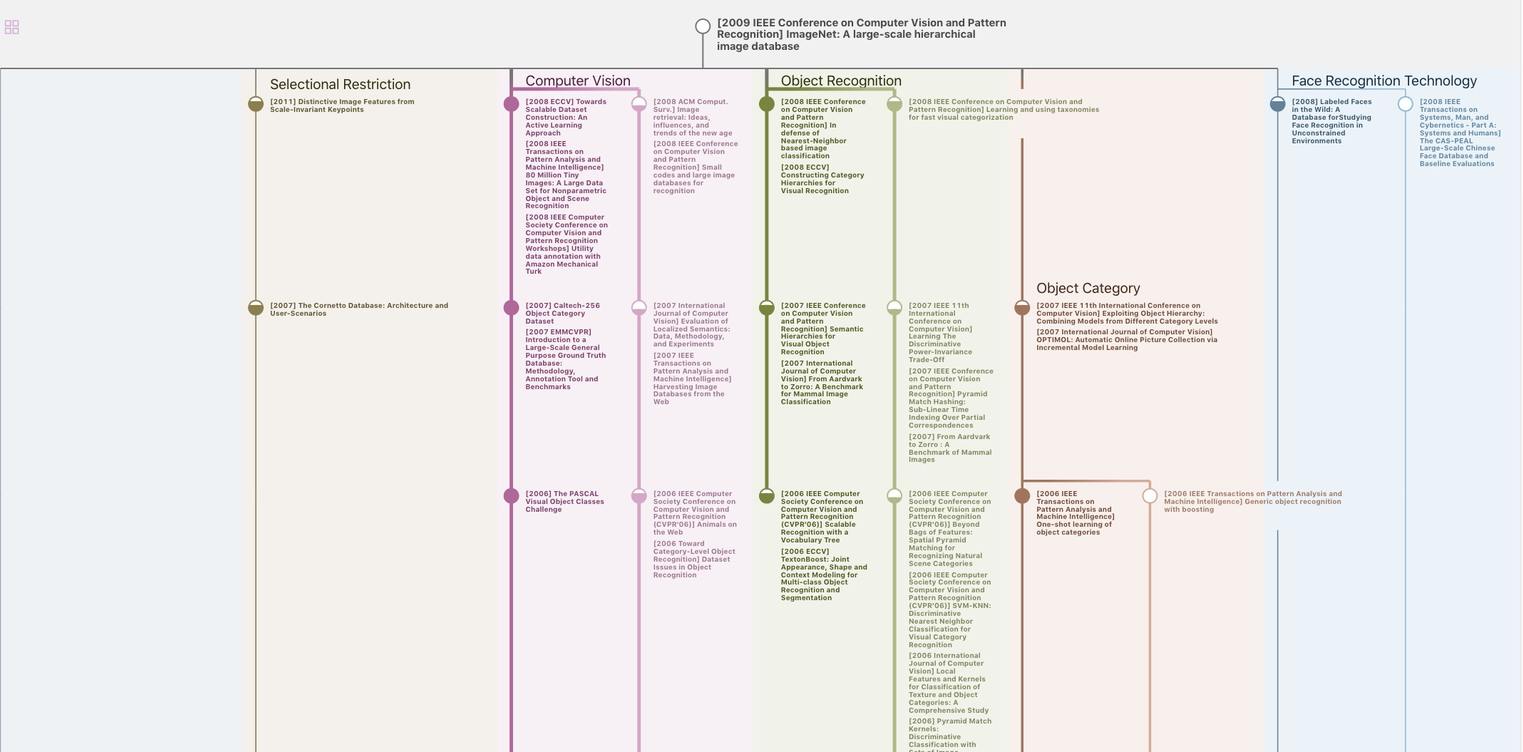
Generate MRT to find the research sequence of this paper
Related Papers
2018
被引用367 | 浏览
International Multimedia Conference 2019
被引用91 | 浏览
2019
被引用210 | 浏览
2019
被引用320 | 浏览
2020
被引用239 | 浏览
2021
被引用29 | 浏览
Data Disclaimer
The page data are from open Internet sources, cooperative publishers and automatic analysis results through AI technology. We do not make any commitments and guarantees for the validity, accuracy, correctness, reliability, completeness and timeliness of the page data. If you have any questions, please contact us by email: report@aminer.cn
Chat Paper
Summary is being generated by the instructions you defined