Accurate Segmentation of Nuclear Regions with Multi-Organ Histopathology Images Using Artificial Intelligence for Cancer Diagnosis in Personalized Medicine.
Journal of personalized medicine(2021)
摘要
Accurate nuclear segmentation in histopathology images plays a key role in digital pathology. It is considered a prerequisite for the determination of cell phenotype, nuclear morphometrics, cell classification, and the grading and prognosis of cancer. However, it is a very challenging task because of the different types of nuclei, large intraclass variations, and diverse cell morphologies. Consequently, the manual inspection of such images under high-resolution microscopes is tedious and time-consuming. Alternatively, artificial intelligence (AI)-based automated techniques, which are fast and robust, and require less human effort, can be used. Recently, several AI-based nuclear segmentation techniques have been proposed. They have shown a significant performance improvement for this task, but there is room for further improvement. Thus, we propose an AI-based nuclear segmentation technique in which we adopt a new nuclear segmentation network empowered by residual skip connections to address this issue. Experiments were performed on two publicly available datasets: (1) The Cancer Genome Atlas (TCGA), and (2) Triple-Negative Breast Cancer (TNBC). The results show that our proposed technique achieves an aggregated Jaccard index (AJI) of 0.6794, Dice coefficient of 0.8084, and F1-measure of 0.8547 on TCGA dataset, and an AJI of 0.7332, Dice coefficient of 0.8441, precision of 0.8352, recall of 0.8306, and F1-measure of 0.8329 on the TNBC dataset. These values are higher than those of the state-of-the-art methods.
更多查看译文
关键词
multi-organ histopathology images,triple-negative breast cancer,The Cancer Genome Atlas,artificial intelligence,nuclear segmentation,stain normalization,cancer grading and prognosis
AI 理解论文
溯源树
样例
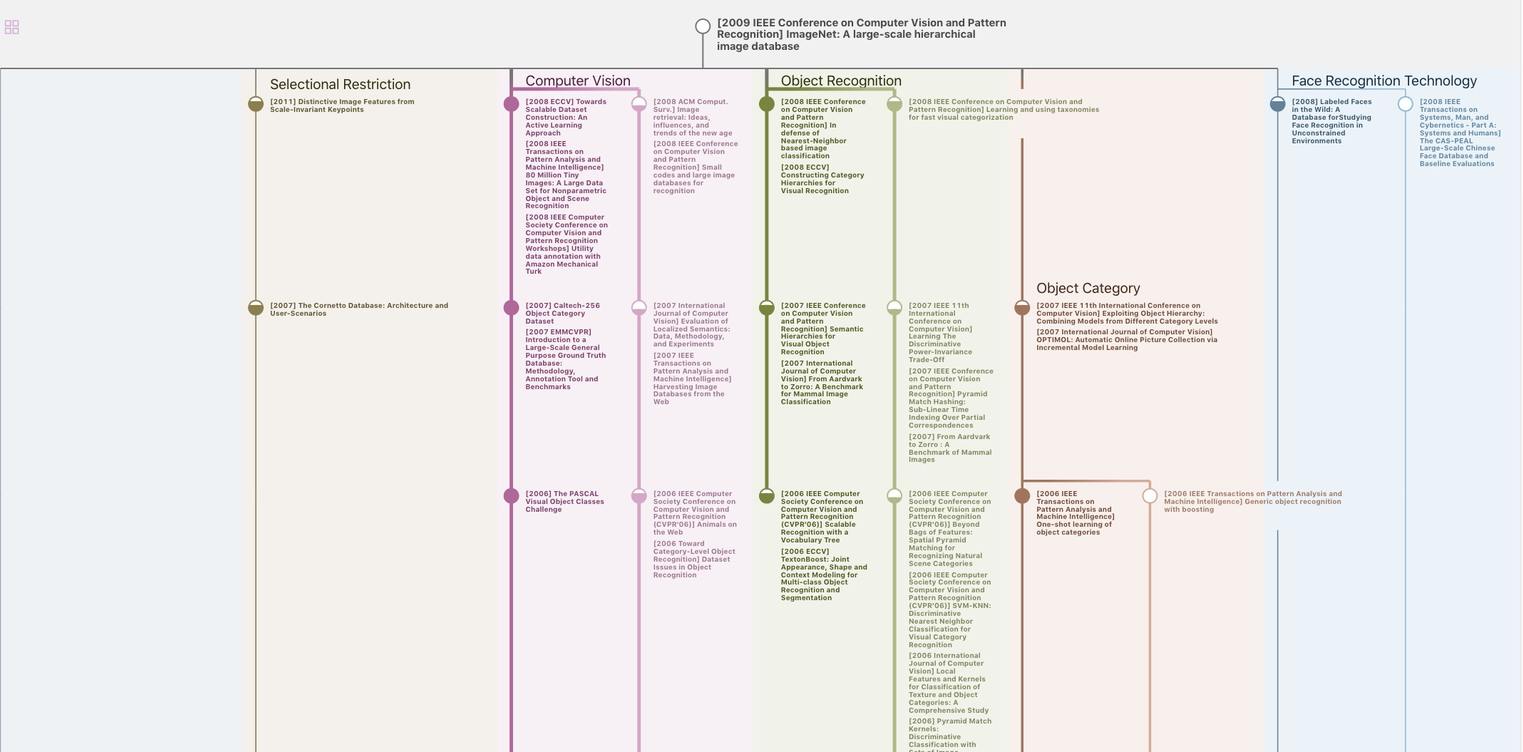
生成溯源树,研究论文发展脉络
Chat Paper
正在生成论文摘要