Semi-supervised Meta-learning with Disentanglement for Domain-Generalised Medical Image Segmentation
MEDICAL IMAGE COMPUTING AND COMPUTER ASSISTED INTERVENTION - MICCAI 2021, PT II(2021)
摘要
Generalising deep models to new data from new centres (termed here domains) remains a challenge. This is largely attributed to shifts in data statistics (domain shifts) between source and unseen domains. Recently, gradient-based meta-learning approaches where the training data are split into meta-train and meta-test sets to simulate and handle the domain shifts during training have shown improved generalisation performance. However, the current fully supervised meta-learning approaches are not scalable for medical image segmentation, where large effort is required to create pixel-wise annotations. Meanwhile, in a low data regime, the simulated domain shifts may not approximate the true domain shifts well across source and unseen domains. To address this problem, we propose a novel semi-supervised meta-learning framework with disentanglement. We explicitly model the representations related to domain shifts. Disentangling the representations and combining them to reconstruct the input image allows unlabeled data to be used to better approximate the true domain shifts for meta-learning. Hence, the model can achieve better generalisation performance, especially when there is a limited amount of labeled data. Experiments show that the proposed method is robust on different segmentation tasks and achieves state-of-the-art generalisation performance on two public benchmarks. Code is publicly available at: https://github.com/vios-s/DGNet.
更多查看译文
关键词
Domain generalisation, Disentanglement, Medical image segmentation
AI 理解论文
溯源树
样例
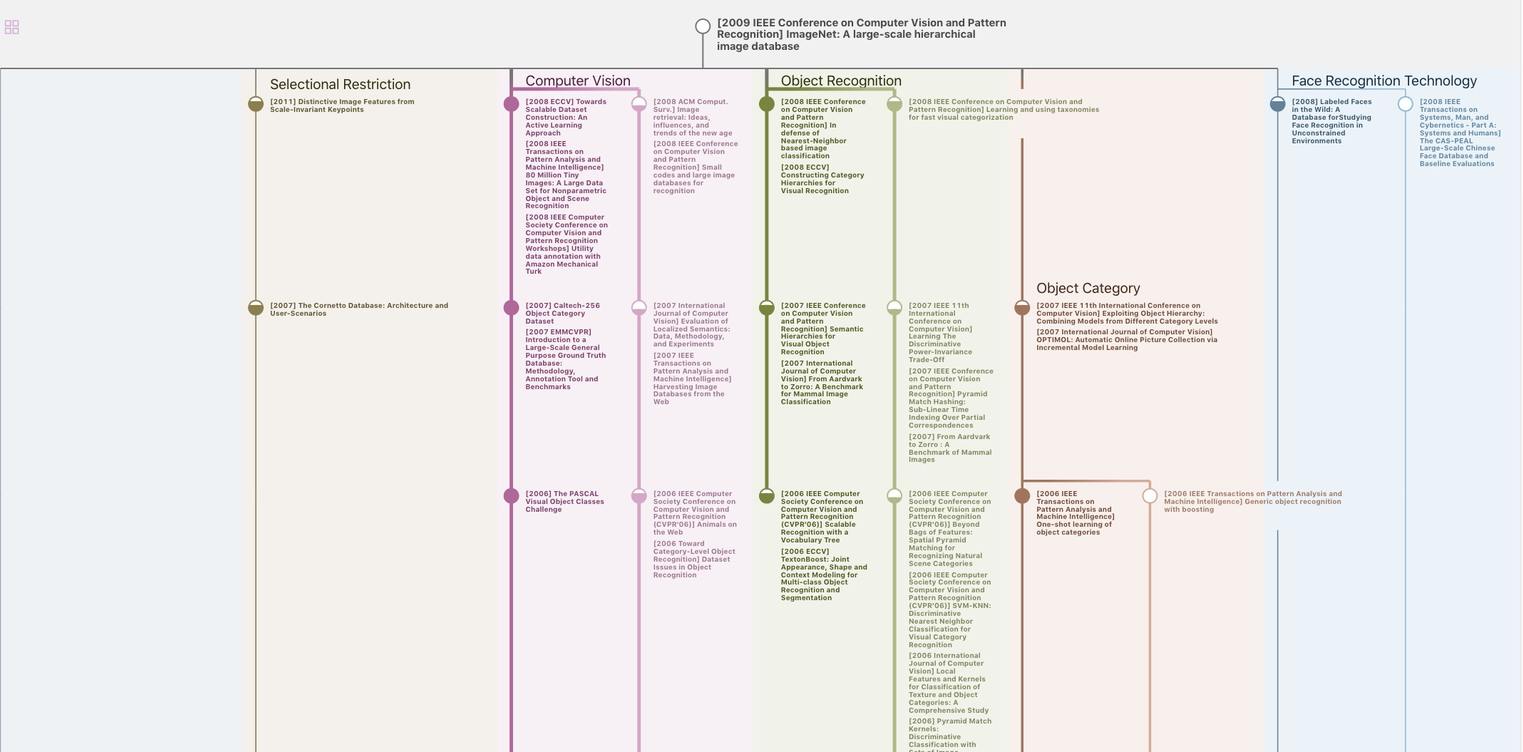
生成溯源树,研究论文发展脉络
Chat Paper
正在生成论文摘要