Adversarial Feature Translation for Multi-domain Recommendation
Knowledge Discovery and Data Mining(2021)
摘要
ABSTRACTReal-world super platforms such as Google and WeChat usually have different recommendation scenarios to provide heterogeneous items for users' diverse demands. Multi-domain recommendation (MDR) is proposed to improve all recommendation domains simultaneously, where the key point is to capture informative domain-specific features from all domains. To address this problem, we propose a novel Adversarial feature translation (AFT) model for MDR, which learns the feature translations between different domains under a generative adversarial network framework. Precisely, in the multi-domain generator, we propose a domain-specific masked encoder to highlight inter-domain feature interactions, and then aggregate these features via a transformer and a domain-specific attention. In the multi-domain discriminator, we explicitly model the relationships between item, domain and users' general/domain-specific representations with a two-step feature translation inspired by the knowledge representation learning. In experiments, we evaluate AFT on a public and an industrial MDR datasets and achieve significant improvements. We also conduct an online evaluation on a real-world MDR system. We further give detailed ablation tests and model analyses to verify the effectiveness of different components. Currently, we have deployed AFT on WeChat Top Stories. The source code is in https://github.com/xiaobocser/AFT.
更多查看译文
关键词
recommender system, multi-domain recommendation, GAN
AI 理解论文
溯源树
样例
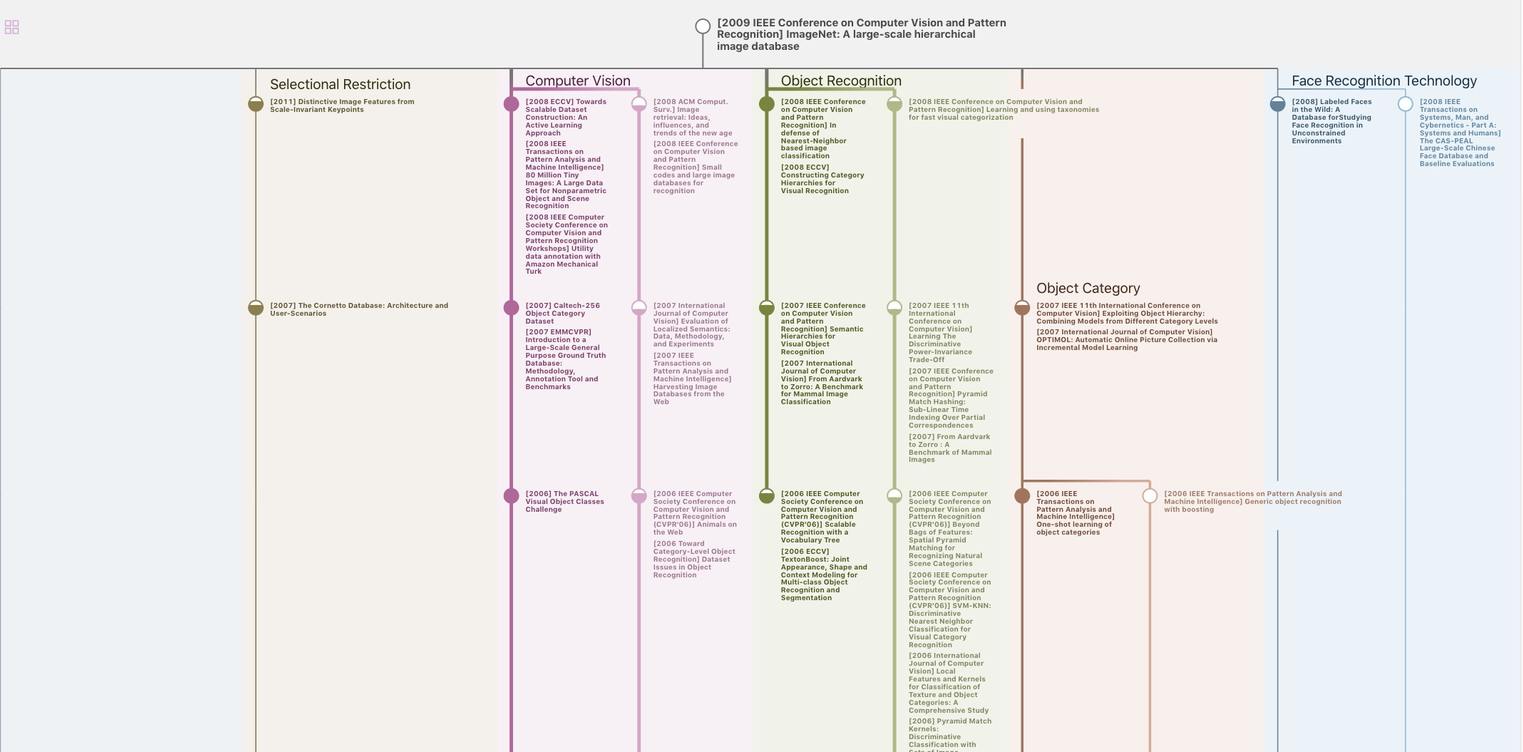
生成溯源树,研究论文发展脉络
Chat Paper
正在生成论文摘要