Privacy-Preserving Representation Learning on Graphs: A Mutual Information Perspective
Knowledge Discovery and Data Mining(2021)
摘要
ABSTRACTLearning with graphs has attracted significant attention recently. Existing representation learning methods on graphs have achieved state-of-the-art performance on various graph-related tasks such as node classification, link prediction, etc. However, we observe that these methods could leak serious private information. For instance, one can accurately infer the links (or node identity) in a graph from a node classifier (or link predictor) trained on the learnt node representations by existing methods. To address the issue, we propose a privacy-preserving representation learning framework on graphs from the mutual information perspective. Specifically, our framework includes a primary learning task and a privacy protection task, and we consider node classification and link prediction as the two tasks of interest. Our goal is to learn node representations such that they can be used to achieve high performance for the primary learning task, while obtaining performance for the privacy protection task close to random guessing. We formally formulate our goal via mutual information objectives. However, it is intractable to compute mutual information in practice. Then, we derive tractable variational bounds for the mutual information terms, where each bound can be parameterized via a neural network. Next, we train these parameterized neural networks to approximate the true mutual information and learn privacy-preserving node representations. We finally evaluate our framework on various graph datasets.
更多查看译文
关键词
Graph representation learning, privacy, mutual information
AI 理解论文
溯源树
样例
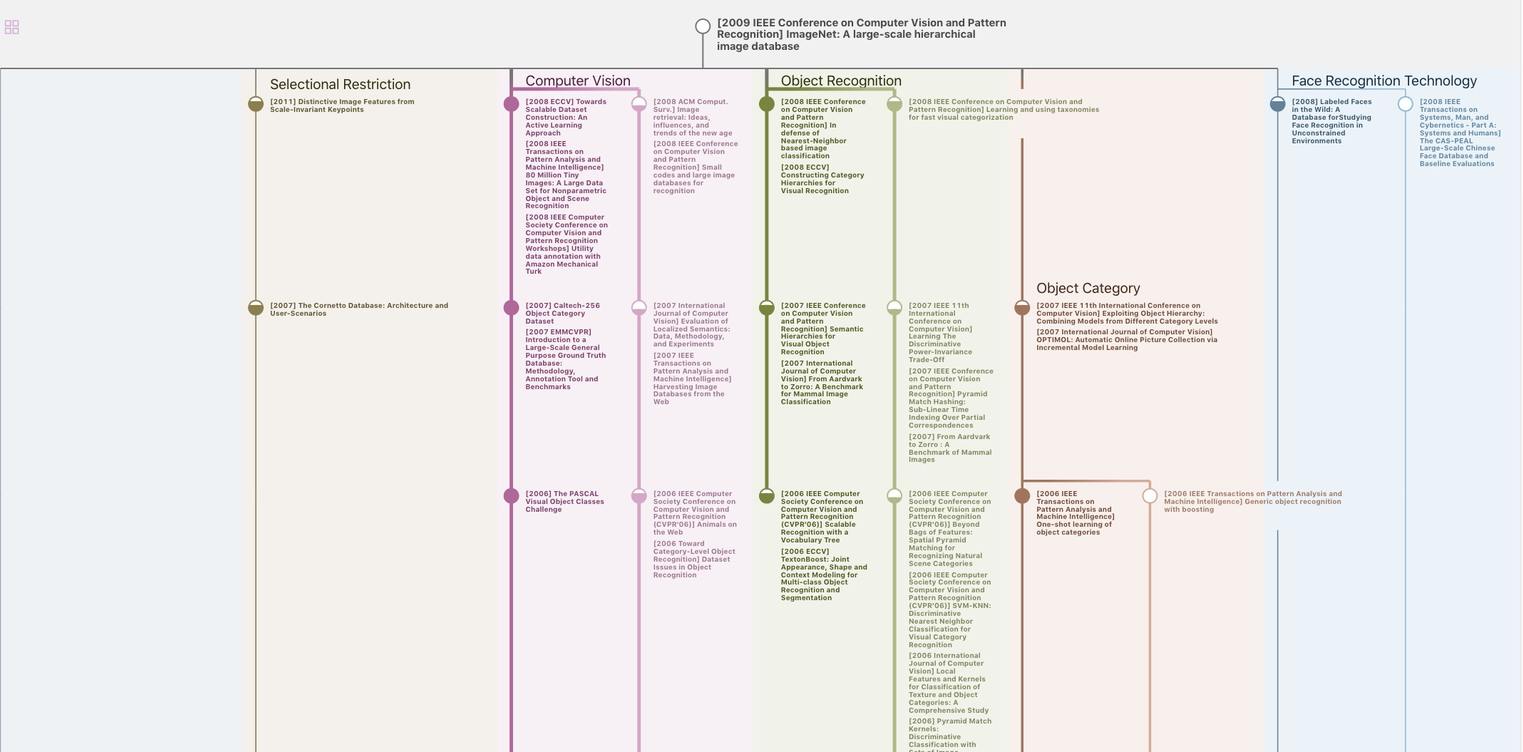
生成溯源树,研究论文发展脉络
Chat Paper
正在生成论文摘要