Popularity Bias in Dynamic Recommendation
Knowledge Discovery and Data Mining(2021)
摘要
ABSTRACTPopularity bias is a long-standing challenge in recommender systems: popular items are overly recommended at the expense of less popular items that users may be interested in being under-recommended. Such a bias exerts detrimental impact on both users and item providers, and many efforts have been dedicated to studying and solving such a bias. However, most existing works situate the popularity bias in a static setting, where the bias is analyzed only for a single round of recommendation with logged data. These works fail to take account of the dynamic nature of real-world recommendation process, leaving several important research questions unanswered: how does the popularity bias evolve in a dynamic scenario? what are the impacts of unique factors in a dynamic recommendation process on the bias? and how to debias in this long-term dynamic process? In this work, we investigate the popularity bias in dynamic recommendation and aim to tackle these research gaps. Concretely, we conduct an empirical study by simulation experiments to analyze popularity bias in the dynamic scenario and propose a dynamic debiasing strategy and a novel False Positive Correction method utilizing false positive signals to debias, which show effective performance in extensive experiments.
更多查看译文
关键词
popularity bias, dynamic recommendation
AI 理解论文
溯源树
样例
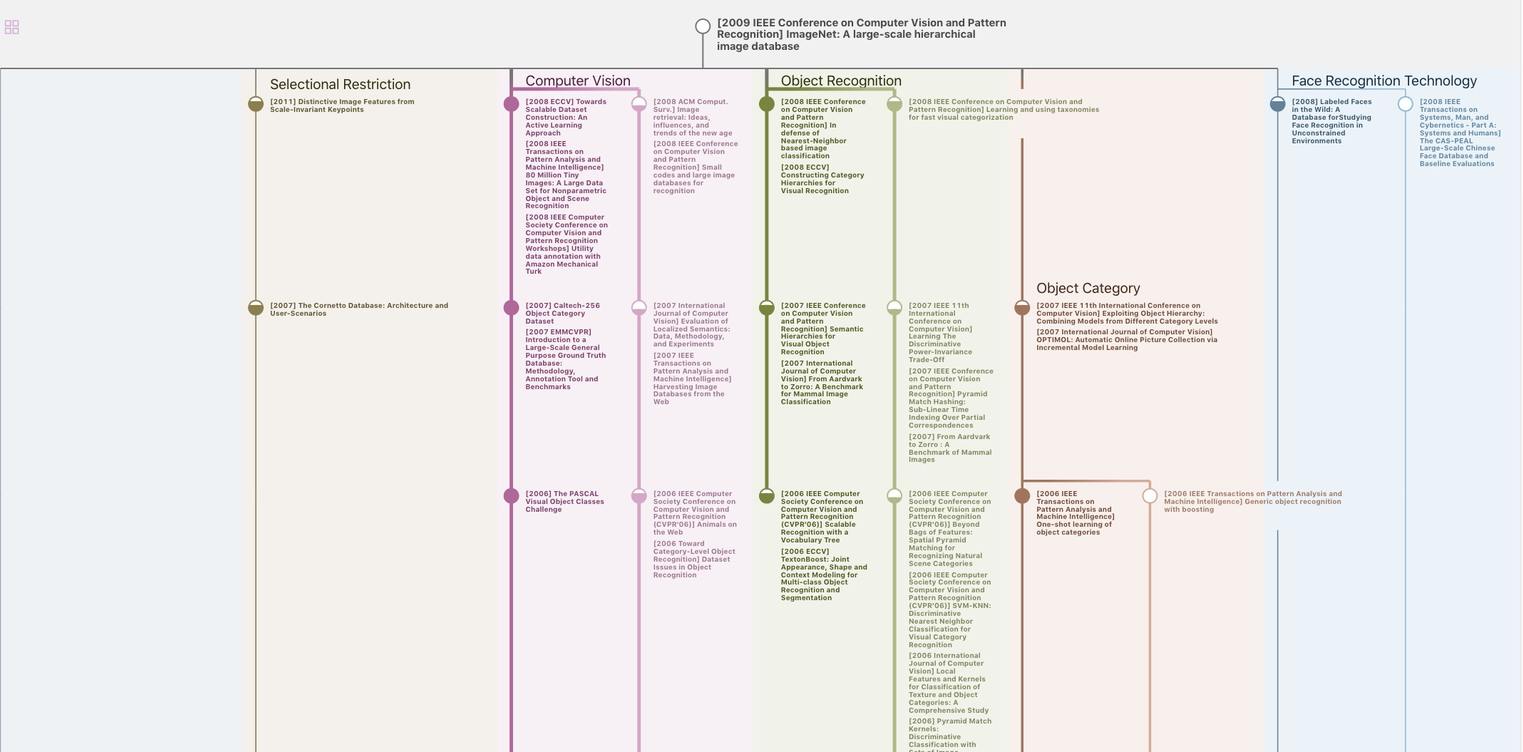
生成溯源树,研究论文发展脉络
Chat Paper
正在生成论文摘要