T3S: Effective Representation Learning for Trajectory Similarity Computation
2021 IEEE 37TH INTERNATIONAL CONFERENCE ON DATA ENGINEERING (ICDE 2021)(2021)
摘要
Advances of the sensor and GPS techniques have motivated the proliferation of trajectory data in a wide spectrum of applications. Trajectory similarity computation is one of the most fundamental problems in trajectory analytics. Considering that the high complexity of similarity computation is usually a bottleneck for large-scale trajectory data analysis, there are many research efforts for reducing the complexity such as the approximate algorithms. However, most of them are proposed for only one or two specific similarity measures, and thus cannot support different similarity measures well. In this paper, we propose a deep learning based model, namely T3S, which embeds each trajectory (i.e., a sequence of points) into a vector (point) in a d-dimensional space, and hence can significantly accelerate the similarity computation between the trajectories. By applying recurrent and attention neural networks, T3S can capture various unique characteristics of the trajectories such as the ordering of the points, spatial and structural information. Furthermore, our learning based T3S can easily handle any trajectory similarity measures by adjusting its parameters through the training. Extensive experiments on two real-life datasets demonstrate the effectiveness and efficiency of T3S. T3S outperforms state-of-the-art deep learning based methods under four popular trajectories similarity measures.
更多查看译文
关键词
effective representation learning,trajectory similarity computation,sensor techniques,large-scale trajectory data analysis,deep learning based model,trajectory similarity measures,GPS techniques,T3S,attention neural networks,recurrent neural networks
AI 理解论文
溯源树
样例
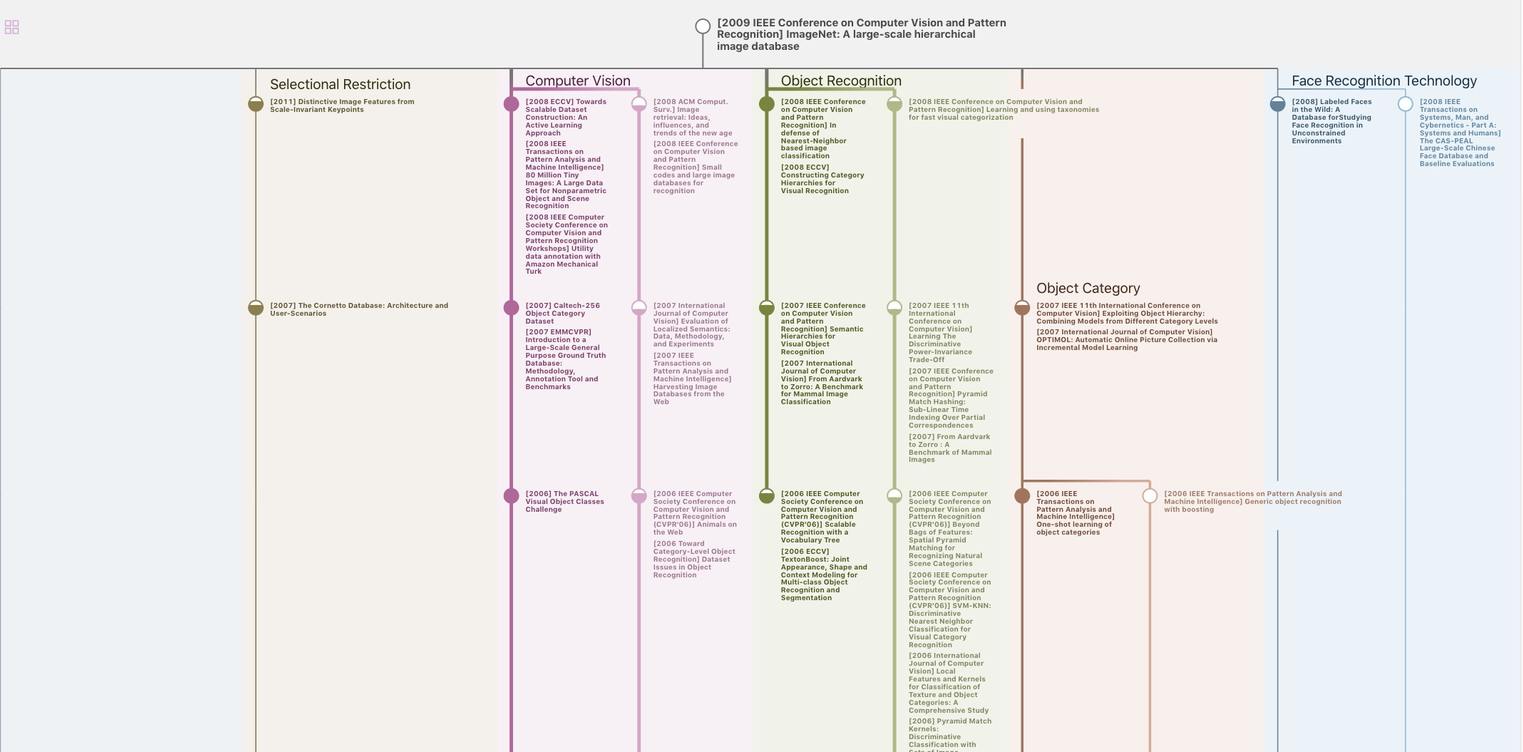
生成溯源树,研究论文发展脉络
Chat Paper
正在生成论文摘要