Towards Consistent Predictive Confidence through Fitted Ensembles
2021 INTERNATIONAL JOINT CONFERENCE ON NEURAL NETWORKS (IJCNN)(2021)
摘要
Deep neural networks are behind many of the recent successes in machine learning applications. However, these models can produce overconfident decisions while encountering out-of-distribution (OOD) examples or making a wrong prediction. This inconsistent predictive confidence limits the integration of independently-trained learning models into a larger system. This paper introduces separable concept learning framework to realistically measure the performance of classifiers in presence of OOD examples. In this setup, several instances of a classifier are trained on different parts of a partition of the set of classes. Later, the performance of the combination of these models is evaluated on a separate test set. Unlike current OOD detection techniques, this framework does not require auxiliary OOD datasets and does not separate classification from detection performance. Furthermore, we present a new strong baseline for more consistent predictive confidence in deep models, called fitted ensembles, where overconfident predictions are rectified by transformed versions of the original classification task. Fitted ensembles can naturally detect OOD examples without requiring auxiliary data by observing contradicting predictions among its components. Experiments on MNIST, SVHN, CIFAR-10/100, and ImageNet show fitted ensemble significantly outperform conventional ensembles on OOD examples and are possible to scale.
更多查看译文
关键词
towards consistent predictive confidence,deep neural networks,recent successes,machine learning applications,overconfident decisions,out-of-distribution examples,wrong prediction,inconsistent predictive confidence,independently-trained learning models,larger system,separable concept,classifier,OOD examples,separate test,current OOD detection techniques,auxiliary OOD datasets,separate classification,detection performance,deep models,called fitted ensembles,overconfident predictions,contradicting predictions,conventional ensembles
AI 理解论文
溯源树
样例
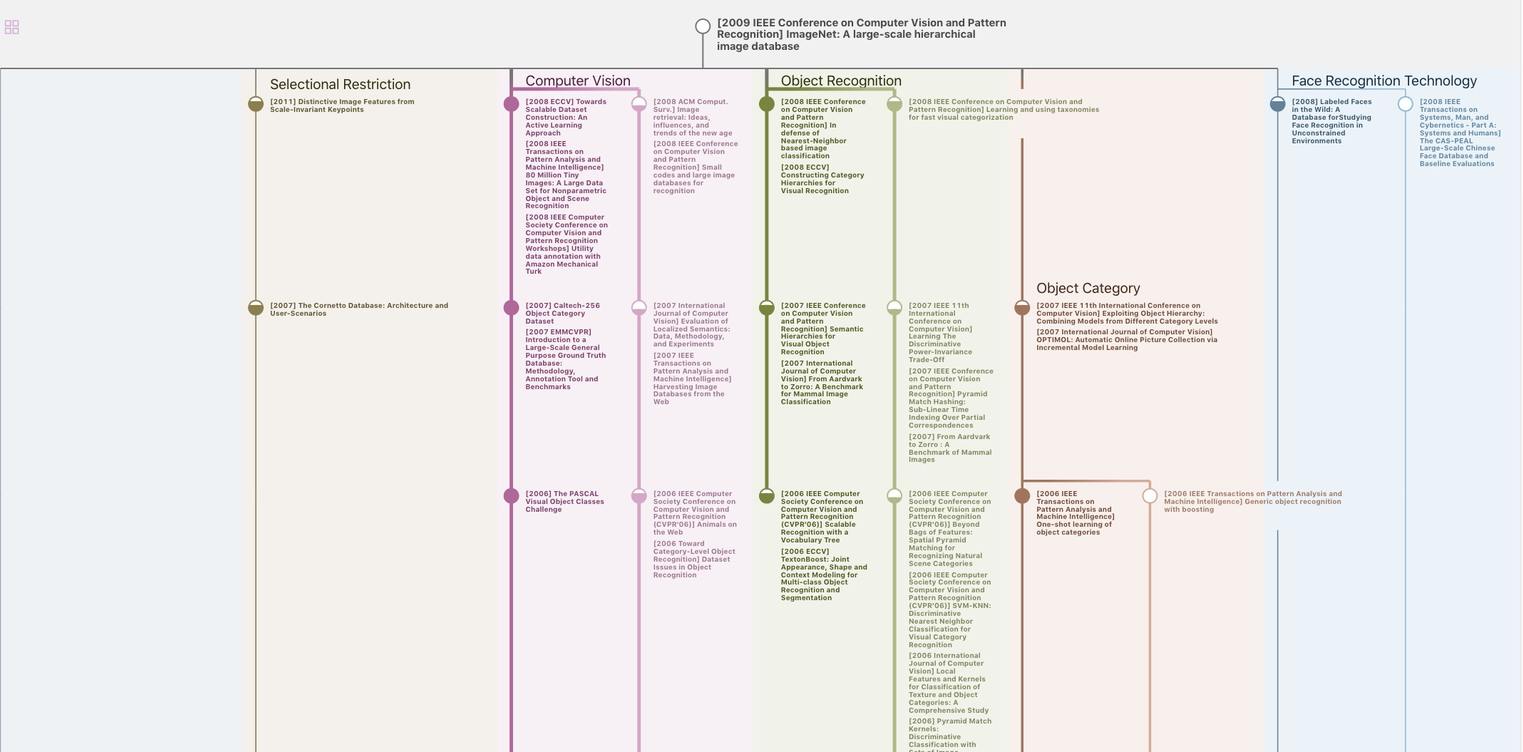
生成溯源树,研究论文发展脉络
Chat Paper
正在生成论文摘要