Wide stochastic networks: Gaussian limit and PAC-Bayesian training
arxiv(2023)
摘要
The limit of infinite width allows for substantial simplifications in the analytical study of over-parameterised neural networks. With a suitable random initialisation, an extremely large network exhibits an approximately Gaussian behaviour. In the present work, we establish a similar result for a simple stochastic architecture whose parameters are random variables, holding both before and during training. The explicit evaluation of the output distribution allows for a PAC-Bayesian training procedure that directly optimises the generalisation bound. For a large but finite-width network, we show empirically on MNIST that this training approach can outperform standard PAC-Bayesian methods.
更多查看译文
关键词
wide stochastic networks,gaussian limit,pac-bayesian
AI 理解论文
溯源树
样例
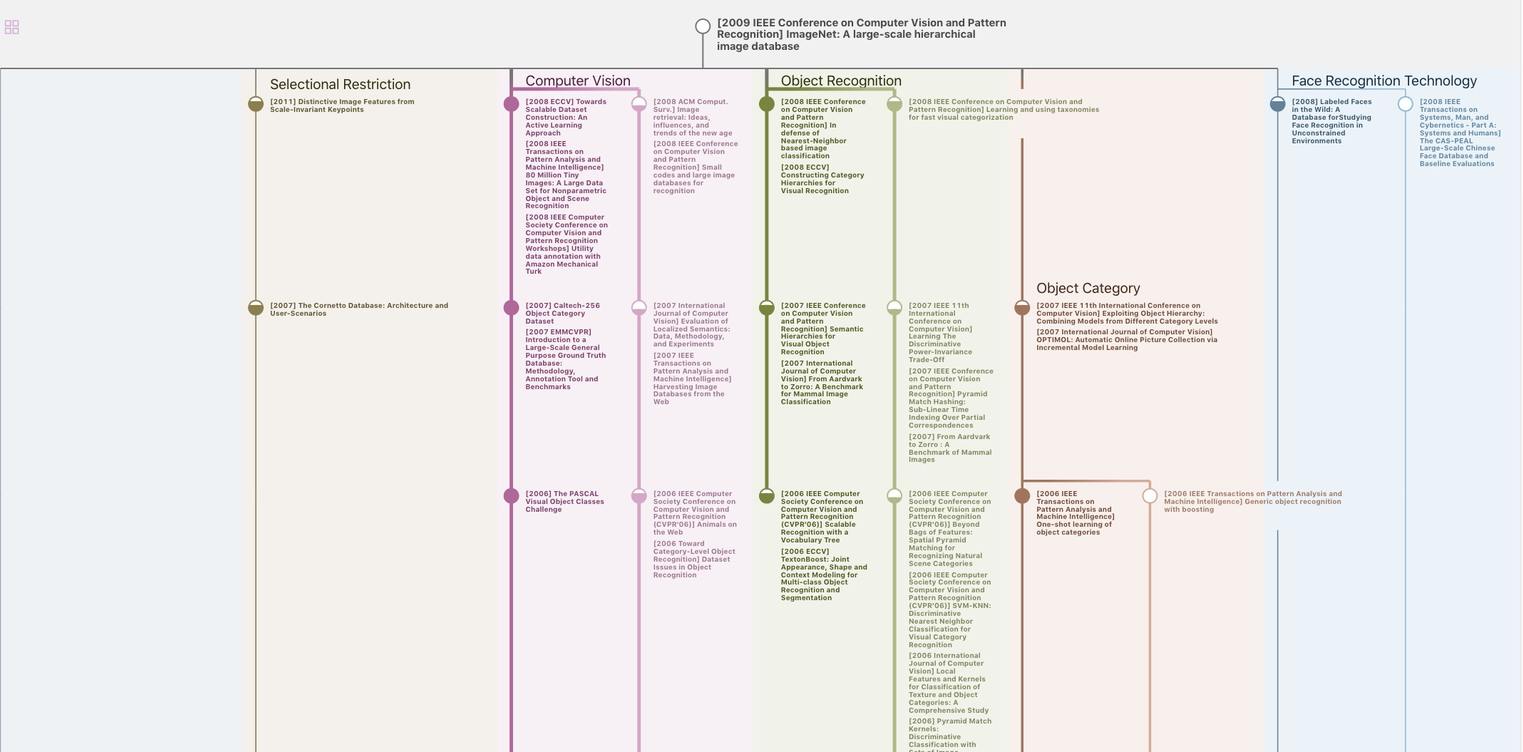
生成溯源树,研究论文发展脉络
Chat Paper
正在生成论文摘要