Pre-training RNNs on ecologically relevant tasks explains sub-optimal behavioral reset
bioRxiv(2021)
摘要
When faced with a new task, animals’ cognitive capabilities are determined both by individual experience and by structural priors evolved to leverage the statistics of natural environments. Rats can quickly learn to capitalize on the trial sequence correlations of two-alternative forced choice (2AFC) tasks after correct trials, but consistently deviate from optimal behavior after error trials, when they waive the accumulated evidence. To understand this outcome-dependent gating, we first show that Recurrent Neural Networks (RNNs) trained in the same 2AFC task outperform animals as they can readily learn to use previous trials’ information both after correct and error trials. We hypothesize that, while RNNs can optimize their behavior in the 2AFC task without a priori restrictions, rats’ strategy is constrained by a structural prior adapted to a natural environment in which rewarded and non-rewarded actions provide largely asymmetric information. When pretraining RNNs in a more ecological task with more than two possible choices, networks develop a strategy by which they gate off the across-trial evidence after errors, mimicking rats’ behavior. Our results suggest that the observed suboptimal behavior reflects the influence of a structural prior that, adaptive in a natural multi-choice environment, constrains performance in a 2AFC laboratory task.
### Competing Interest Statement
The authors have declared no competing interest.
更多查看译文
AI 理解论文
溯源树
样例
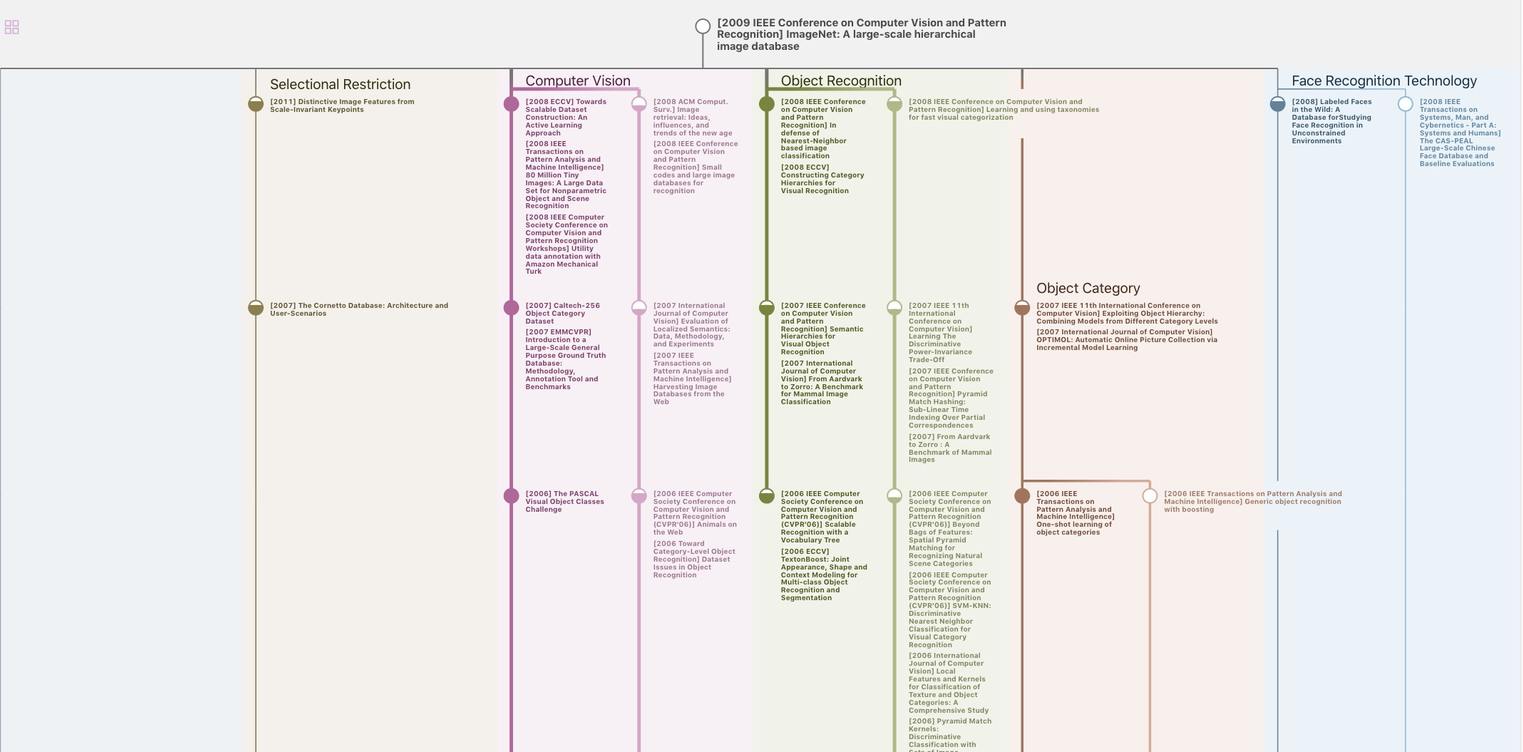
生成溯源树,研究论文发展脉络
Chat Paper
正在生成论文摘要