The Limitations of Federated Learning in Sybil Settings.
RAID(2020)
摘要
Federated learning over distributed multi-party data is an emerging paradigm that iteratively aggregates updates from a group of devices to train a globally shared model. Relying on a set of devices, however, opens up the door for sybil attacks: malicious devices may be controlled by a single adversary who directs these devices to attack the system. We consider the susceptibility of federated learning to sybil attacks and propose a taxonomy of sybil objectives and strategies in this setting. We describe a new DoS attack that we term training inflation and present several ways to carry out this attack. We then evaluate recent distributed ML fault tolerance proposals and show that these are insufficient to mitigate several sybil-based attacks. Finally, we introduce a defense against targeted sybil-based poisoning called FoolsGold, which identifies sybils based on the diversity of client updates. We show that FoolsGold exceeds state of the art approaches when countering several types of poisoning attacks. Our work is open source and is available online: https://github.com/DistributedML/FoolsGold
更多查看译文
关键词
federated learning
AI 理解论文
溯源树
样例
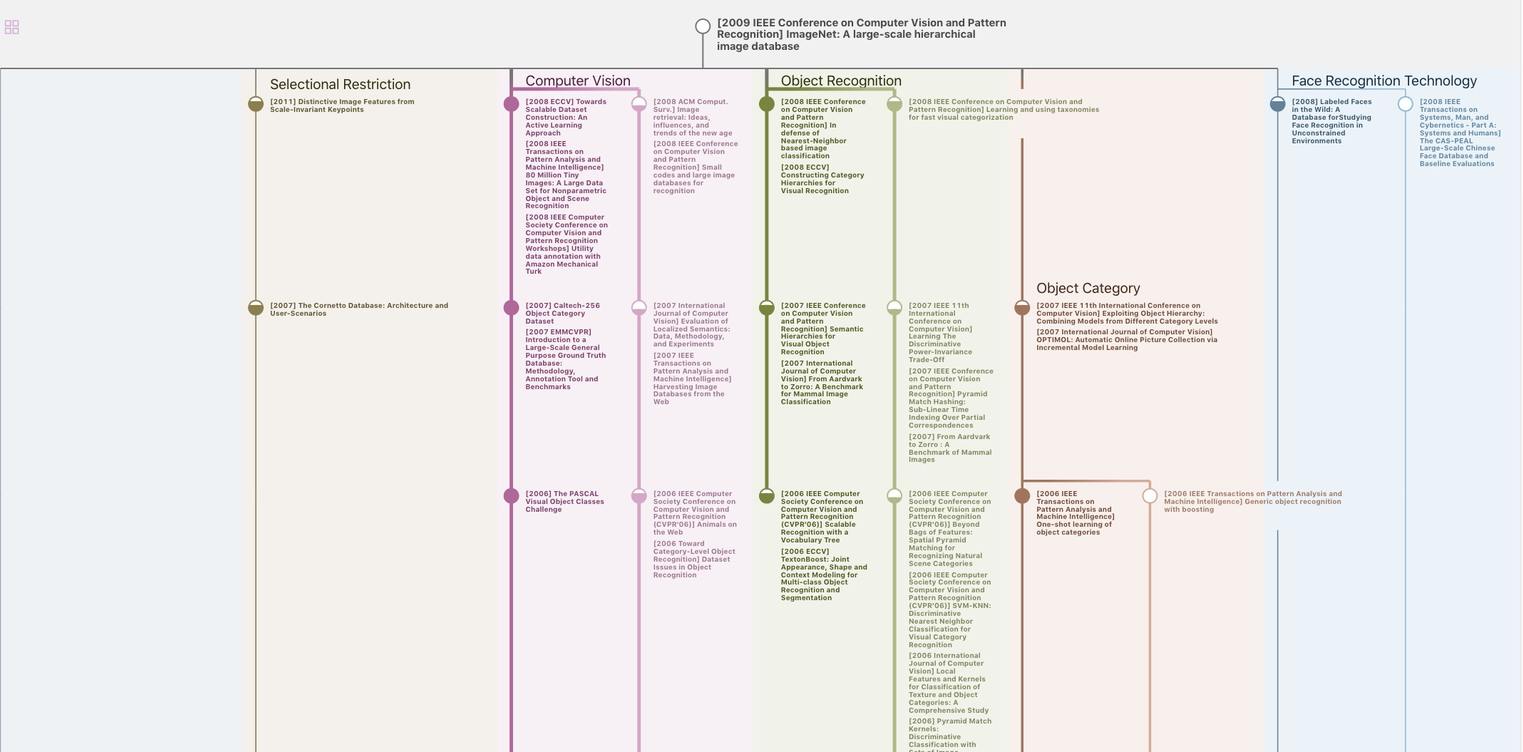
生成溯源树,研究论文发展脉络
Chat Paper
正在生成论文摘要